Data analysts and data scientists are performing two crucial roles today in the modern world of data. They both help organisations use and understand data in different ways. They want to see what’s in the data for patterns and clues, so they named it Data Analyst. What they do with the raw data we as consumers are given and turn into something, something useful that businesses can use to make decisions.
On data and reports, they use Excel and use SQL. However, Data Scientists take the next step. In this post, we will discuss what is the difference between a data analyst and a data scientist. They predict what might come using tools from machine learning and predictive modelling. They are good with Python and R, but they build complex models with which you can predict what is going to happen next.
Also Read: Data Science vs. Data Analytics
Data Analyst vs Data Scientist: What’s the Difference?
Data Analyst
A Data Analyst collects the data, processes them, and checks the end to enlighten or help the companies or organisations to make smart decisions. Most often they are looking to uncover trends, patterns, and insight in data sets and rely on statistical methodology and data visualisation tools. Data Analysts make reports and other presentations through which they dress up complex data to provide useful insights that will help businesses respond to what is happening now.
Data Scientist
A highly data-oriented data scientist takes large complex data and extracts insightful patterns through statistical analysis, machine learning, and predictive modelling. In addition to exploring data, they develop algorithms and models to predict how people will behave in the future. Data Scientists combine data-based insights in the business strategy, and more often they are working on data-driven decision making and performance implied deeper in a business organisation.
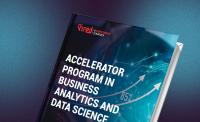
Get curriculum highlights, career paths, industry insights and accelerate your data science journey.
Download brochure
Data Scientist and Data Analyst: Qualifications and Work Experience
Data Analyst
A Bachelor’s Degree in a STEM field (Science, Technology, Engineering, and Mathematics), and a Master’s degree for some is the standard of a Data Analyst. Their education is heavily mathematical, statistical, computer science, engineering, or business-based. Programming, data handling, and statistical analysis all receive training.
Work Experience:
- Entry-Level: The base level of being a data analyst is by starting with an internship and a junior position where they learn the way to collect, process, and analyse data. With these tools, like Excel, sql, and data visualisation software they gain experience.
- Mid-Level: With a handful of years of experience, Data Analysts are moved on to more involved projects, conducting deeper data analysis, creating more intricate reports, and creating dashboards.
- Senior-Level: Projects are led by senior data analysts and mentor junior analysts, as well as offer strategic insights using data. Most of them have experience in several industries and knowledge of many business intelligence tools.
Data Scientist
Data Scientists usually have an advanced degree (Master’s or PhD) in technical subjects associated with advanced topics like machine learning, statistical modelling, and programming languages such as Python, and R.
Work Experience:
- Entry-Level: Internships, research assistant roles, and junior data science roles are the ways entry-level data scientists start their careers. Their initial works involve data cleaning, exploratory data analysis, and the beginning of data building models.
- Mid-Level: As data scientists gain more experience they move to jobs that involve complex projects in predictive modelling, algorithm development, and advanced statistical analysis. Usually, they work in teams and they work with the other departments.
- Senior-Level: Data science teams consist of senior data scientists who lead, and data science teams oversee project management, and are engaged in strategic decision-making processes. It was by no means the first startup that we’ve worked at, and they had a lot of experience working with large datasets, with infrastructure for data, and with the latest and greatest machine learning.
Complementary Roles
Despite their differing focuses, Data Analysts and Data Scientists complement each other in their work. Data Analysts provide actionable insights for immediate business needs, while Data Scientists build predictive models to prepare for future challenges. Understanding both roles is essential for leveraging data-driven opportunities effectively, whether starting a career in data science or hiring professionals.
Roles and Responsibilities of Data Scientist and Data Analyst
Data Analyst:
- Data Querying with SQL: Have a good hand extracting/manipulating data with SQL queries.
- Data Analysis and Forecasting with Excel: It makes use of Excel for analysis and forecasting tasks at a low level of detail.
- Creating Dashboards with Business Intelligence Software: Builds visual dashboards from business intelligence tools.
- It performs diverse analytics (i.e. descriptive, diagnostic, predictive, or prescriptive) in search of patterns and/or trends.
Data Scientist:
- Mining Data with APIs or ETL Pipelines: Data querying experience through APIs or ETL (Extract, Transform, Load) experience.
- Cleaning Data with Programming Languages: Expert in cleaning and processing data using effective software languages including Python and R among others.
- Statistical Analysis: Performs further and detailed statistical calculations from databases to arrive at valuable conclusions.
- Creating Programming and Automation Techniques: Designs and implements solutions to workflows and automation practices that reduce daily tasks to improve their effectiveness.
- Developing Data Infrastructures: Develop the high-quality data structures necessary for processing big and diverse data sets and improving the specifics of the organisation’s data handling.
Additional Notes:
- Tasks and duties can be different within various businesses and companies, and this means the necessity to read over the job descriptions.
- Usually, “data analyst” and “data scientist” are viewed as synonymous, which can also imply overlapping of needed skills.
- Both of the documents, job description, and job advertisement, are important in gaining insight into what a particular company expects from data professionals in particular.
- A combination of both may be found from the competencies elicited from the samples of job descriptions, hence the need to acknowledge the constant changes within the data analytics field.
Data Science vs Data Analyst: Skills and Salary Comparison
Skills Comparison
Data Analyst Skills |
Data Scientist Skills |
Data Mining |
Data Mining |
Data Warehousing |
Data Warehousing |
Mathematics, Statistics |
Mathematics, Statistics, Computer Science |
Tableau and Data Visualization |
Tableau and Data Visualization/Storytelling |
SQL |
Python, R, Java, Scala, SQL, Matlab, Pig |
Business Intelligence |
Big Data/Hadoop |
Advanced Excel Skills |
Machine Learning |
SAS |
Economics |
From this table, the reader can observe the skills related to data analysts and data scientists. Although both occupy common competence areas such as data mining, data warehousing, and data visualisation, contain a wider emphasis on advanced programming and machine learning algorithms, besides report writing. According to the data analysts, they must possess skills in business intelligence and Excel.
Salary Overview of Data Scientist and Data Analyst
Data Analyst Salary:
The nature of a data analyst’s salary depends on a specific experience, industry, and even the area of the globe. Beginners who have just joined the industry earn $ 60000 to $ 70000 per year. In one year a senior data analyst in a competitive industry earns more than $90,000.
Data Scientist Salary:
In terms of remuneration, data scientists satisfy themselves with higher pay compared to data engineers. Entry-level data scientists can be paid anything from $ 80000 – $100000. It means that the senior data scientists or the leaders of such departments can earn $120,000 +, and the mid-level workers can earn from $100,000 to $120,000, while some can even earn more than $150,000 if working in tech-focused industries.
Also Read: Data Scientist Salary in India
Data Scientist Job Outlook
Career Opportunities
Data science skill versatility makes you ready for many roles and industries. Data scientists can work in tech, health care, finance, and all across retail, applying their skill set to improve operations, improve customer experience, and build new products. Granted, skills in data science lend themselves to being widespread across contexts to help make life more convenient.
Advancement Potential
Senior roles for data scientists include lead data scientist, data science manager, and even the chief data officer for the organisations all of which require experience and further education. They provide not only better-paid opportunities but also much better decision-making power concerning the overall management of their companies or other organisations.
Also Read: How to Become a Data Scientist in India
Similarities Between Data Analysts and Data Scientists
Aspect |
Similarities |
Primary Focus |
Both work with large datasets to derive insights |
Data Visualization Tools |
Both use similar tools for data visualisation |
Statistics and Analysis |
Both share a strong foundation in statistics and data analysis |
Collaboration with Stakeholders |
Both require collaboration with stakeholders |
Programming Skills |
Both may use SQL for data querying |
Data Interpretation |
Both derive actionable insights from the data |
Report Generation |
Both communicate findings through reports and visualisations |
Typical Projects |
Both work on projects that drive business decisions |
Educational Background |
Both have strong backgrounds in quantitative fields |
Also Read: Data Analyst Interview Questions and Answers
Conclusion
The employment of both data scientists and data analysts increases in demand, due to the increasing role of quantitative techniques for predicting outcomes of different companies’ decision-making. Since most organisations are beginning to embrace the importance of data and its ability to cause a revolution in any aspect of a business, there has been a concern for experts who can make sense of the objectives from the data. In that landscape, data scientists and data analysts are different, but they have interrelated tasks because their specialised skills are valuable for the strategic management of organisations.
If you imagine yourself at one point launching your career into Business Analytics or Data Science, the Accelerator Program in Business Analytics and Data Science at Hero Vired is a gold-standard course that offers extensive programs to help breathe life into those vital skills of the future. Right now, you have the opportunity to be a part of this evolving area of data analytics and learn what skills you need to create a future in this thriving sector. Join us in embracing the future of data — analytics meets excellence — with Hero Vired!
FAQs
The best degree for you depends on your dream and
career goals. For you to have a degree in data analytics if your interests are data processing and statistical modelling. However, if you’re deeply interested in machine learning or big data, a data science degree is not a bad idea for you.
Data scientists could be paid more competitive salaries because they typically come from somewhat more progressive educational backgrounds and because they tend to be in more lead development roles on the front end. Your pay as a data analyst or data scientist will depend on other factors like geographical location, seniority, and industry.
Let's be honest, coding is crucial to gaining an Online Data Analytics Degree. Although understanding the basics of R and Python is not necessarily very tough, it is somewhat necessary to get fair experience in the essentials of R and Python. Also, one needs to be thoroughly proficient in querying languages like SQL, to succeed in this field.
Certainly, the answer is yes. Aspiring data scientists should learn coding. To be good at data science you need to be fluent in programming languages such as Python and R, but also have a clear understanding of stats and maths.
Thorough knowledge of Python programming is a big advantage for data analysts. Typically, employers foresee data analysts to have some chops with
Python libraries, helping you save time with things like data. As a result, if you wish to succeed in this field, it makes perfect sense to learn Python.
Updated on February 10, 2025