A decision tree in machine learning is a well-liked machine learning approach that can be applied to classification and regression problems. They serve as the best option for beginners in the discipline of machine learning since they are simple to understand, decode, and use.
This guide will cover every facet of the decision tree algorithm in machine learning, covering its basic operating principles, types of decision trees in machine learning, how to design a decision tree learning, and more. You will possess a thorough grasp of decision trees by the conclusion of this article.
Table of Content –
Definition and Concept of Decision Trees in Machine Learning
A decision tree in machine learning is a structure resembling a flowchart where each internal node denotes a test on a feature (such as whether a coin will land tails or heads), each leaf node signifies a class label (decision made upon successfully computing all features), and branches represent connections of features that result in those class labels.
Classification rules are represented by the routes from root to leaf. A decision tree learning model is used to learn simple decision rules inferred from training data to build a training model that can be used to predict the value or class of the target variable.
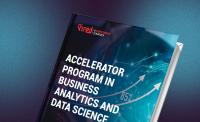
Get curriculum highlights, career paths, industry insights and accelerate your data science journey.
Download brochure
How Does the Decision Tree Algorithm Work?
The decision tree in machine learning operates by recursively dividing the data into subsets according to the most important attribute of the entire node tree.
As long as a stopping requirement is satisfied, such as the minimum quantity of samples in a leaf node or the maximum depth of the tree, the splitting process doesn’t cease. The following steps can be used to summarize the decision tree in machine learning:
- As the root node, start with the complete dataset.
- Find the feature that divides the data into two subsets most effectively.
- Two child nodes should be created, one for each subset.
- On each child node, repeat steps 2 and 3 iteratively until a stopping requirement is satisfied.
Decision Tree Algorithms and their Applications
A supervised learning approach known as a decision tree in machine learning can be applied to regression and classification problems. They are a popular option for novices in machine learning since they are simple to comprehend and interpret. Examples of typical applications include customer churn, fraud detection, medical diagnosis, and email spam filtering.
Advantages and Limitations of a Decision Trees
Advantages:
- It is easy to comprehend since it uses the same reasoning process that a human would use to arrive at any decision in the actual world.
- For difficulties involving decisions, it can be quite helpful.
- It is beneficial to consider every scenario that could result from an issue.
- Compared to other algorithms, less data cleansing is needed.
Limitations:
- The decision tree is complicated since it has several tiers.
- The Random Forest algorithm can fix any overfitting problems it may have.
- The decision tree’s technical complexity might rise with more class labels.
Decision Tree Structure and Terminology
Root Node |
The complete population or sample is represented by the root node, which is then partitioned into two or more homogenous sets. |
Splitting |
This process involves splitting or dividing a node into two or more sub-nodes. |
Decision Node |
A sub-node is called a decision node when it divides into more sub-nodes. |
Terminal Node |
Leaf or Terminal Nodes are nodes that do not split. |
Pruning |
It is the process of removing sub-nodes from a decision node. You might describe splitting in reverse. |
Sub-Tree/Branch |
A branch or sub-tree is a division of the overall tree. |
Sub-Nodes |
Sub-nodes are the offspring of a parent node |
Understanding the Hierarchical Structure of a Decision Tree
The decision-making process is reflected in a decision tree’s hierarchical structure. The tree’s root node represents the initial decision, while the branches show the potential consequences. The last judgments that can be made are represented by the tree’s leaf nodes.
Interested in learning more about machine learning concepts? Check this article about logistic regression machine learning to uncover new insights.
Introduction to Decision Criteria, Splitting, And Pruning
Machine learning algorithms called decision trees can be applied to classification and regression problems. They operate by splitting the data into ever smaller subsets, each of which is then pure.
This is accomplished by segmenting the data according to decision criteria, like the importance of an attribute or the likelihood of a result. Recursively repeating the splitting procedure until the necessary degree of purity is attained.
An approach for enhancing decision tree performance is pruning. It entails cutting back on the tree’s branches, which can lessen overfitting. When a tree gets overly complicated and begins to memorize the training set rather than understanding the underlying patterns, overfitting takes place.
Learn more about: Regression Testing – Meaning, Types and Tools
Step-By-Step Process of Building a Decision Tree from Scratch
- Step 1: Obtain the dataset’s list of rows that are considered (recursively) at each node when constructing a decision tree.
- Step 2: Determine our dataset’s uncertainty, the Gini impurity, the degree of data blending, etc.
- Step 3: List all the inquiries that must be made at that node.
- Step 4: Depending on the question, divide the rows into True and False rows.
- Step 5: Given the data’s partitioning and gini impurity, determine the information gain.
- Step 6: Regarding each inquiry posed, update the most information gained.
- Step 7: Revise the optimal question based on knowledge gained (greater information gain).
- Step 8: Subtract the node from the best query.
- Step 9: Continue from Step 1 until we have only pure nodes (leaf nodes).
Calculation of Impurity Measures and Attribute Selection
Measures of impurities are used to estimate how homogeneous a data set is. Entropy and Gini impurity are two popular impurity measurements. The attribute selection method selects the attribute that divides the data set according to the impurity measure most effectively.
The first preference is given to the attribute with the biggest impurity reduction. This process is repeated until the data set is divided into only leaf nodes.
Ensemble Methods and Decision Trees in Machine Learning
To build a more robust model, ensemble approaches mix several different decision trees in machine learning. This is accomplished by first merging the predictions of the individual decision trees after each tree has been trained on a distinct sample of the data. Ensemble techniques can increase the model’s accuracy and lessen overfitting.
Learn more about: What is Bagging vs. Boosting in Machine Learning?
Use Cases of Decision Trees in Various Domains
Here are the following use cases of a decision tree in machine learning in different domains:
Healthcare |
Planning for diagnosis, risk assessment, treatment |
Logistics |
Preventive maintenance, route planning, inventory control |
Finance |
Portfolio management, fraud detection, and credit rating |
Marketing |
Determining the target market, improving the campaign, and preventing churn |
Retail |
Fraud detection, consumer segmentation, and product recommendations |
Best Practices and Tips
Here are the best practices and tips for making and implementing a decision tree in machine learning:
- Use the appropriate impurity measurement. Entropy and Gini impurity are two popular impurity measurements.
- Pick the appropriate attribute selection technique. There are numerous options for attribute selection techniques.
- Pruning will help prevent overfitting. Overfitting happens when the tree gets too complicated and starts to remember the training set.
- Cross-validation should be used to assess the model.
- Cross-validation aids in avoiding overfitting and provides a more accurate assessment of the model’s effectiveness.
- Make sense of the tree. To comprehend the data and make predictions, decision trees are frequently simple to interpret.
Conclusion
With this comprehensive guide on decision tree classifiers in machine learning, you should now face no problems in resolving complex decisions. With Hero Vired’s Machine Learning and Artificial Intelligence course, you can master every aspect of data science without hassle.
Begin your educational journey right away!
FAQs
Compared to using a single decision tree in machine learning, ensemble approaches integrate multiple decision trees to generate higher predicting performance. The ensemble model's basic tenet is that weak learners can be combined to create stronger learners.
One of the most popular and useful supervised learning methods is the ML decision tree. It can be used to complete classification and regression tasks, with the latter being more frequently utilized in real-world settings. There are three different sorts of nodes in this tree-structured classifier.
Interpretability, accuracy, complexity, deployment, and maintenance are specific considerations for using decision tree in machine learning in real-world applications. To ensure the model is precise, understandable, and deployable when utilizing decision trees in practical applications, it is crucial to consider these variables.
When it comes to decision tree algorithms in machine learning, the popular ones are CART, QUEST, CHAID, and Random Forest.
Updated on April 24, 2024