Every machine learning beginner faces difficulty in devising project ideas. This is where our article on the 12 Ultimate Machine Learning Projects Ideas for Beginners comes to their aid. The blog highlights all the best project ideas an ML beginner can work on.
However, programmers now wonder how to enhance prediction accuracy while working on such projects. To date, implementing ensemble ML methods is one of the best ways to improve model accuracy.
In this article, you will get an in-depth overview of the two popular ensemble learning methods: bagging and boosting. Also, you’ll learn how to use bagging and boosting in machine learning solutions. So, without any further ado, let’s start.
What is an Ensemble Method?
An ensemble learning method is a notion that integrating different models can result in more effective and accurate models.
The ensemble learning method combines several models, often known as ‘weak learners,’ to generate better outcomes, stability, and better prediction performance that are superior to those of the individual models used in isolation.
In predictive modeling, you can create an endless number of ensembles. Despite that, bagging and boosting are the two strategies most frequently leveraged in ensemble learning.
Understanding the fundamental notions of bagging and boosting with useful examples is crucial to understanding these techniques. Let’s start with that, then.
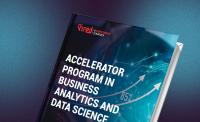
Get curriculum highlights, career paths, industry insights and accelerate your data science journey.
Download brochure
What is Bagging?
The bagging technique in machine learning is also known as Bootstrap Aggregation. It is a technique for lowering the prediction model’s variance. Regarding bagging and boosting, the former is a parallel strategy that trains several learners simultaneously by fitting them independently of one another. Bagging leverages the dataset to produce more accurate data for training. This is accomplished when the original dataset extracts replacement sampling for subsequent usage.
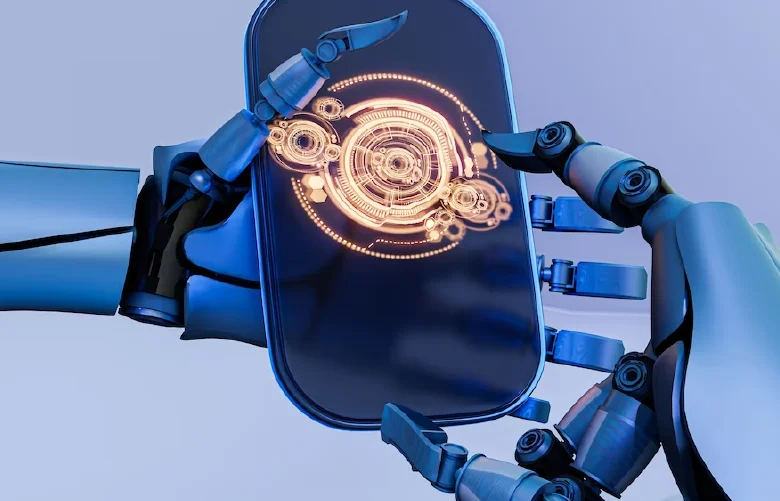
When sampling with replacement, every new training data set may experience repetition in certain observations. Every component of Bagging has an equal chance of turning up in a fresh dataset.
How Does Bagging Work?
Regarding bagging and boosting in machine learning, bagging works in the following way:
- The actual dataset (original one) is divided and categorized into numerous subsets, picking observations with replacements.
- There will be the creation and development of a base model in every subset.
- As the subsets are independent of one another, there will be parallel training conducted for each model.
- The final prediction is derived and made once you integrate the predictions from each and every model.
Several machine learning experts use bagging as a technique to create ML models for the healthcare sector. Don’t know how? Give a read to the 14 Machine Learning in Healthcare Examples to Know!
Implementation of Bagging
When it comes to bagging and boosting in machine learning, the implementation of the former technique is done in the following ways:
- Step 1: Let’s say that a training set comprises ‘p’ features and ‘n’ observations. Without replacement, go to the training dataset and pick a random sample.
- Step 2: Once you randomly choose a subset of ‘p’ features, you leverage the sample observations to create a model.
- Step 3: To split the nodes, you’ll have to use the feature providing the most effective split out of the lot.
- Step 4: You’ll observe that the tree is grown. It implies you’ve access to the best root nodes.
- Step 5: You’ll have to repeat the above-mentioned steps n times. Once done, you’ll get the best prediction result as it clusters the output of the separate decision trees.
Advantages of Bagging
- Enhanced Accuracy: Bagging boosts the accuracy and precision of the ML (machine learning) algorithms to ensure statistical classification and regression.
- Lowers Variance: It lowers the overfitting and variance to devise a more accurate and precise learning model.
- Weak Learners Conversion: Parallel processing is the most efficient solution to convert weak learner models into strong learners.
Examples of Bagging
When comparing bagging vs. boosting, the former leverages the Random Forest model. This model includes high-variance decision tree models. It lets you grow trees by enabling random feature selection. A Random Forest comprises numerous random trees.
What is Boosting?
Now let’s look at the latter when it concerns bagging vs. boosting. The sequential ensemble technique known as “boosting” iteratively modifies the weight of each observation based on the most recent categorization.
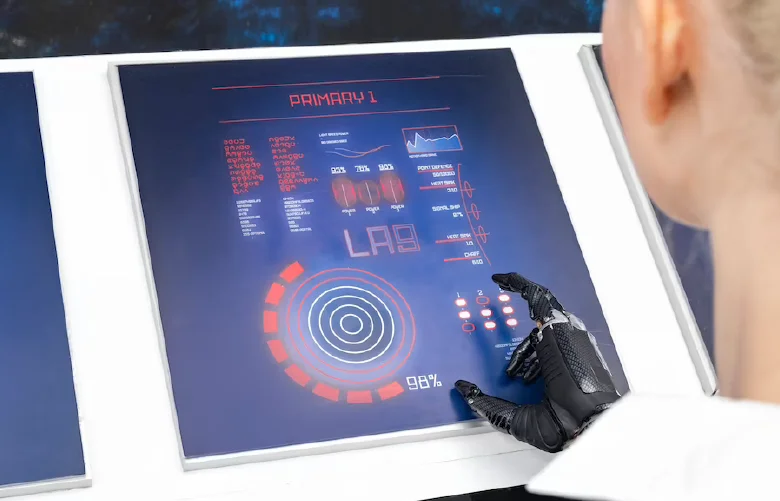
The weight of the observation is increased if it is mistakenly classified. In plain English, “boosting” alludes to algorithms that strengthen a poor learner. It creates robust predictive models and reduces bias error.
How Does Boosting Work?
When it comes to bagging and boosting in machine learning, the latter works in the following ways:
- The training dataset creates a subset comprising data points with equivalent weightage.
- For the initial dataset, you’ll create a base model. This model can later serve the purpose of making predictions on the whole dataset.
- The actual and predicted values will be used to calculate and determine errors. Higher weightage is given to the incorrectly predicted observations.
- While the subsequent model is created or devised, the boosting attempts to rectify the previous model’s errors. The procedure is repeated for numerous models, and every time, boosting rectifies the errors of the last model.
- In the end, the final model comes out as a strong learner. It is then considered the weighted means of every other model.
Implementation of Boosting
When it comes to bagging and boosting, the latter is implemented in the following ways:
- Step 1: Start with the dataset and give equivalent weightage to all data points.
- Step 2: To locate and find the incorrectly classified data points, provide this as input to the model.
- Step 3: To do so, lower the weights of the correctly classified data points while increasing the weights of the incorrectly classified ones.
- Step 4: Once done, normalize every data point’s weightage.
Advantages of Boosting
- Reduce Variance: Boosting techniques in machine learning enables a quick solution to the two-classification problem while lowering the variance effectively.
- Deals With Missing Data: Boosting is beneficial in dealing with missing data. That’s because numerous models are connected sequentially to resolve the issue of missing data.
Examples of Boosting
The AdaBoost leverages the boosting techniques in machine learning, where the model maintenance necessitates less than 50% error. Here, a single learner can either be discarded or kept via boosting. If not, the steps are repeated until a strong learner is achieved.
Given the importance and benefits of bagging and boosting, the technology space is witnessing a surge in ML trends. Want to find out what they are? Check this blog: 5 Trends in Artificial Intelligence and Machine Learning You Should Know About.
Difference Between Bagging and Boosting
Here is a table demonstrating the basic differences between bagging and boosting:
Basis |
Bagging |
Boosting |
Dataset |
Every time it trains the subsequent learner, it increases the dataset weight. |
It trains the models using multiple datasets along with some dataset replacement. |
Working Order |
Parallel homogenous model |
Sequential homogenous model |
Weights |
Observation comes with the same weight |
Observation weight increases when there’s an error detection |
Pro |
Lowers overfitting and variance in machine learning |
Lowers bias in machine learning |
Example |
Random Forest |
AddaBoost |
Similarities Between Bagging and Boosting
Bagging and Boosting in machine learning, both being the popularly used method. There are some prominent similarities between bagging and boosting. Let’s take a look at them:
Bagging Vs Boosting: Similarities |
- Bagging and boosting in machine learning deduce and infer N learners via solitary learner.
- Both bagging and boosting produce multiple training datasets by using random sampling.
- Both assist in producing stabilized models by reducing the model variance.
- To make a final prediction, bagging and boosting depend on aggregating the Majority voting or the N learner’s results.
|
FAQs
When it comes to bagging and boosting, the former resolves over-fitting problems in a model by reducing variance, not bias. However, the latter reduces bias rather than variance. Every model in Bagging is given the same weight. Models are scored in Boosting according to how well they perform.
By using repetitions and combinations to construct several sets of the original data, bagging is a strategy for minimizing prediction variance that produces additional data for training from a dataset.
Boosting is an iterative technique for modifying the weight of an observation in accordance with the previous classification. It tries to give an observation more weight if it was incorrectly classified. In general, boosting produces accurate predictive models.
Two techniques for enhancing the efficacy of a model by altering the input data are bagging and boosting. They enable the combination of outcomes from various iterations of the same model and raise its precision.
Bagging is recommended for use when the model has low bias and high variance. Meanwhile, boosting is recommended when there’s high bias and low variance.
Updated on March 19, 2024