Like masterful alchemists, data scientists weave their magic, transforming raw data into invaluable nuggets of wisdom, from the initial spark of problem definition, where the quest for understanding ignites, to the meticulous collection of data, akin to gathering precious gems from distant lands. The voyage continues through the labyrinth of data exploration, where patterns and trends unveil themselves like ancient secrets waiting to be deciphered. With the artistry of data modelling, mathematical marvels are crafted, breathing life into predictions and prophecies.
As the journey nears its zenith, evaluation becomes the arbiter of truth, ensuring the sanctity of insights gleaned. And finally, like a triumphant crescendo, the fruits of labour are deployed into the world, where they wield the power to shape destinies and illuminate paths forward. As the sun rises on this age of data enlightenment, the demand for these modern-day wizards, the data scientists, is poised to soar, promising a future ablaze with opportunity and discovery. According to the Occupational Outlook Handbook, their ranks are set to swell by a staggering 35% from 2022 to 2032, a testament to their indispensable role in shaping our data-driven world. Join the adventure, and let the Data Science Process be your guide to unlocking the mysteries of our digital universe.
What is Data Science?
Data Science is a field of study that involves extracting results from large amounts of data using various scientific methods, processes and algorithms. It facilitates the uncovering of concealed patterns within raw data. The emergence of the term ‘Data Science’ is attributed to the advancements in mathematical statistics, data analysis, and the advent of big data.
Data Science represents an interdisciplinary domain enabling the extraction of insights from both structured and unstructured data. It empowers individuals to convert a business challenge into a research endeavour, subsequently translating it into a viable solution.
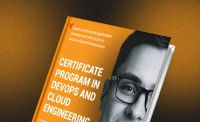
Get curriculum highlights, career paths, industry insights and accelerate your technology journey.
Download brochure
What is the Data Science Process?
The data science process is the systematic journey that converts raw data into actionable insights. Right from identifying the problem, and decoding the data to building models, coming up with the results, and finally deploying solutions, all the steps play a very crucial role in extracting value from the given data.
Components of Data Science Process
No doubt, data science is a very vast field. Therefore, you need to apply different and multiple methodologies and use tools to get the best out of the data you have. Also, you need to make sure that you maintain the integrity of data and keep it private.
Machine Learning and Data Analysis involve concentrating on deriving insights from available data. Conversely, Data Engineering is primarily concerned with ensuring effective data management and establishing seamless data pipelines to facilitate smooth data flow. If we were to delineate the primary elements of Data Science, they would be:
At times, there is no need to apply heavy and advanced learning methods to derive some patterns from the data at hand. In such cases, exploratory data analysis is performed to derive a basic idea. This further helps you understand that do you need to apply any complex and deep learning analysis method or not.
Many real-life datasets often exhibit a normal distribution as a natural occurrence. When we possess knowledge about the distribution a specific dataset follows, it enables comprehensive analysis of its properties in one go. Additionally, descriptive statistics, correlations, and covariances among dataset features contribute to a deeper comprehension of the relationships between different factors within the dataset.
When managing substantial volumes of data, it’s imperative to safeguard it against online threats and ensure seamless accessibility and modifiability. Data Engineers play a vital role in guaranteeing the efficient utilisation of data.
This component of data science has led to new horizons that have helped a lot in building different advanced methodologies and applications, making machines more efficient. Also, this helps you in giving a personalised experience.
This aspect falls within the realm of Artificial Intelligence and Machine Learning, yet it delves deeper into more advanced territory beyond traditional machine learning. The convergence of substantial computing capabilities and vast datasets has fostered the emergence of this domain within data science.
Steps for the Data Science Process
Defining Research Goals and Creating a Project Charter:
- Spend time understanding the goals and context of your research.
- Continuously ask questions and devise examples until the business expectations are clear.
- Create a project charter outlining:
- Clear research goals
- Project mission and context
- Approach for analysis
- Expected resources
- Proof of project feasibility,
- Deliverables and success metrics
- Timeline
Retrieving Data:
- Start with data stored within the company.
- Data may be stored in databases, data marts, data warehouses, or data lakes.
- Accessing data may require time and adherence to company policies.
Cleansing, Integrating, and Transforming Data:
- Cleaning: Remove errors in data to ensure consistency and accuracy.
- Integrating: Combine data from different sources through joining and appending operations.
- Transforming: Restructure data to meet model requirements, including reducing variables and using dummy variables.
Exploratory Data Analysis:
- Take a deep dive into the data to understand its characteristics.
- Utilise graphical techniques such as bar plots, line plots, scatter plots, histograms, etc., to visualise data and identify patterns.
Building Models:
- Develop models aimed at making predictions, classifying objects, or understanding underlying systems.
Presenting Findings and Building Applications:
- Use soft skills to present results to stakeholders effectively.
- Industrialise the analysis process for repetitive use and integration with other tools.
Following these steps ensures a systematic approach to data science projects, leading to meaningful insights and actionable outcomes.
With time, tools used in the Data Science process have evolved.
Various software tools such as Matlab and Power BI, along with programming languages like Python and R, offer a plethora of utility features that enable us to tackle complex tasks efficiently within tight timeframes. Below is an image showcasing some of the popular tools in the field of Data Science.
Use and Benefits of Data Science Process
The Data Science Process offers a structured approach to addressing data-related challenges, providing numerous benefits across various industries. Here’s a closer look at how businesses leverage each step of the process and its associated advantages:
1. Problem Definition:
Use: Clearly define the problem at hand and establish the objectives of the analysis.
Benefits:
- Ensures alignment with business goals.
- Helps in setting clear expectations for outcomes.
2. Data Collection:
Use: Gather data from diverse sources, perform cleaning, and prepare it for analysis.
Benefits:
- Access to comprehensive datasets for analysis.
- Improves data quality and accuracy.
3. Data Exploration:
Use: Explore data to uncover insights, trends, patterns, and relationships.
Benefits:
- Provides valuable insights into data characteristics.
- Identifies potential opportunities and challenges.
4. Data Modeling
Use: Develop mathematical models and algorithms to solve problems and make predictions.
Benefits:
- Enables predictive analytics and decision-making.
- Enhances understanding of complex data relationships.
5. Evaluation:
Use: Assess the performance and accuracy of the model using relevant metrics.
Benefits:
- Validates the effectiveness of the model.
- Facilitates improvements based on feedback.
6. Deployment:
Use: Implement the model in a production environment for real-time predictions or automated decision-making.
Benefits:
- Enables integration into operational workflows.
- Supports scalable and efficient decision-making processes.
7. Monitoring and Maintenance:
Use: Continuously monitor the model’s performance and make necessary updates to maintain accuracy.
Benefits:
- Ensures ongoing relevance and reliability of predictions.
- Mitigates risks associated with model degradation.
Overall, the Data Science Process empowers organisations to derive actionable insights from data, make informed decisions, and drive business success. By following this systematic approach, businesses can harness the full potential of their data assets and stay competitive in today’s data-driven landscape.
Issues/Challenges Faced During Data Science Process
Data Quality and Availability:
- Data must be accurate, complete, and consistent to ensure model accuracy.
- Challenges may arise when required data is not readily available or accessible.
Bias in Data and Algorithms:
- Bias in data due to sampling techniques or measurement errors can impact model accuracy.
- Algorithms may perpetuate societal biases, leading to unfair outcomes.
Model Overfitting and Underfitting:
- Overfitting occurs when a model is overly complex and fails to generalise to new data.
- Underfitting happens when a model is too simple to capture underlying data relationships effectively.
Model Interpretability:
- Complex models can be challenging to interpret, hindering the explanation of model decisions.
- This lack of interpretability can pose obstacles in making informed business decisions.
Privacy and Ethical Considerations:
- Collection and analysis of sensitive personal information raise privacy and ethical concerns.
- It’s crucial to ensure responsible and ethical use of data to address these concerns.
Technical Challenges:
- Technical hurdles like data storage, processing, algorithm selection, and computational scalability may arise.
- Overcoming these challenges requires robust technical expertise and infrastructure.
Wrapping Up
The Data Science Process offers a structured approach to harnessing the power of data, enabling organisations to derive actionable insights and drive strategic decision-making. By following this systematic methodology, businesses can overcome challenges, unlock opportunities, and stay ahead in today’s data-driven world. The benefits are manifold, from improved decision-making and enhanced operational efficiency to innovative product development and increased competitiveness.
To start on a transformative journey into the realm of data science and business analytics, consider enrolling in the Accelerator Program in Business Analytics and Data Science at Hero Vired. With a cutting-edge curriculum, expert faculty, and hands-on learning experiences, this program equips aspiring data professionals with the skills and knowledge needed to thrive in the dynamic field of data science. Don’t miss this opportunity to propel your career forward and become a driving force in the digital age. Join us at Hero Vired and unlock your potential in data science today.
FAQs
The structured framework of five steps, problem definition, approach selection, data gathering, analysis, and interpretation of results, provides a sturdy foundation for navigating the path from inquiry to actionable insights.
A data science lifecycle encompasses the iterative series of steps essential for completing a project or analysis. There is no universal template that delineates data science projects; therefore, it's crucial to identify the approach that aligns best with your business needs. Every stage within the lifecycle demands meticulous execution. The aim of data science is to establish methods for extracting business-centric insights from data. This necessitates comprehending the flow of value and information within a business and leveraging this comprehension to pinpoint potential business opportunities.
The significance of data science lies in its capacity to leverage existing data, which may not hold intrinsic value individually, and amalgamate it with other data points. This process yields insights that organisations can utilise to deepen their understanding of their customers and target audience.
Updated on February 13, 2025