Database Schema is a blueprint for defining data storage, organisation and relationship management strategies. It provides the necessary framework for database design and maintenance purposes, guaranteeing efficient storage and quick retrieval process. Having a good schema in DBMS enhances performance, scalability, easy maintainability and extensibility of the database over time.
In this blog post, we will examine Database Schemas in depth. We will first define them and then discuss their types, advantages, and integration requirements. We will also discuss ways to create a schema for data integration, as well as dimensional schemas and different styles of database schemas.
What is Schema in DBMS?
A database schema is a framework that defines the structure, organisation, and constraints of data stored in a database. It serves as a blueprint for how the database is constructed and how the data within it is related. Here’s a detailed breakdown:
Key Components of a Database Schema
1. Tables:
- Definition: Tables are the basic units where data is stored in rows and columns.
- Attributes: Columns define the type of data stored, such as integers, text, or dates.
- Records: Rows represent individual entries or records within a table.
2. Fields (Columns):
- Data Types: Each field has a specific data type (e.g., integer, varchar, date) that dictates the kind of data it can hold.
- Constraints: Fields can have constraints like primary keys, foreign keys, and unique constraints to enforce data integrity.
3. Relationships:
- Primary Keys: Unique identifiers for records in a table.
- Foreign Keys: Links between tables that establish relationships, ensuring referential integrity.
4. Indexes:
- Purpose: Enhance the speed of data retrieval.
- Types: Common types include primary, unique, and composite indexes.
5. Views:
- Definition: Virtual tables created by querying data from one or more tables.
- Use Case: Simplify complex queries and enhance data security by restricting access to specific data.
Example of a Database Schema
Consider a simple e-commerce database:
- Tables:
- Customers: CustomerID (Primary Key), Name, Email, Address.
- Orders: OrderID (Primary Key), CustomerID (Foreign Key), OrderDate, TotalAmount.
- Products: ProductID (Primary Key), Name, Price, StockQuantity.
- OrderDetails: OrderDetailID (Primary Key), OrderID (Foreign Key), ProductID (Foreign Key), Quantity, Price.
- Relationships:
- Each order is linked to a customer.
- Each order can include multiple products.
- Each product can be part of multiple orders.
Database schemas are crucial for creating well-structured and efficient databases. By defining the organisation and constraints of the data, they ensure that the database operates smoothly and effectively.
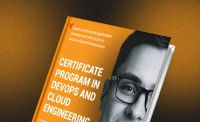
Get curriculum highlights, career paths, industry insights and accelerate your technology journey.
Download brochure
Database schema vs. database instance
Feature |
Database Schema |
Database Instance |
Definition |
The blueprint of the database structure, including tables, fields, relationships, and constraints. |
The actual content of the database at a particular point in time, including data in tables. |
Nature |
Static, defines the design and structure of the database. |
Dynamic, changes as data is added, updated, or deleted. |
Purpose |
To provide a clear structure and organisation for data storage. |
To represent the current state of the data stored in the database. |
Components |
Includes tables, fields, data types, relationships, indexes, and views. |
Consists of records or data entries in tables as per the schema. |
Persistence |
Remains constant unless the database structure is altered through DDL operations. |
Continuously changes with data manipulation operations (INSERT, UPDATE, DELETE). |
Examples |
Defining a table of Customers with fields CustomerID, Name, Email, and constraints. |
A table of customers containing entries like 1, John Doe, john@example.com. |
Management |
Managed using Data Definition Language (DDL) commands like CREATE, ALTER, and DROP. |
Managed using Data Manipulation Language (DML) commands like INSERT, UPDATE, and DELETE. |
Focus |
Focuses on how data should be organised and related within the database. |
Focuses on the actual data values and their current state within the database. |
Types of database schemas
Database schemas come in various types, each serving different purposes and providing different levels of abstraction. Understanding these types helps in designing and managing databases effectively.
Physical Schema
A physical schema describes how data is physically stored in the database. It involves the actual storage mechanisms, such as files, indices, and storage devices. This schema is concerned with how the data is organised on the hardware and the techniques used to optimise storage and retrieval.
- Storage Structure: Defines how data is stored on physical storage media.
- Indexing: Describes the use of indices to speed up data retrieval.
- Partitioning: Involves dividing the database into parts to improve performance and manageability.
- Hardware Configuration: Specifies the setup of hardware resources like disks and memory.
Logical Schema
A logical schema in DBMS outlines the logical design of the database, focusing on the structure without considering physical aspects. It details tables, fields, data types, and relationships, defining how the data is logically organised and related.
- Tables and Fields: Specifies the tables and their respective fields.
- Data Types: Defines the types of data each field can hold (e.g., integers, strings).
- Relationships: Establishes relationships between different tables (e.g., primary and foreign keys).
- Constraints: These include rules like unique constraints and check constraints to ensure data integrity.
Conceptual Schema
A conceptual schema provides a high-level overview of the entire database structure. It abstracts the logical schema and presents an overall picture of the database without getting into implementation details.
- Overall Structure: Represents the overall logical structure of the database.
- Abstracted View: Provides an abstract view, hiding implementation complexities.
- Data Model: Includes entities, relationships, and constraints at a high level.
Each type of database schema plays a crucial role in the design, management, and use of a database. Understanding these types helps in effectively planning and implementing database solutions that are efficient, scalable, and secure.
Benefits of database schemas
Database schemas provide a structured framework for organising and managing data within a database. They offer numerous benefits that enhance the efficiency, performance, and security of database systems.
Data Integrity
Database schemas ensure data integrity by maintaining consistency and accuracy across the database. They enforce data types and constraints, preventing the entry of invalid data. Relationships such as primary and foreign keys help maintain referential integrity, ensuring that data remains reliable and interconnected.
Improved Performance
Schemas facilitate query optimization by organising data efficiently. This structure, combined with the use of indices, speeds up data retrieval and reduces query response times. Additionally, partitioning large tables into manageable parts enhances overall performance, making the database more efficient.
Scalability
With a modular design, database schemas allow for easy scaling as data volumes grow. They provide the flexibility to add new tables and fields without disrupting existing data. This adaptability helps manage storage capacity and resource allocation effectively, ensuring the database can grow alongside business needs.
Easier Maintenance
A clear and well-defined schema simplifies database management. It makes implementing updates and upgrades straightforward and helps in troubleshooting by making it easier to identify and fix issues within the database. This structured approach reduces the complexity of maintaining the database over time.
Enhanced Security
Database schemas enhance security by restricting access to sensitive data through user-specific views and permissions. Data masking techniques can hide certain data elements to protect privacy, and audit trails track changes and access to the data, facilitating security audits and ensuring compliance with regulations.
Better Data Management
Schemas organise data logically, making it easier to store and retrieve information. They enforce standardisation, ensuring that data follows a consistent format, which improves data quality. Detailed schema descriptions aid in documentation and understanding, making data management more efficient.
Facilitates Data Integration
A well-defined schema in DBMS integrates various data sources to provide a unified view of information. This interoperability between different systems and applications simplifies data migration processes. By ensuring that data can be seamlessly transferred and combined, schemas support comprehensive data integration efforts.
Support for Business Intelligence
Schemas support advanced analytics by providing a structured data framework that improves the accuracy and efficiency of business reports. They facilitate better decision-making by ensuring that reliable and well-organised data is available for analysis. This support is crucial for businesses aiming to leverage data for strategic insights.
How to design a schema for data integration
Designing a schema for data integration involves several critical steps to ensure that data from different sources can be combined into a cohesive, functional, and efficient database.
Here’s a detailed guide on how to achieve this:
1. Define Integration Objectives
Before starting, clearly define the goals and objectives of the data integration:
- Purpose: Determine why you need to integrate the data and what outcomes you expect.
- Scope: Identify the data sources and the specific datasets that need to be integrated.
- Requirements: List the functional and nonfunctional requirements, such as performance, security, and scalability.
2. Analyze Source Data
Thoroughly analyse the data sources to understand their structure and content:
- Data Structure: Study source databases’ schemas, including tables, fields, data types, and relationships.
- Data Quality: Look at the validity of the facts, considering any inconsistencies, duplicates or missing values.
- Compatibility: Check for compatibility problems like different data types, and naming conventions.
3. Create a Unified Data Model
Develop a unified data model that can accommodate all data sources:
- Entity-Relationship Diagram (ERD): Use ERD to show entities’ attributes and relations in the unified schema.
- Normalisation: Normalise your information so that there is no redundancy or ambiguity in your work.
- Denormalization: In some cases, it denormalises data for performance optimization by maintaining an equilibrium between normalisation and denormalization.
4. Define Data Mapping and Transformation Rules
Establish rules for mapping & transforming source schema’s data into target schema:
- Data Mapping: Map out fields from source schemas onto the target one, ensuring that even their properties match with each other.
- Transformation Rules: Define some rules about making transformations to such datasets like changing variable types joining variables or splitting variables apart.
- Data Cleansing: Cleaning the dataset should also include things such as deleting duplicates or correcting errors.
5. Design the Integrated Schema
Design the schema that will house the integrated data:
- Tables and Fields: Define the tables and fields, including data types and constraints.
- Primary and Foreign Keys: Establish primary and foreign keys to maintain relationships between tables.
- Indexes: Create indexes to improve query performance.
- Views: Define views to simplify access to the integrated data for different user groups.
Styles of database schemas
Database schemas come in various styles, each with unique structures and uses. Understanding these styles and their examples can help in selecting the right schema for specific database needs.
Flat Model
The flat model is the simplest form of a schema in DBMS. All records are stored in one single two-dimensional table. One row represents one record, and one column represents an attribute of the data. Although easy to understand and use, this model does not support complex data relationships.
For instance, a spreadsheet containing customer contact details (names, addresses, phone numbers) can be thought of as a flat model.
Hierarchical Model
The hierarchical model organises data in a tree-like structure, with a single root and multiple levels of nested nodes. Each node represents a record, and edges define parent-child relationships. This model is efficient for representing data with a clear hierarchy, such as organisational structures or file systems.
For instance, an employee database where each employee has one direct manager can be represented in a hierarchical model, with the company CEO at the top and various levels of management below.
Network Model
The network model is an extension of the hierarchical model that allows more complex relationships by enabling nodes to have multiple parent and child nodes. Here, records are represented as nodes with relationships between them as edges in a graph. It is good for many-to-many relations, and complex data structures can be easily represented using it.
As an illustration, a university course registration system where students can enrol in many courses and each course can have many students best exemplifies this schema.
Relational Model
The relational model is the most widely used database schema style. A relational schema organises data into relations or tables made up of rows and columns in which each table has only one unique key field but at least two other fields that refer to foreign keys. SQL (Structured Query Language) is the language supporting querying as well as manipulating data on this system.
As an example, consider an e-commerce website’s database consisting of customers’ information, orders’ details, and product details with connections between these tables indicating which customer ordered what product.
Star Schema
The star schema is a type of dimensional schema commonly used in data warehousing and business intelligence. It consists of a central fact table connected to multiple dimension tables. The fact table contains quantitative data, while dimension tables hold descriptive data related to the dimensions of the business.
An example of this schema is a sales database where the fact table contains sales data (e.g., sales amount, transaction date), and dimension tables include information on customers, products, and time periods.
Snowflake Schema
The snowflake schema is a more normalised version of the star schema. In this schema, dimension tables are further broken down into related tables, reducing redundancy and saving storage space.
An example of a snowflake schema is an extended version of the sales database, where the customer dimension is split into separate tables for customer demographics, addresses, and contact information. This structure improves data organisation but can lead to more complex queries due to the additional joins.
Conclusion
Database schemas are fundamental to the effective organisation, management, and integration of data within a database system. They define the structure and constraints of data, ensuring that it is stored efficiently, accessed quickly, and maintained easily.
From understanding the different types of schemas to learning how to design a schema for data integration, this guide provides a comprehensive overview of everything you need to know about database schemas. By implementing well-designed schemas, businesses can enhance data integrity, improve performance, and support advanced analytics, ultimately leading to better decision-making and business insights.
FAQs
A database schema is a blueprint that defines the structure of a database, including tables, fields, relationships, and constraints.
A database schema is a static blueprint of the database's structure, while a database instance is the actual data stored in the database at a specific point in time.
The main types of database schemas include physical schema, logical schema, and conceptual schema.
A star schema is a type of dimensional schema used in data warehousing that consists of a central fact table connected to multiple dimension tables.
A snowflake schema is a more normalised version of a star schema, where dimension tables are further broken down into related tables to reduce redundancy.
Data integrity ensures that the data is accurate, consistent, and reliable, which is crucial for maintaining the trustworthiness of the database.
The relational model offers flexibility, supports SQL for data manipulation, and allows the definition of complex relationships between data tables.
Updated on August 22, 2024