Ever wondered why your database queries run slow or return unexpected results? We all want our databases to be fast and reliable.
That’s where join dependencies in DBMS come in.
Join dependencies ensure our tables are correctly joined, keeping our data accurate and our queries efficient.
A join dependency in DBMS means that a table can be recreated by joining multiple smaller tables, ensuring the integrity and consistency of the data.
Let’s dive into this essential concept and see how it can improve our database management.
Types of Join Dependency in DBMS
Join dependency can be sub-categorised into two types; understanding the difference between these types helps us design better databases.
Lossless Join Dependency
A lossless join dependency ensures that when we join tables, no data is lost. This is crucial because losing data means losing valuable information.
For example, imagine a library database. If we split the book and borrower information into separate tables, a lossless join ensures we can still see who borrowed which book without losing any details.
Lossy Join Dependency
On the other hand, a lossy join dependency means some data might be lost during the join. This can lead to inaccuracies and incomplete data.
It’s like tearing a photo into pieces and not being able to put it back together perfectly. Some parts might be missing.
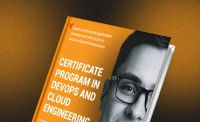
Get curriculum highlights, career paths, industry insights and accelerate your technology journey.
Download brochure
Detailed Explanation of Join Dependency with Unique Examples
Let’s look at two unique examples to understand join dependency better.
Example 1: Library Management System
In a library management system, we have three tables:
- Books: Book_ID, Title, Author
- Borrowers: Borrower_ID, Name
- Transactions: Book_ID, Borrower_ID, Date_Borrowed
Here’s how join dependency works:
1. Books Table:
Book_ID |
Title |
Author |
1 |
1984 |
Orwell |
2 |
Hamlet |
Shakespeare |
2. Borrowers Table:
Borrower_ID |
Name |
1 |
Sonam |
2 |
Shivani |
3. Transactions Table:
Book_ID |
Borrower_ID |
Date_Borrowed |
1 |
1 |
2023-01-01 |
2 |
2 |
2023-02-01 |
When we join these tables using Book_ID and Borrower_ID, we get:
Book_ID |
Title |
Author |
Borrower_ID |
Name |
Date_Borrowed |
1 |
1984 |
Orwell |
1 |
Sonam |
2023-01-01 |
2 |
Hamlet |
Shakespeare |
2 |
Shivani |
2023-02-01 |
This join shows the complete transaction history without losing any data. It’s a perfect example of a lossless join.
Example 2: Online Course Enrollment System
In an online course enrollment system, we have three tables:
- Courses: Course_ID, Course_Name, Instructor
- Students: Student_ID, Student_Name
- Enrollments: Course_ID, Student_ID, Enrollment_Date
Here’s how join dependency works:
1. Courses Table:
Course_ID |
Course_Name |
Instructor |
101 |
Math |
Dr. Govinda |
102 |
Science |
Dr. Salman |
2. Students Table:
Student_ID |
Student_Name |
1 |
Ranveer |
2 |
Arjun |
3. Enrollments Table:
Course_ID |
Student_ID |
Enrollment_Date |
101 |
1 |
2023-03-01 |
102 |
2 |
2023-04-01 |
When we join these tables using Course_ID and Student_ID, we get:
Course_ID |
Course_Name |
Instructor |
Student_ID |
Student_Name |
Enrollment_Date |
101 |
Math |
Dr. Govinda |
1 |
Ranveer |
2023-03-01 |
102 |
Science |
Dr. Salman |
2 |
Arjun |
2023-04-01 |
This join ensures we see who enrolled in which course and when. Again, we retain all data, showing a lossless join.
What if your database could handle complex queries without redundancy? That’s where the Fifth Normal Form (5NF) steps in.
5NF is like the gold standard for database normalisation, and it’s deeply tied to join dependency in DBMS.
5NF is all about breaking down data into the smallest pieces that can be reassembled without any redundancy. It ensures that every piece of data is stored just once, making your database lean and efficient.
So, how does join dependency fit into this?
How Does Join Dependency Relate to 5NF?
Join dependency in DBMS directly connects to 5NF. If a table has a join dependency, it can’t be in 5NF unless that dependency is handled.
When we talk about 5NF, we’re looking at the most refined version of data normalisation. It means that every join in your table should reconstruct the original table without creating unnecessary data.
When we break down a table into smaller tables, each representing a unique relationship, join dependency ensures we can still piece those tables back together without losing any data.
If a table satisfies all the conditions for 4NF but still has complex join dependencies, it isn’t in 5NF.
To move to 5NF, we decompose the table further, ensuring that these dependencies are managed correctly.
Here’s a quick example.
Imagine a student database where students enrol in courses taught by multiple instructors. We might start with a single table like this:
Student_ID |
Course_ID |
Instructor_ID |
1 |
101 |
501 |
2 |
102 |
502 |
1 |
102 |
503 |
To satisfy 5NF, we need to decompose this table into three separate tables:
1. Students and Courses:
Student_ID |
Course_ID |
1 |
101 |
2 |
102 |
2. Courses and Instructors:
Course_ID |
Instructor_ID |
101 |
501 |
102 |
502 |
3. Students and Instructors:
Student_ID |
Instructor_ID |
1 |
501 |
2 |
502 |
Now, by joining these tables, we can recreate the original table.
But more importantly, we avoid redundancy and ensure that the data is stored in its most efficient form. This is how join dependency drives us towards achieving 5NF.
Understanding Trivial Join Dependencies and Their Importance
Sometimes, a join dependency doesn’t require us to break down a table. This happens when one of the tables in the join dependency is identical to the original table.
In this case, we say the join dependency is trivial.
For example, if we have a table like:
Employee_ID |
Department |
1 |
IT |
2 |
HR |
And a join dependency suggests breaking it into:
- Employee_Department: Employee_ID, Department
Here, the join dependency is trivial because the original table is the same as the joined tables.
So, what’s the big deal?
Trivial join dependencies might seem harmless, but they can lead to unnecessary joins and over-complication. We want to avoid them to keep our databases simple and efficient.
When designing a database, we should aim to identify and eliminate trivial join dependencies. This keeps our data structure clean, making it easier to manage and faster to query.
How Join Dependencies Help Maintain Data Integrity
Data integrity is the backbone of any database. Without it, our data can quickly become unreliable, leading to mistakes and inefficiencies.
Join dependencies in DBMS play a crucial role in maintaining this integrity.
Why Does Data Integrity Matter?
Data integrity ensures that our data remains accurate and consistent. It prevents anomalies, like missing or duplicated data, which can cause problems down the line.
For example, imagine a retail database where customer orders are linked to products and shipments.
If the join dependency isn’t handled correctly, we could end up with orders that don’t match shipments, leading to unhappy customers.
Join Dependencies and Accurate Data
Join dependencies keep our data intact during operations like splitting tables and joining them back together. This is especially important in large databases where data is frequently updated or queried.
Here’s how it works.
When we decompose a table into smaller tables based on join dependencies, we ensure that each piece of data is stored in only one place. This reduces redundancy and keeps our data consistent.
For example, in an employee database, we might have tables for departments, employees, and projects.
If these tables are designed with join dependencies in mind, we can be confident that an employee’s department or project assignment won’t be recorded incorrectly.
Real-World Example: Banking System
Consider a banking system where customers have accounts linked to transactions.
If we don’t maintain join dependencies properly, a customer’s account could show the wrong balance or transaction history.
By following join dependency rules, we ensure that every transaction is accurately linked to the correct account. This keeps the system reliable and prevents costly errors.
Steps to Maintain Data Integrity with Join Dependencies
- Identify Join Dependencies: Determine how your tables are related and where join dependencies exist.
- Decompose Tables: If needed, break down larger tables into smaller ones to remove redundancy.
- Test for Lossless Joins: Ensure that joining the decomposed tables gives you back the original data without any loss.
- Regularly Review Database Design: As your database grows, revisit the design to ensure that join dependencies still hold true.
The Role of Join Dependencies in Query Optimization
When we break down a table into smaller tables using join dependencies, we reduce the amount of redundant data.
Less redundancy means smaller tables, which leads to faster queries. Here’s how:
- Faster Access: Smaller tables are quicker to scan. This means the database can retrieve the needed information faster.
- Efficient Indexing: With reduced redundancy, indexes become more effective, further speeding up query execution.
- Reduced I/O Operations: By minimising the size of data to be processed, join dependencies lower the number of input/output operations, which are often the slowest part of database queries.
Practical Example: Retail Database
Consider a retail database where products, orders, and customers are all linked.
Without considering join dependencies, a single query to retrieve customer orders might have to sift through a massive table filled with redundant data.
By applying join dependency, we can split this data into smaller, more manageable tables.
For instance:
1. Products Table:
Product_ID |
Product_Name |
Price |
1 |
Laptop |
1000 |
2 |
Phone |
500 |
2. Customers Table:
Customer_ID |
Customer_Name |
101 |
Dolly |
102 |
Pratik |
3. Orders Table:
Order_ID |
Product_ID |
Customer_ID |
Order_Date |
1001 |
1 |
101 |
2024-01-01 |
1002 |
2 |
102 |
2024-02-01 |
Now, when we query for Alice’s orders, the database only needs to look through the smaller Orders and Customers tables instead of a massive, monolithic table.
This leads to faster and more efficient query performance.
Steps to Use Join Dependencies for Optimising Queries
- Identify Redundant Data: Start by pinpointing tables where data is repeated unnecessarily.
- Decompose Tables: Break down these tables into smaller ones using join dependency principles.
- Index Key Columns: Ensure that key columns used in joins are indexed for faster access.
- Test Query Performance: Run queries before and after the changes to measure performance improvements.
By following these steps, we can ensure that our database queries run as efficiently as possible.
Practical Applications and Real-World Scenarios Involving Join Dependency
Join dependency isn’t just a theoretical concept. It has real-world applications that can make a big difference in how our databases perform.
Example: Healthcare Database
In a healthcare system, patient records are linked to doctors, treatments, and prescriptions. Without join dependencies, queries to retrieve a patient’s full medical history could be painfully slow.
By applying join dependency, we can break down the patient data into smaller tables:
1. Patients Table:
Patient_ID |
Patient_Name |
DOB |
1 |
Srishti |
1980-05-10 |
2 |
Riya |
1975-09-21 |
2. Doctors Table:
Doctor_ID |
Doctor_Name |
Specialty |
201 |
Dr. Ankit |
Cardiology |
202 |
Dr. Virat |
Neurology |
3. Treatments Table:
Treatment_ID |
Patient_ID |
Doctor_ID |
Treatment_Date |
3001 |
1 |
201 |
2024-01-15 |
3002 |
2 |
202 |
2024-02-10 |
This structure allows the healthcare database to quickly retrieve patient records, ensuring that doctors have all the information they need without delay.
Example: Financial Services
In a financial services firm, data about clients, accounts, and transactions is linked. A poorly designed database might store all this information in one giant table.
But with join dependencies, we can split it into:
- Clients Table: Client details like name and contact information.
- Accounts Table: Account details linked to clients.
- Transactions Table: Transaction records linked to accounts.
This reduces redundancy and speeds up queries when, for example, retrieving a client’s transaction history.
These practical examples show how join dependency in DBMS can be applied to make databases more efficient in real-world scenarios.
Conclusion
Join dependency in DBMS is a vital concept that directly impacts the efficiency and integrity of our databases.
The relationship between join dependency and the Fifth Normal Form (5NF) highlights the importance of decomposition in achieving a fully normalised database. This not only ensures data integrity but also makes our queries faster and more efficient.
Practical applications in various fields, from healthcare to finance, demonstrate the real-world benefits of properly managing join dependencies, making our databases not just functional but truly optimised.
By understanding how join dependencies work, we can structure our databases to minimise redundancy, optimise query performance, and maintain accurate data.
FAQs
Join dependency involves relationships between multiple tables, ensuring they can be joined without data loss. Functional dependency, on the other hand, deals with relationships within a single table, ensuring that a particular column is uniquely determined by another column.
Join dependency reduces redundancy and breaks down large tables into smaller ones. This minimises the data that needs to be scanned during a query, speeding up the query execution process.
A trivial join dependency occurs when one of the smaller tables in the join dependency is the same as the original table. In this case, no further decomposition is needed.
Join dependency helps maintain data integrity by ensuring that data remains consistent and accurate when tables are joined. This prevents issues like missing or duplicate data.
Challenges include identifying where join dependencies exist, determining the best way to decompose tables, and ensuring that the decomposition doesn’t lead to data loss or overly complex queries.
Updated on August 9, 2024