Developments in artificial intelligence (AI) are so fast-paced that keeping track of the new changes can be a little tricky. But if you want to begin from scratch, you will have to divide the whole genre into two broad streams – machine learning (ML) and deep learning. These two terms are often used interchangeably and have become buzzwords. However, it is equally important to understand their differences to have a hold over artificial intelligence. Owing to the enormous demand for artificial intelligence, AI and machine learning programs have swamped the internet. Several online learning platforms are offering online certificate programs for young professionals to get ahead in the competition. But before going there, it is always wise to do your homework.
Artificial intelligence has revolutionized human lives like none other. From getting apt recommendations on Netflix to self-driving cars, nothing is impossible with AI. Hence, several companies are employing AI for solutions related to their industry. The aim is to enhance customer satisfaction and make every activity automated, seamless, and hassle-free. Given this situation, there is a huge demand for professionals trained in machine learning. Check out our Artificial Intelligence and Machine Learning Course.
Let us try and understand a little more about the major difference between machine learning and deep learning.
What is Machine Learning?
In simple words, machine learning is a branch of AI which includes algorithms that analyze data and learn from it to carry out informed functions without human involvement. For example, in an on-demand music streaming service, the algorithm recommends new songs to listeners by drawing in data from other listeners that have similar choices. The automated recommendations on Facebook, Netflix, and Spotify, are a result of machine learning algorithms. However, it involves a lot of complicated mathematical calculations and coding.
Functions of Machine Learning
There are several factors about machine learning vs deep learning that make them suitable for different applications. The functions of machine learning are:
- Supervised Learning – In this type of learning, the machine learning model is trained on labeled data, where the desired output is known.
- Unsupervised Learning – In this type of learning, the machine learning model is trained on unlabeled data, where the desired output is not known.
Applications of Machine Learning
Machine learning has several applications, including image recognition, natural language processing, and fraud detection. Explore more about What is Bagging vs. Boosting in Machine Learning?
Examples of Machine Learning Algorithms
There are several machine learning algorithms, including
- linear regression
- Decision trees,
- Random forests.
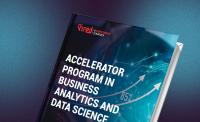
Get curriculum highlights, career paths, industry insights and accelerate your data science journey.
Download brochure
What is Deep Learning?
Deep learning is a sub-branch of machine learning that uses algorithms in multiple layers to create artificial neural networks. Deep learning is the sophisticated programming of machines that allows them to think just like a human brain. Multidimensional and complex deep neural networks are created that exchange data just like neurons of a human brain.
Deep learning is a tremendous task that involves massive amounts of data. However, once it is put in place, there is no requirement for human intervention at all.
Functions of Deep Learning
One of the main differences between machine learning and deep learning (machine learning vs deep learning) are their functions:
- Feature Extraction – DL learns to extract relevant features from the input data by passing it through several layers of artificial neurons.
- 2. Classification – DL uses the extracted features to classify the input data into one or more categories.
Applications of Deep Learning
When it comes to machine learning vs deep learning, deep learning has several applications, including image and speech recognition, self-driving cars, and natural language processing. Read about Understanding the Difference Between Artificial Intelligence and Machine Learning?
Examples of Deep Learning Algorithms
There are several DL algorithms, including
- Convolutional neural networks (CNNs)
- Recurrent neural networks (RNNs)
Differences between Machine learning and Deep learning (Machine learning vs Deep learning)
Let’s understand the major difference between Deep learning vs Machine Learning:
Criteria |
Machine Learning |
Deep Learning |
Problem-solving Approach |
Deductive |
Inductive |
Applications |
Structured data applications |
Complex applications |
Feature Engineering |
Manual feature engineering required |
Less manual feature engineering required |
Suitable For |
Small to medium-sized datasets |
Large and complex datasets |
Type of Data |
Structured |
Structured and unstructured |
Execution Time |
Faster |
Slower |
Model Complexity |
Simple |
Complex |
Data Dependency |
Dependent on data quality and quantity |
Less dependent on data quality and quantity |
Performance |
Less accurate |
More accurate |
Interpretation of Results |
Easily interpreted |
Difficult to interpret |
Problem-solving Approach |
Deductive approach |
Inductive approach |
Hardware Requirements |
Simple hardware (CPUs) |
Powerful hardware (GPUs) |
|
It uses algorithms to study data, learn from it, and make better decisions based on the learnings. |
It organises algorithms in several layers to create an “artificial neural network” that can learn and decide by itself. |
Data |
It can work with a lesser amount of data |
It requires massive volumes of data. |
CPU |
It requires a CPU for training. |
It requires a CPU for training. |
Software Requirements |
Simple software libraries |
Specialized software libraries |
Machine learning vs Deep learning – Accuracy and Efficiency
There are several differences between Machine learning vs Deep learning in terms of accuracy and efficiency, let’s explore them:
- When it comes to Machine learning vs Deep learning, DL algorithms tend to be more accurate than traditional ML algorithms.
- DL algorithms require more computational resources and take longer to train, which makes them less efficient than traditional ML algorithms.
Machine learning vs Deep learning – Hardware and Software Requirements
There are several differences between Machine learning vs Deep learning in terms of software requirements, let’s explore them:
- In terms of Machine Learning vs Deep Learning, DL algorithms require more powerful hardware, such as GPUs, to train and execute.
- In contrast, traditional ML algorithms can be executed on simpler hardware, such as CPUs.
Conclusion
In conclusion, Machine Learning vs Deep Learning are two powerful technologies that have transformed the field of artificial intelligence. While they have many similarities, they also have several key differences that make them suited for different applications. Also read about 14 Machine Learning in Healthcare Examples to Know.
Hero Vired is a reputed online learning platform that offers the best machine learning online program in collaboration with the Massachusetts Institute of Technology (MIT). Learners can take up this 11-month long program in both full-time and part-time modes of learning. To find out more about this, visit the Hero Vired website today.
FAQs
Major differences between machine learning vs deep learning are that DL is a subset of ML.
Machine learning vs Deep learning: ML can be used for smaller data and DL for bigger.
The future of Machine Learning vs Deep Learning looks promising. With the advent of big data and advancements in hardware and software technology, the potential applications of machine learning and deep learning are limitless.
The choice between Machine Learning vs Deep Learning depends on the problem at hand. If the problem requires structured data and relatively simple models, then traditional machine learning algorithms may be sufficient.
Updated on March 19, 2024