Reinforcement learning is a part of Machine Learning, taking suitable actions to maximize rewards in a scenario. Various machines and software employ it to discover the best path or behavior that it must take in a specified scenario. This post narrates everything about reinforcement learning, its advantages, disadvantages, application, how it works, and how it differs from supervised learning. Let’s dive into the post to get a detailed understanding.
What is Reinforcement Learning?
Reinforcement Learning (RL) is a subfield of machine learning where an agent learns to make sequential decisions by interacting with an environment. It is a feedback-based ML technique where the agent learns how to behave in a scenario by performing actions and checking their results. In short, reinforcement learning in machine learning allows the agent to learn using feedback without labeled data automatically. Reinforcement Learning can solve a specified type of issue where decision-making has to be sequential, and the goal must be long-term. The best reinforcement learning examples and applications include robotics, game-playing, and more.
Learn more about Machine learning models here.
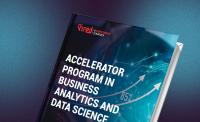
Get curriculum highlights, career paths, industry insights and accelerate your data science journey.
Download brochure
How does Reinforcement Learning Work?
In Reinforcement Learning, developers formulate a methodology to reward the desired behavior and punish negative behavior. The method can assign positive values to desired actions, thereby encouraging agents and negative values to the undesired behavior. It programs the agent to find long-term rewards and achieve a solution.
The long-term objectives prevent agents from stalling on the lesser goals. Gradually, the agent learns how to avoid negative and seek positive goals and methods. This practice is adopted in AI as a fundamental mode to direct unsupervised ML via penalties and rewards.
4 Key Features of Reinforcement Learning
Here’s presenting the key features of reinforcement learning:
- The agent isn’t instructed about the overall actions to be implemented
- Includes a hit and trial practice
- An agent can take actions as per the previous action’s feedback
- The agent might receive a delayed reward
The environment is stochastic, so the agent must explore it for maximum positive rewards.
Outlining the 4 Types of Reinforcement Learning
The following are the 4 major types of reinforcement learning:
- Negative reinforcement: This includes removing something in order to increase response. The individual should remain motivated until the job’s end to get the payment.
- Positive reinforcement: This includes adding something to increase the response, like praising a kid when he/she completes the designated task. It means motivating the kid to engage in the work.
- Extinction: It is all about removing something in order to modify a response. It is also termed negative punishment.
- Punishment: It is all about adding something aversive and modifying the behavior.
4 Core Elements of Reinforcement Learning
The following points present the elements of reinforcement learning:
- Policy
A policy is a method explaining the way the agent behaves at a particular time. By mapping the perceived state of the environment, it takes action on the states. A policy is a fundamental component of reinforcement learning because it can demonstrate the agent’s behavior. In a few circumstances, it might be a function/lookup table, while in other cases, it might involve computation, like a search process. This could be stochastic or even deterministic policy.
- Reward Signal
The next is the reward signal, where the objective of Reinforcement Learning is demonstrated by a reward signal. The environment at every state sends an instant signal to a learning agent. This signal is the reward signal. The prime goal here is maximizing the rewards for good actions. A reward signal might alter the policy. For instance, the time when the action chosen by the agent contributes to a low reward. In such a case, the policy may change to choose other actions in the future.
- Value Function
In the value function, information is received about how excellent the situation or action is. It involves describing the rewards that an agent may expect. While a reward is an immediate signal for every bad or good action, the value function assesses the good action or state for the future. It is based on the reward because, without rewards, no value can be achieved. The prime objective of estimating the values is to achieve rewards.
- Model
Model is the last Reinforcement Learning element, mimicking the environment’s behavior. It makes inferences about how an environment shall behave. For example, when the state and action are given, the model may predict the reward and states.
Evaluating the Advantages of Reinforcement learning
Now, coming to the advantages of reinforcement learning, the following points describe its benefits:
- Reinforcement learning is used for solving extremely complicated issues that might not be solved by any traditional technique
- The model may correct errors occurring during training
- Reinforcement Learning involves training data obtained through direct interactions between the agent and the environment
- Reinforcement Learning may also handle non-deterministic environments, which means that the actions’ outcomes cannot be predicted. It is valuable in real-world applications where the environment changes over a timeframe
- Reinforcement Learning is used even for solving different problems like the ones that include control, optimization, and decision-making
- Another benefit of reinforcement learning is that it is a flexible practice that can be combined with ML techniques, including deep learning, to improve the overall performance
Understanding the Disadvantages of Reinforcement learning
Look for the disadvantages of Reinforcement Learning in the following points:
- Reinforcement Learning isn’t preferable for solving simplified problems
- Reinforcement learning requires an excessive amount of data and computation
- It is dependent on the reward function’s quality. So, when the reward function is designed poorly, it becomes difficult for the agent to learn the behavior
- Debugging and interpreting are complicated tasks for Reinforcement Learning. Since it is clear why an agent behaves in a particular way, it makes it more complicated for it to diagnose and troubleshoot problems.
Common Application of Reinforcement Learnings
The following are the applications of reinforcement learning:
- Robotics with pre-programmed behavior can be valuable in structured environments, including an assembly line of an automobile manufacturing plant (which involves repetitive tasks)
- The master chess player makes a move, where the choice is well-informed by planning, envisioning replies, as well as counter replies
- The adaptive controller adjusts the parameters of the petroleum refinery’s operation, which occurs in real-time
Difference between Reinforcement learning and Supervised learning:
The following is a tabulated version presenting the differences between reinforcement learning and supervised learning:
Reinforcement Learning |
Supervised Learning |
RL interacts with the environment. |
Supervised learning only works on existing datasets. |
Reinforcement learning algorithm works like human brains when making decisions |
Supervised learning works in such a manner that a human is learning under the guidance of someone or something |
RL does not include any labeled dataset |
SL includes labeled dataset |
It does not offer any previous training to learning agents. |
Training will be provided to algorithms such that it predicts outputs easily |
RL can take decisions in a sequential manner. |
In SL, decisions will be made only of the input is already given. |
Read More: Major Differences Between Data Science and Artificial Intelligence
Conclusion
So, this post has narrated what reinforcement learning is, its advantages, disadvantages, applications, and the difference between SL and RL. Basically, In RL, the agent takes actions in the environment, receives feedback in the form of rewards or penalties, and uses this feedback to adjust its decision-making strategy. Hope this guide helped you understand Reinforcement Learning in more detailed and better way.
Hero Vired allows you to upskill your career via reinforcement learning practices. Discover the Artificial Intelligence and Machine Learning course today.
FAQs
The key elements of reinforcement learning include the following:
- Policy
- Reward Signal
- Value Function
- Model
Policy gradient methods in reinforcement learning are one of its types that rely on optimizing parametrized policies in accordance with expected returns (the long-term cumulative reward) by gradient descent.
Temporal Difference or TD Learning is the
unsupervised learning practice. It is used in reinforcement learning for anticipating the total expected reward over the future. In addition, they are also used for predicting other quantities too.
Reinforcement Learning has revolutionized the gaming universe as it enables game agents to play complicated games with human-like performance. RL can also be used for robotic control to let robots perform tasks like navigating environments, grasping objects, and more.
Reinforcement Learning can be used in real-world scenarios like gaming, traffic control, automated robots, energy conservation, image processing, and more.