When it comes to efficient data retrieval, binary search trees are a popular choice. However, not all binary search trees are created equal. An Optimal Binary Search Tree (OBST) is a specialized version that minimizes the cost of search operations, making it highly efficient. In this blog, we will delve into the key concepts of an Optimal Binary Search Tree, understand the algorithm used to construct it, explore dynamic programming as a powerful approach to solving it, and analyze its complexity.
Additionally, we will explore different approaches to constructing an OBST and highlight its advantages over conventional binary search trees.
What is an Optimal Binary Search Tree?
An Optimal Binary Search Tree is a variant of binary search trees where the arrangement of nodes is strategically optimized to minimize the cost of searches. The key idea behind an OBST is to place frequently accessed items closer to the root, reducing the search time for those elements. Conversely, less frequently accessed items are placed further away, leading to a balanced and efficient search tree.
Understanding Threaded Binary Trees is essential for working with Optimal Binary Search Trees. So, you must understand the concept of threads.
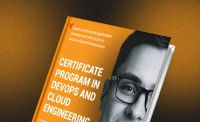
Get curriculum highlights, career paths, industry insights and accelerate your technology journey.
Download brochure
Optimal Binary Search Tree Algorithm
The optimal binary search tree algorithm involves a dynamic programming approach. The fundamental principle that drives the algorithm is “optimal substructure.” It means that the overall optimal solution can be derived from the optimal solutions of its subproblems.
Examples of Optimal Binary Search Tree
Let’s consider an OBST example scenario where we have the following items along with their associated probabilities of access:
Items: [A, B, C] Probabilities: [0.2, 0.3, 0.5]
Using the optimal binary search tree algorithm, we can construct the optimal binary search tree with the least cost as follows:
A: 0.2
B: 0.3
C: 0.5
In this OBST example, the search cost for this OBST will be minimized.
Also Read: Asymptotic Notation in Data Structure
Dynamic Approach to Optimal Binary Search Trees: Solving OBST Using Dynamic Programming
The dynamic approach to constructing Optimal Binary Search Trees (OBSTs) is a powerful technique that utilizes dynamic programming to find the optimal solution efficiently. Dynamic programming solves complex problems by dividing them into smaller overlapping subproblems which get calculated only once and preserved for later reuse. The “memoization” technique prevents unnecessary computations which ultimately accelerates the entire operation.
Optimal Substructure
A key concept in the OBST using dynamic programming approach is “optimal substructure.” It means that the optimal solution to a larger problem can be obtained by combining the optimal solutions of its smaller subproblems. For OBSTs, the optimal substructure property is crucial because it allows us to find the best arrangement of nodes in a subtree and then reuse this solution when constructing the overall tree.
Moreover, you can also look for Introduction to Binary Search Trees: Definition and Overview to better understand binary search trees and their properties.
OBST Using Dynamic Programming: Bottom-Up Strategy
The bottom-up dynamic programming approach begins by considering all individual nodes as subtrees of height 1 and gradually builds the optimal solution for larger subtrees. It constructs a table to store the costs of optimal subtrees. This table is then utilized to build the final optimal binary search tree.
Complexity Analysis of Optimal Binary Search Tree: OBST Time Complexity and Space Complexity
The dynamic programming approach allows us to construct an OBST efficiently. The time complexity of optimal binary search tree is O(n^3), where n is the number of nodes.
The space complexity of constructing an OBST is O(n^2).
To reduce the OBST time complexity, Knuth’s optimisation can be used. It can be improved to O(n^2).
Knuth’s Optimisation leverages the monotonicity property of the OBST recurrence to reduce unnecessary computations and reduced the time complexity of optimal binary search tree.
Also Read: Bellman–Ford Algorithm – A Complete Guide
Different Approaches to Optimal Binary Search Tree Algorithm
Below are the different approaches optimal binary search tree:
- Greedy Approach: The greedy approach constructs an OBST by making locally optimal choices at each step. However, it may only sometimes result in the overall optimal solution.
- Weighted Median Approach: This approach selects the root based on the weighted median of the elements, aiming to balance the subtrees’ costs.
- Huffman Coding: Huffman coding, a data compression technique, can be adapted to construct an optimal binary search tree when probabilities are used as frequencies.
Advantages of Optimal Binary Search Tree
Below are the advantages of optimal binary searches tree:
- Fast Search Operations: An OBST ensures that frequently accessed items are closer to the root, leading to faster search operations.
- Efficient Data Retrieval: By minimizing search costs, an OBST enhances the efficiency of data retrieval in various applications.
- Space Optimization: Compared to other tree structures, OBSTs often require less memory while maintaining efficient search capabilities.
Optimal Binary Search Tree in DAA
The optimal binary search tree plays a significant role in Design and Analysis of Algorithms (DAA) by providing an efficient way to structure search operations in cases where search probabilities are known and non-uniform. It is a classic example of dynamic programming, where subproblems are solved optimally to construct an overall optimal solution. It helps in solving real-world problems that require optimized searching.
The OBST plays a crucial role in algorithm design for structured searching by ensuring:
- Minimised search time
- Efficient use of resources
- Optimal substructure property
- Reduces worst-case scenarios
Here are some of the real-world application of optimal binary search tree in DAA
Compiler Design
- Used in symbol tables to optimise identifier lookups.
- Frequently used variables and functions are placed in an optimal structure.
Database Indexing
- Database queries often have non-uniform access frequencies.
- OBST helps optimize search cost by placing frequently accessed records at the top.
Huffman Encoding
- Similar to Huffman trees, OBST minimises the cost of lookup for symbols with varying probabilities.
Spell Checkers and Auto-Completion
- In text processing and search engines, words are not equally probable.
- OBST improves lookup efficiency for frequently searched terms.
Conclusion
An Optimal Binary Search Tree is a specialized binary search tree designed to minimize search costs, leading to faster data retrieval. By employing a dynamic programming approach, we can efficiently construct an OBST. Although other approaches exist, dynamic programming is preferred due to its optimal substructure property.
OBSTs find applications in various fields where efficient data retrieval is crucial, such as databases, language processing, and network routing. If you want to know more about the OBST, algorithm design, and dynamic programming, you can choose Certificate Program in Full Stack Development with Specialization for Web and Mobile by Hero Vired. This course will help you to master full-stack development tools & technologies and grab some better opportunities in this technology driven world.
FAQs
An Optimal Binary Search Tree is a variant of binary search trees where the arrangement of nodes is strategically optimized to minimize the cost of searches, making data retrieval more efficient.
The algorithm for constructing an Optimal Binary Search Tree involves a dynamic programming approach based on the principle of "optimal substructure."
OBST using dynamic programming allows us to efficiently construct an OBST by breaking the problem into smaller overlapping subproblems, resulting in optimal solutions.
An Optimal Binary Search Tree optimality is achieved by minimizing the search costs through strategic arrangement of nodes, placing frequently accessed items closer to the root.
Updated on February 17, 2025