It is widely known that modern businesses increasingly use data and business analytics to make smarter, better-informed decisions. The organisation finds trends and patterns within large datasets, making it easier for leaders to enhance performance and achieve better outcomes. Indeed, decisions made on data are more likely to be successful. Thus, a descriptive analysis of data is necessary. So, let’s dig down to the core of descriptive analysis and understand how it works.
Understanding Descriptive Analysis
Descriptive analysis transforms raw data into meaningful information. These data offer insights into historical performance and trends. Comprehensively representing it using statistical tools and visualisation techniques that answer the crucial question: “What happened ?”
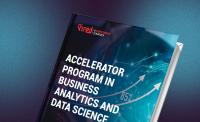
Get curriculum highlights, career paths, industry insights and accelerate your data science journey.
Download brochure
Key Features of Descriptive Analysis
Descriptive Analysis represents data mining and aggregation processes that help businesses extract actionable insights. An organisation can summarise its operations using metrics such as averages, trends, and frequencies. Many tools include dashboards, reports, and visualisations like charts and graphs that help understand patterns at a glance.
For instance, companies use descriptive analysis to analyse monthly revenue, peak sales periods, or customer demographics. These insights help set benchmarks and prepare for advanced analysis stages, such as predictive and prescriptive analytics.
Techniques in Descriptive Analysis
The core of descriptive analysis is in statistical measures that include:
- Mean, Median, and Mode: Simplify datasets to central values.
- Standard Deviation and Variance: Indicates dispersion of data.
- Trend Analysis: Identifies patterns or trends over time.
Tableau, Microsoft Power BI, and QlikView are visualisation tools that transform raw data into understandable, easy-to-interpret formats.
Applications of Descriptive Analysis Across Industries
Descriptive analysis has become a key driver across various industries, offering tailored solutions to complex problems:
- Healthcare: Hospitals analyse patient data, monitor admission rates, and track treatment outcomes to optimise resource allocation. The use of data in healthcare has improved operational efficiency while enhancing patient care.
- Retail: Descriptive analysis helps businesses monitor sales trends and inventory levels. Retailers can identify seasonal purchasing patterns by analysing historical sales data and stocking their inventory appropriately.
- Finance: Financial institutions use descriptive analysis in finance to review transaction history, detect fraud, and comply with regulations. It also lets banks personalise their product offerings by summarising customer spending behaviour.
- E-commerce: Online platforms use descriptive analysis to monitor website traffic, cart abandonment rates, and conversion metrics. These enable businesses to understand their customer journeys and refine their strategies.
- Education: Universities use descriptive analysis to assess student performance and maximise learning outcomes. Curriculum creation is informed by aggregated attendance, grade, and course feedback data.
Role of Technology in Descriptive Analysis
Procedures for Descriptive Analysis
- Data Collection
The first part of the descriptive analysis process is data collection. This entails compiling statistics from various sources, such as databases, spreadsheets, web services, and sensors. The acquired data must be accurate and complete because it is the foundation for all further studies. Proper data gathering guarantees accurate, pertinent, and adequate data for the intended analysis.
- Data Cleansing
Data cleansing is necessary to ensure the accuracy and consistency of the statistics. It includes error detection and correction, missing values management, duplication removal, and information codec standardisation. This technique frequently uses tools such as OpenRefine, Trifacta, and Python applications like Pandas to gain data cleaning. Smooth statistics are the backbone of accurate analysis because errors and inconsistencies can significantly affect findings.
- Data Integration
This process incorporates multiple statistics into one united and comprehensive dataset. Data integration combines statistics from various sources, which can be a combination of data shape alignment, desk becoming a member of, or merging datasets. Data integration generally uses ETL solutions like Talend, Informatica, or Apache Nifi. When integrated well, the information has coherence and is structured appropriately for analysis, giving comprehensive insight into the topic.
- Data Transformation
Data transformation into appropriate form and structure or transformation is generally called fact transformation. This would mean aggregation, normalisation, and clean computed fields may come forth. The facts should be well-normalised to provide uniformity in appearance; transformations ensure such consistency within the facts. Apart from Python and R, ETL technology is commonly applied to operations to transform data information.
- Data Analytics
Data exploration is examining information for the first time to determine its prime strengths and potential areas to study further. This method is used to discover styles, trends, and anomalies using succinct information, visualisations, and EDA tools. Analysts can formulate hypotheses to be studied further and gain insights from exploring statistics.
- Information Profiling
Information profiling includes the evaluation of first-rate and organisation of facts. The process helps one understand kinds of facts, their distributions, completeness, and variable connections. Profiling technologies like Informatica Data Quality, Talend, and SQL queries are utilised to create metadata in the records. It is imperative to realise these capabilities before beginning a more thorough examination to ensure the great and dependability of the information.
- Recognition of Patterns
Sample identity aims to identify patterns or regularities in the records. Examples include seasonal trends, correlations among variables, and other crucial linkages. These patterns are located using techniques such as time collection evaluation, mining association regulations, and clustering. Finding patterns in the facts is vital to comprehending the underlying causes and assisting with selection-making based on beyond tendencies.
Advantages of Descriptive Analysis
- Better Decision-Making: Descriptive analysis offers a clear picture of historical trends rather than intuitive decision-making.
- Process Efficiency: With a better understanding of inefficiencies in processes, businesses can streamline their workflows.
- Strategic Planning: Historical data assists organisations in planning for the future by providing realistic benchmarks and goals.
Problems and Limitations
While descriptive analysis offers rich benefits, it also has constraints. Since it relies on information from the past and the present data, it cannot predict future trends or strategise actionable steps.
Descriptive vs. Predictive vs. Prescriptive Analytics
Descriptive analysis offers lucrative benefits but, at the same time, is subject to constraints. Since it depends on past and present data, it does not predict future trends, strategies or actionable steps. It’s the organisations that bridge this descriptive analysis with predictive and prescriptive analytics to create such insights.
|
Descriptive Analysis
|
Predictive Analysis
|
Prescriptive Analysis
|
Summary |
What happened? |
What’s going to happen? |
What should happen? |
Function |
It uses data mining and aggregation to discover historical data. |
It looks at historical data and analyses past data trends to predict what could happen. |
It takes conclusions from descriptive and predictive analysis and recommends the best future action. |
Pros |
It’s easy to employ in daily operations. Little experience is needed. |
It’s a valuable forecasting tool. |
It offers crucial insights into making the most informed decisions. |
Cons |
It offers a limited view and doesn’t go beyond the data’s surface. |
It needs lots of historical data to work. It will never be 100% accurate. |
The main cons of prescriptive analytics are that it requires a high cost of setup. |
Conclusion: Unlocking Your Potential through Descriptive Analysis
The foundation of any data-driven strategy is descriptive analysis, which provides lucid and useful insights into historical and contemporary trends. It spans sectors including healthcare and retail, where unstructured data is transformed into insightful knowledge that can help with better decision-making.
However, it doesn’t predict future trends or prescribe specific actions. Instead, it creates an opportunity for advanced analytics, such as predictive and prescriptive models, to guide organisations to new heights of success.
As the world becomes more data-dependent and needs to solve complex challenges, professionals must master descriptive analysis to lead in this data-driven era. Hero Vired’s Certification Program in Data Analytics, offered in collaboration with Microsoft, will prepare you for influential roles in various industries by providing the fundamental knowledge and real-world experience you need to manage the constantly changing analytics landscape.
FAQs
A descriptive statistic shows the percentages of various age groups in a population or the ratio of men to women in a sample. The modest average is yet another often-used descriptive metric.
Three primary categories of descriptive statistics exist:
- The frequency of each value is the focus of the distribution.
- The averages of the values are the subject of the central tendency.
- The degree of dispersion or variability refers to how widely distributed the numbers are.
The mean and, most likely, the standard deviation should be included at the very least when presenting statistics on a data set. This is the minimum required information to understand how your data set might be distributed. It is completely up to you how much extra information you offer.
Updated on December 23, 2024