Technology is changing the way we live and work. Advanced computer systems are now part of many industries, helping to solve complex problems and improve daily operations.
These intelligent systems are important because they can analyse large amounts of data quickly and accurately. This leads to better decisions and more efficient processes across various fields, enhancing productivity and driving innovation in our modern world.
In this blog, we will explore over 25+ applications of deep learning in different industries. From healthcare to finance, automotive to education, we will look at how deep learning is making a difference in each sector.
What is Deep Learning?
Deep learning is a subset of AI that imitates the functioning of the human brain. It involves creating a stack of algorithms to facilitate the process of dealing with vast amounts of information. This helps computers identify trends, make choices, and even comprehend language.
One of the key strengths of deep learning is its ability to improve over time. As the data expands, the ability of the system to perform tasks gets enhanced. This means deep learning is very resourceful in solving intricate problems that are complicated for conventional techniques.
In various industries, deep learning is being used to enhance operations, increase efficiency, and drive innovation. The effect of its applications can be felt across industries as it changes the mode of operations of businesses and the services provided to clients.
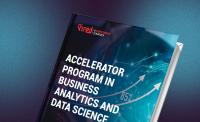
Get curriculum highlights, career paths, industry insights and accelerate your data science journey.
Download brochure
Top 25+ Applications of Deep Learning In Different Industries
It is not a surprise that deep learning is gaining popularity in many areas and it has enabled business processes and service provision to change for the better, while driving many innovations. Here, we will look at 25 applications of deep learning solutions in various sectors and appreciate how far it has come and how many industries it can change.
Deep Learning Applications in the Healthcare Industry
Medical Image Analysis
Medical image analysis is gaining massive traction due to improved accuracy, reliability and efficiency of diagnoses, which has been powered by deep learning. It enables physicians to streamline workflows, support better workflow, diagnose diseases in an earlier stage, and automate image processing tasks in addition to improving overall image interpretation.
Below are its key contributions:
- Early Disease Detection: Assists in the early detection of diseases such as cancer, pneumonia, and even neurological disorders by analysing the medical images with incredibly high precision.
- Automated Image Segmentation: Enables separation of different structures of anatomy present in medical images, allowing a radiologist to locate such areas that require focus with ease.
- Enhanced Diagnostic Accuracy: Minimises the chances of human error, thus increasing the consistency of the outcomes of the tests. This greatly improves patient care and enhances decision making regarding the treatment.
Disease Prediction
The use of patient information to forecast and develop clinical measures for effective healthcare is enabled through deep learning. The model uses risk prevention and prescription medicines for effective health management.
Here are its primary uses:
- Risk Assessment Models: This allows estimating the probability of diabetics and heart illnesses based on history and genetic information from the patient.
- Preventive Healthcare Strategies: This helps the healthcare provider to come up with strategies that will reduce the chances of disease development or advance the disease.
- Personalised Treatment Plans: This produces specific treatment strategies for patients with special needs and risk factors to promote effective medical care efforts.
Also Read: What are the Differences Between Machine Learning and Deep Learning?
Deep Learning Applications in the Finance Industry
Fraud Detection
Deep Learning is important in detecting fraud in financial transactions by uncovering suspicious activities in a huge database of transactions. This helps banks and businesses avoid incurring heavy losses and protects their institutions.
Below are its key contributions:
- Transaction Monitoring: Monitors external transactions to ensure that clients’ money is not lost through suspicious activities.
- Anomaly Detection: Identifies deviations from normal behaviour in user activities to flag potential fraud.
- False Positive Reduction: Helps eliminate incidents of false alerts by being able to differentiate normal and abnormal transactions.
Credit Scoring
Deep learning systems transform credit scoring by providing a data-oriented way of estimating a borrower’s credit risk. It examines several aspects and offers reasonable forecasts which improve lending decisions.
Below are its key contributions:
- Data Integration: Combines information from several sources such as payment records, expenditure patterns and general financial conduct to yield a single assessment.
- Improved Accuracy: Provides very precise credit scores thus increasing profitability for financial institutions.
- Inclusive Scoring: Evaluates non-traditional data, enabling credit access for individuals without extensive credit histories.
Algorithmic Trading
Deep learning improves algorithmic trading by forecasting financial markets’ moving patterns and making timely investments. This enables a more profitable trading operation with lower risks.
Below are its key contributions:
- Market Pattern Recognition: Discovers various tendencies and patterns within vast areas of financial information making it possible to use them in accurate trading.
- Risk Management: Analyses fluctuation levels within the market to enable appropriate and less risky investments.
- Automated Trading: Buys and sells automatically using the most current information decreasing the amount of human input required.
Deep Learning Applications in the Automotive Industry
Autonomous Driving
Deep learning is now essential in the development of autonomous vehicles, allowing them to integrate, plan, and safely traverse any environment. Upon evaluating multiple sensor data ranging from camera images to LiDAR data, deep learning enables autonomous vehicles to locate objects, identify traffic signals, and be aware of people. This leads to an increase in driving safety.
Below are its key contributions:
- Object Detection: Finds vehicles, people, intersections and other objects and hazards in real time.
- Traffic Sign Recognition: Recognises and understands traffic signals and signs to comply with traffic laws.
- Path Planning: Establishes route for driving while factoring out the safety threats.
Predictive Maintenance
In vehicles, predictive maintenance applies deep learning to foretell certain breakdowns of the vehicle systems and maintenance activities. This reduces expenditure on repairs and downtime by analysing data provided by vehicle sensors to determine how long the component parts are expected to last. As a result, it enables the vehicles to work in an efficient manner and also be dependable.
Below are its key contributions:
- Sensor Data Analysis: Monitors engine, brakes, and other components for signs of wear.
- Failure Predictions: Provides alerts about possible breakdowns before they occur.
- Cost Optimisation: Helps schedule repairs proactively, reducing unexpected expenses.
Also Read: Top Deep Learning Interview Questions and Answers
Deep Learning Applications in the Retail Industry
Personalised Product Recommendations
The shopping experience has been revolutionised due to advances in Deep learning techniques that recommend products with much higher accuracy. It considers customer’s preferences, history of purchases and online browsing, and recommends items based on what fits them best. Retailers utilise this to increase the level of satisfaction of customers and sales. By understanding what customers want, businesses can tailor their offerings effectively and foster loyalty.
Below are its key contributions:
- Behavior Analysis: Tracks customer interactions to identify preferences and interests.
- Dynamic Suggestions: Provides real-time recommendations during shopping sessions.
- Increased Sales: Encourages additional purchases by presenting complementary or popular items.
Inventory Management
Deep learning uses demand forecasting, waste reduction, and stock-level management for optimising inventory control. It considers past sales data, market conditions and seasonality to help retailers maintain good stock levels. This decreases both overstocking and stock-out situations and improves the efficiency of the firm’s operations.
Below are its key contributions:
- Demand Forecasting: Predicts future sales trends for effective stock planning.
- Waste Reduction: Helps minimise surplus inventory and associated losses.
- Supply Chain Optimisation: Ensures timely restocking by tracking product movement.
Deep Learning Applications in the Agriculture Industry
Crop Health Monitoring
Deep learning helps in crop production by monitoring crop health using satellite images, drones and IoT devices. Deep learning identifies the symptoms of disease, pests, or deficiencies in nutrients so that farmers can act upon them quickly. This helps in achieving better yields and decreasing losses which result in more sustainable farming processes. With the right data, farmers can concentrate on improving their crops and use fewer resources.
Below are its key contributions:
- Disease Detection: Identifies signs of disease or pest attacks early.
- Nutrient Analysis: Monitors soil and plant nutrient levels.
- Yield Protection: Helps safeguard crops from potential risks.
Precision Farming
Deep learning supports smart farming by providing effective resource management and optimisation across the various operations conducted on a farm. It allows farmers to use water fertilizers and pesticides effectively, yet yield more. With technology-enabled surveillance and foresight, smart farming reduces loss and encourages eco-friendly agricultural processes.
Below are its key contributions:
- Resource Optimisation: Guides the use of water and fertilizers for better efficiency.
- Crop Growth Prediction: Forecasts crop performance based on environmental conditions.
- Automated Equipment Control: Integrates with smart farming machines for precise actions.
Deep Learning Applications in the Energy Industry
Smart Grid Management
Deep learning revolutionises energy distribution by enabling smart grid management systems. It predicts energy demand, balances supply, and ensures efficient electricity distribution. This helps prevent power outages, reduces energy waste, and enhances grid reliability. By analysing real-time data, utilities can optimise energy flow and improve customer satisfaction.
Below are its key contributions:
- Demand Forecasting: Predicts power usage patterns to meet energy needs.
- Load Balancing: Distributes electricity efficiently across the grid.
- Fault Detection: Identifies and resolves issues in the grid quickly.
Renewable Energy Optimisation
Deep learning plays a critical role in renewable energy by improving the efficiency of solar, wind, and other green energy sources. It predicts weather conditions and optimises energy production, making renewable energy systems more reliable. This drives sustainability and reduces dependency on fossil fuels.
Below are its key contributions:
- Energy Production Forecasting: Predicts solar and wind energy output based on weather data.
- System Performance Monitoring: Ensures optimal functioning of renewable energy systems.
- Grid Integration: Balances renewable energy with traditional sources effectively.
Deep Learning Applications in the Education Industry
Personalised Learning Paths
Deep learning enables personalised learning by adapting educational content to meet individual student needs. It analyses learning patterns, strengths, and weaknesses to deliver tailored study plans. This approach enhances engagement and helps students achieve better results. By focusing on each learner’s unique requirements, personalised learning fosters more effective education.
Below are its key contributions:
- Learning Pattern Analysis: Identifies areas where students excel or struggle.
- Adaptive Content Delivery: Provides customised lessons and materials for better understanding.
- Improved Student Outcomes: Ensures students learn at their own pace effectively.
Automated Grading Systems
Deep learning simplifies the evaluation process by automating grading for assignments and exams. It provides consistent, unbiased assessments while saving educators significant time. This technology improves accuracy and allows teachers to focus more on instruction rather than manual tasks.
Below are its key contributions:
- Speed and Efficiency: Grades assignments quickly, even for large classes.
- Bias-Free Evaluation: Ensures fair and objective grading for all students.
- Error Reduction: Minimises the chances of human mistakes in evaluations.
Student Performance Prediction
Deep learning helps predict student performance by analysing historical academic data, attendance, and engagement levels. It identifies at-risk students early, enabling educators to provide necessary support and interventions. This proactive approach promotes student success and reduces dropout rates.
Below are its key contributions:
- Risk Identification: Flags students who may struggle academically.
- Proactive Support: Guides timely interventions to address performance gaps.
- Data-Driven Insights: Provides actionable feedback for teachers and administrators.
Deep Learning Applications in the Transportation and Logistics Industry
Route Optimisation
Deep learning transforms transportation by enabling intelligent route optimisation. It calculates the most efficient paths for deliveries, reducing travel time and costs. By analysing traffic patterns, weather conditions, and delivery schedules, this technology ensures smooth logistics operations.
Below are its key contributions:
- Traffic Prediction: Anticipates congestion for better route planning.
- Fuel Efficiency: Reduces fuel consumption by optimising travel distances.
- Delivery Time Reduction: Ensures faster deliveries and improved customer satisfaction.
Demand Forecasting
Deep learning improves logistics by accurately predicting demand for goods and transportation services. It analyses historical data, market trends, and seasonality to help businesses allocate resources efficiently. This minimises operational costs and ensures the timely fulfilment of customer needs.
Below are its key contributions:
- Resource Planning: Optimises the deployment of vehicles and staff.
- Inventory Management: Aligns stock levels with predicted demand.
- Cost Savings: Reduces unnecessary expenses through precise forecasting.
Deep Learning Applications in the Telecommunications Industry
Network Optimisation
In terms of connectivity as well as network performance, deep learning aids in optimising networks by studying data trends over time. It forecasts outages, suggests changes to avoid interruptions, and more. This guarantees dependable services and content clients.
Below are its key contributions:
- Traffic Management: Balances network load to prevent congestion during peak hours.
- Fault Prediction: Identifies potential disruptions before they affect users.
- Performance Enhancement: Recommends configurations for higher speed and stability.
Customer Service Automation
Deep learning provides the possibility to provide seamless services by automating customer service processes through systems like chatbots and virtual assistants. These service systems quickly respond to frequently asked questions, thus lessening wait time for customers.
Below are its key contributions:
- 24/7 Availability: Ensures round-the-clock support for customer inquiries.
- Quick Query Resolution: Handles routine tasks without human intervention.
- Personalised Interactions: Provides responses tailored to individual customer needs.
Deep Learning Applications in the Security Industry
Intelligent Surveillance Systems
Deep learning enhances the surveillance of security by running automated systems that provide supervision and review of video clips. It detects unusual activities, unauthorised access, and potential threats, enabling quick responses. This enhances public and private safety measures.
Below are its key contributions:
- Real-Time Monitoring: Monitors live streams to detect and verify suspicious actions.
- Face Recognition: Recognises a person or group of people for whether they have the right to enter.
- Anomaly Detection: Reports processes or actions which are unusual.
Threat Detection
Deep learning improves threat detection by analysing cybersecurity data for vulnerabilities and malicious activities. It protects systems from cyberattacks and helps businesses maintain data integrity. This is crucial in preventing financial and reputational losses.
Below are its key contributions:
- Intrusion Detection: Logs all attempts to access the computer network against the set policies.
- Malware Analysis: It is the process that determines the malicious software.
- Proactive Defense: It is a method that assumes further possible threats and as such builds additional security provisions.
Deep Learning Applications in the Real Estate Industry
Property Valuation Models
Deep learning enhances property valuation by analysing various factors like location, market trends, property features, and historical data. It provides accurate and real-time estimates, enabling buyers, sellers, and investors to make informed decisions. This improves the efficiency of real estate transactions and ensures fair pricing.
Below are its key contributions:
- Market Trend Analysis: Tracks price fluctuations and demand patterns in specific areas.
- Feature-Based Valuation: Considers property characteristics, such as size and amenities, for precise pricing.
- Real-Time Updates: Adjusts property values based on changing market conditions.
Virtual Property Tours
Virtual property tours, including homes and commercial spaces, are made easier through deep learning, which helps to create 3D models of these properties and enable computerised walkthroughs. The innovation enhances the experience of the buyer as they have the opportunity to visualise the property without physically visiting it. This is beneficial to both buyers and sellers while increasing the exposure of listings.
Below are its key contributions:
- Immersive Visualisation: Creates detailed, interactive 3D models of properties.
- Remote Accessibility: Allows potential buyers to explore properties from anywhere.
- Enhanced Decision-Making: Helps buyers make confident choices without physical visits.
Deep Learning Applications in the Marketing Industry
Campaign Optimisation
Deep learning redesigns marketing campaigns by utilising the existing customer data and performing a series of functions that enable one to predict how well the campaign will fare. This way the campaign is able to point out the best strategies, audiences and the best channels to use in order to get the best returns. These insights may be utilised by marketers to optimise their efforts and achieve better outcomes.
Below are its key contributions:
- Audience Segmentation: Identifies and categorises customers based on behaviour and preferences.
- Performance Prediction: Forecasts campaign success based on historical data.
- Resource Allocation: Optimises budgets and resources for better impact.
Sentiment Analysis
Deep learning enables sentiment analysis by understanding customer opinions through reviews, social media posts, and feedback. It helps businesses gauge public perception of their brand, products, or services, allowing them to adapt strategies accordingly.
Below are its key contributions:
- Opinion Mining: Extracts customer sentiments from large datasets.
- Real-Time Insights: Provides immediate feedback on campaigns or product launches.
- Brand Perception Management: Guides businesses to address negative feedback effectively.
Conclusion
Deep learning is at the forefront of modernisation as it develops new ideas and improves efficiency in various sectors. Its use cases include but are not limited to, the medical, financial, and automotive sectors, hinting at its power to turn things around. With its strength, businesses are increasing their level of precision, lowering operational expenses, and moving towards better judgment.
As technology evolves, deep learning will open new opportunities across different areas. Its flexibility and possibility for further advancement confirm it as an important element of progress and industry development. Get more information on deep learning with the Integrated Program in Data Science, Artificial Intelligence & Machine Learning in collaboration with MIT Open Learning by Hero Vired and get professional guidance and certification.
FAQs
Deep learning is used for medical image analysis, disease prediction, and personalised treatment plans to enhance patient care.
It is used for fraud detection, credit scoring, and algorithmic trading to improve accuracy and reduce risks.
Deep learning optimises routes, forecasts demand, and powers autonomous vehicles for safer and more efficient transportation.
Of course, it allows for the use of chatbots and virtual aids to enable customers to have fully automated, personalised support 24 hours a day.
Deep learning enables marketers to better adjust their campaigns according to multiple parameters including client segmentation, sentiment analysis and even social media leverage to improve the outreach to their audiences.
Deep learning applications are widely used in industries such as healthcare, finance, retail, automobile industry, educational sectors and agriculture among others.
Updated on December 26, 2024