Today, data and analysis are becoming very important in decision-making processes. Companies have started utilising the data to find business trends from the data and improve their business strategies. However, using the right tools can simplify and enhance data analysis. These make data cleaning, charting and performing statistical analysis easier.
When there are many tools available in the market, choosing the appropriate one becomes a daunting task. Every tool has its strengths, which are designed to serve its purpose, from subsequent data gathering to drawing conclusions.
In this blog, we will discuss the 25 best tools of data analytics that assist in gathering and analysing data. Their categories include data cleansers, data visualisation, data analytics, machine learning, etc. Afterwards, you will learn to choose the tools that will suit your needs the best.
What is Data Analytics?
Data analytics encompasses the defining or management of the datasets to derive relationships, patterns, trends or other useful insights. This assists businesses to make informed decisions, discover new possibilities and streamline their operations. Companies are required to perform such analysis in order to make their business practices more responsive to the market as well as the customers’ needs.
Analytics tools support this process by simplifying tasks like cleaning, drawing charts or graphs, and performing statistical operations. Such tools would enable people to do such analysis faster and more accurately, which is particularly crucial for comprehending complicated information. Generally, data analytics has established its role as an integral aspect for organisations wanting to be competitive in today’s era, which has been dominated by massive amounts of data.
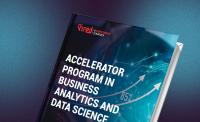
Get curriculum highlights, career paths, industry insights and accelerate your data science journey.
Download brochure
Data cleaning and preparation tools are used for organising, cleaning and transforming the data for analysis. Such tools also assist in improving the data quality, hence increasing the reliability and efficiency of analysis.
Alteryx
Alteryx is an advanced tool that assists in automatically preparing, blending and analysing the data. Most data analysts use the drag-and-drop workflow, which is easy to master, and this allows bulk data cleaning and transformation without complex encoding.
Key Features
- Workflow Automation: Automated workflow so that an individual does not have to repeat certain data preparation efforts.
- Data Blending: Linking Up to include data from different sources easily.
- Integration: Being able to connect to lots of different platforms and data sources.
- Analytics Tools: Inbuilt predictive and spatial analytics capabilities.
OpenRefine
OpenRefine, previously known as Google Refine, is a very useful open-source software for cleaning up messy data. It is available for free and highly praised by specialists as a quick way to analyse and reshape datasets, including large ones.
Key Features
- Data Exploration: This feature allows fast data browsing and basic filtering
- Undo and Redo: In the operation of the system, every single action is recorded and can be rolled back in a moment.
- Data Transformation: Offers a range of functions for transforming data formats.
- Faceting and Clustering: These automated functions employ the same logic as sorting and averaging and allow system users to quickly and simply merge closely related entries.
Trifacta
Trifacta’s primary purpose is to allow users to wrangle and prepare raw data. Trifacta is designed to be used by data professionals, as it incorporates easy-to-use functions for preparing data for analytics and machine learning.
Key Features
- Visual Interface: Allows users to perform data cleaning tasks with graphics assistance.
- Data Wrangling: Provides tools for shaping data in various formats.
- Team Project: Facilitate staff collaboration on data projects.
- Machine Learning Model: Helps users to suggest transformations and recognize patterns using machine learning.
Using visualisation tools makes it easier to interpret and present data, such as tables and maps. These tools enable easy tracking of changes over time and efficiency in creating informative reports.
Tableau
Among the best tools for data presentation and visualisation, which can create beautiful dashboards with the possibility of interactivity, is Tableau. Due to its practicality and powerful analytic capabilities, it is used in the business sector for large volumes of data. It has become the best and most widely used data visualisation tool in industries.
Key Features
- Interactive Dashboards: Allows users to drill down into data for detailed insights.
- Wide Data Integration: Has wide integration capabilities with other tools, including Excel, SQL, and cloud sources.
- Real-Time Analysis: Provides real-time data updates for live dashboards
- Drag-and-Drop Interface: Enables the creation of visualisation without coding requirements.
Power BI
Power BI, as developed by Microsoft, enhances data presentation and integration with other Microsoft products. The solution allows users to develop interactive reports and dashboards, which is a plus for companies that work in the Microsoft ecosystem.
Key Features
- Seamless Microsoft Integration: Office 365, Azure and SQL Server integration is seamless and easy.
- Custom Visuals: Offers a marketplace for additional visual customization options.
- Data Automation: Automatically updates visuals with refreshed data.
- Interactive Visualization: Enables users to develop graphics within a few minutes using drag-and-drop functionality.
Google Data Studio
Google Data Studio is a data visualisation application that is free of charge and is ideal for users with Google-based data sources such as Google Analytics, Google Ads and BigQuery. It allows the creation of customizable dashboards, making it easier for users in data handling and presentation.
Key Features
- No Charge: Free of charge, suitable for small businesses and individual users.
- Google Services: Easily integrates with other Google services and data.
- Teamwork: Facilitates sharing and working with other members of the team.
- Easily Editable Templates: Has ready-made templates to ease report writing.
Qlik Sense
Qlik Sense is recognized for its distinctive associative data engine, which allows users to navigate through data and find relationships that other tools would overlook. It’s Favoured for its intuitive, self-service features of visualising the data.
Key Features
- Associative data engine: This feature enables users to search and connect the dots between multiple datasets.
- Data Literacy Tools: This gives a user several processes involved in understanding data better.
- Data self-service: This lets people discover and accept insights without assistance.
- Accessibility: Performs well on both mobile devices and desktop computers.
Looker
Looking at Looker, firstly, it is a data analysis platform that is part of Google and the key focus is data exploration, which is done in the cloud. It pulls live databases from the database to allow a user to see predicted attributes and also interact with the data during presentation.
Key Features
- Real-Time Data Exploration: Live Monitoring Monitoring Insight with Information from Connected Databases.
- Interactive Charting: Presents a number of charts & graphs which can be personalised.
- SQL-Based Queries: Enables advanced data querying for detailed insights.
- Google Cloud Integration: Integrates with Google’s cloud services for seamless data handling.
Statistical analysis tools help users in performing complex calculations, as well as data analysis and forecasting. These are common tools used by researchers, analysts, and data scientists, dealing with statistical data.
R
R is a programming language that is a free and open-source software application for statistical computing and graphics. The language is flexible and is reported to have a strong inclination towards offering a hefty number of packages for statistical analysis, thus its popularity amongst data scientists and researchers on the top.
Key Features
- Extensive Package Library: Provides numerous packages for various statistical methods.
- Graphics: Offered with strong graphics and data visualisation functions.
- No Cost: Free to use and has a large, active community.
- Made Available For Various Systems: Windows, Mac OS, and Linux are all supported.
SAS
SAS (Statistical Analysis System) is one of the most used and trusted software in the field of advanced statistical analysis, which is mostly employed in the business and research realms. The software is very popular as it is engineered with excellent features, including data information, predictive analysis and machine learning, along with the capability to work with large datasets.
Key Features
- Robust Data Handling: Handles massive datasets without breaking a sweat.
- Advanced Analytics: Offers features for predictive and prescriptive analytics.
- High Security: Marketed with safe and reliable data handling.
- Industry Standard: The healthcare industry, finance sector and government, among other industries, commonly use this.
SPSS
SPSS is a software package used for mid-range computer statistical analyses. It is popularly known due to its simplicity and offering an extensive number of statistical functions.
Key Features
- Easy-to-use Interface: It offers a straightforward, menu-driven approach to analysis.
- Comprehensive Analysis: Capable of conducting more advanced statistical tests or analyses than other types of studies.
- Data Management: The software contains built-in solutions for cleaning and restructuring datasets.
- Visualisation Options: Allows users to create charts and graphs for easy interpretation.
Excel
This is another application developed by Microsoft used for simple statistical operations and computing more than one variable. Unlike others, excel is integrated into many computers and has great potential for computation, display, and even statistical calculations.
Key Features
- Widely Available: This is part of the Microsoft Office package, so more people will have access to it.
- Basic Statistical Functions: Has common statistical analytic functionalities and calculations.
- Data Visualization: This tool provides a wide range of different chart types to help display information.
- Add-Ins Support: Allows additional functionalities through plugins like Analysis ToolPak.
MATLAB
MATLAB is a high-level language and interactive environment that is primarily designed for technical computation and mathematical modelling. Its capabilities of statistical and graphical representations of data make it useful across a number of fields, such as engineering, physics, and finance.
Key Features
- Matrix-Based Language: Best suited for high-order algebra and statistical problems.
- Data Visualization: Provides extensive options for plotting and graphing data.
- Customizable Scripts: It is possible for users to customise scripts for certain analyses.
- Toolboxes: It also provides tool boxes specifically on machine learning and data analysis.
Machine learning and predictive analytics tools have been developed with the objective of aiding companies to develop models based on historical data that will help in predicting future events. They help in automating processes or transforming data and information into intelligence with the ability to forecast certain events in the future.
RapidMiner
RapidMiner is a data science platform that encompasses end-to-end data preparation, machine learning and model deployment. It’s characterised by a drag-and-drop feature on its user interface that facilitates machine learning model building for those who are not programmers.
Key Features
- No-Code Environment: Mendeley enables users to create models without coding and with the help of a visual interface.
- End-to-End Workflow: Encompasses all processes, including data and model generation.
- Algorithm Variety: Contains many types of algorithms meant for classification, regression and clustering.
- Collaborative Features: Provides for easy interaction of members in teams during the course of the assignment.
DataRobot
DataRobot is a machine learning platform that automates the model-building process, making it easier for users to create accurate predictions. It is motioned to accelerate the workflow involved with data scientists and analysts while providing automated insights that are effortless yet accurate.
Key Features
- Automated Machine Learning: All stages of building a machine learning model, including tuning, are fully automated.
- Model Interpretability: Provides tools to explain model predictions.
- Deployment Flexibility: This can be deployed on distributed cloud or on-premise and even hybrid systems.
- Built-in AI Accuracy: The assertions made on the predictions are further enhanced by AI techniques.
KNIME
KNIME, or Konstanz Information Miner in full, is a free and open-source software that is self-proclaimed for capabilities in data analytics and visual workflows. It is widely used by data scientists and other analysts for the processes of construction, fitness testing, as well as the deployment of ML algorithms.
Key Features
- Visual Workflow: Workflows can be built by the users in order to facilitate easy upload and completion via the use of nodes.
- Open Source: Easy to use and has a very active support base.
- Integration with R and Python: There is an extended support for R and Python, which are coded into the platform.
- Data Blending: The users get the benefit of merging and integrating data from several sources, thus increasing the depth of the analysis.
TensorFlow
TensorFlow is an open-source machine learning framework that was developed by the tech giant Google. This software is ideal when it comes to making intricate, deep learning systems, and this is paramount in sectors such as image recognition, natural language processing or time series analysis.
Key Features
- Deep Learning Capabilities: Known for its support of deep neural networks.
- Scalable: It is capable of being employed in large-scale ventures in diverse environments.
- Cross-Platform Support: This can be operated in different operating systems and different devices.
- Community and Resources: A robust developer community also supports this tool, along with resourceful instructions.
Big data tools are software solutions used to store and manipulate a large batch of data in an efficient and fast manner. Big data tools help businesses in utilising large data sets and transparency towards their decision-making that helps achieve their strategies and objectives.
Apache Spark
Spark is an open-source big data processing framework with an extended processing speed because of its in-memory computing capabilities. It is commonly used for both batch and real-time analysis. Therefore, data engineers and data scientists find It useful.
Key Features
- High-Speed Processing: It processes data faster by incorporating in-memory computing.
- Real-Time Data Handling: It can manage both batch and real-time streaming data.
- Compatibility: Integrates seamlessly with Hadoop or other big data platforms.
- Multiple Language Support: Enables developers to build in Python, Java, Scala, and R.
Cloudera
Cloudera is a modern platform designed for data management and analytics incorporating data processing technologies, machine learning, and large-scale data analytics. Cloudera is recognized for its scalability, and it is, therefore, applied in enterprise settings to filter and analyse huge datasets.
Key Features
- Enterprise-Level Solution: The platform is secure and highly scalable for large data.
- Hybrid Cloud Support: It has both on-premise, cloud and hybrid deployments.
- Data Security and Governance: Adheres to the enterprise’s standards for data security.
- Machine Learning Integration: Integrates machine learning and predictive analytics.
Snowflake
Snowflake is defined as a contemporary cloud data warehouse for businesses that can be used to load and analyse large datasets efficiently. Snowflake is a flexible cloud data warehousing with a lot of storage space, so it’s regularly used to store massive data that is more efficient to analyse later on.
Key Features
- Cloud-Native: Completely hosted in the cloud, with no hardware or software to support.
- Scalability: Allows dynamic scaling to handle fluctuating workloads.
- Data Sharing: Cross-cloud data sharing with multiple technologies and infrastructure securely.
- High Performance: Enhanced query input-output with efficient storage and faster data retrieval.
Some of the essential tools for storing, structuring, and administering huge amounts of data are database management and data warehousing tools. These tools make sure the data can be analysed and decisions made based on it consistently and securely.
MySQL
MySQL is a widely used open-source relational database management system. Known for its reliability and ease of use, MySQL is popular in both small businesses and large enterprises for managing structured data.
Key Features
- Open-Source: Use free of charge without a licence and provided with good support from the community.
- High Performance: Has high efficiency in performing operations on large datasets and executing queries promptly.
- Cross-Platform: Available on various operating systems.
- Data protection: It has thorough capabilities with respect to protecting data, including access control at the user level.
Amazon Redshift
Amazon Redshift is a relational database management system implemented as a web service through the Amazon Web Services (AWS) platform. As a result, it stands out among big data and business intelligence technologies because of its capacity to quickly analyse massive amounts of information.
Key Features
- Fast Query Processing: Addressed to large data sets and to complex analytical queries.
- Scalable Architecture: Supports the growth of storage and compute resources as required.
- Integration with AWS: The whole process of moving, transforming, and analysing data can be done within the AWS environment.
- Data Encryption: Advanced features such as data encryption assist in better protection.
Google BigQuery
Google BigQuery is a fully managed, serverless data warehouse that allows users to analyse massive datasets using SQL. The power of BigQuery is its speed and vastness with good scalability and therefore is becoming the go-to tool for businesses that require quick and real-time analytics in a cloud environment.
Key Features
- Serverless Management: Takes away the pain of handling infrastructure and only directs the focus on data analysis.
- Scalable and Fast: Processes huge amounts of data in just a few seconds.
- Machine Learning Integration: Allows the users to build machine learning models right in BigQuery.
- Real-Time Analytics: Capable of providing real-time analysis with live data streaming.
Data integration tools help in the migration of data to a single system from various systems, enabling credible data transfer across functions. These tools are essential for businesses that need a unified view of data to make informed decisions.
Informatica
Informatica is a premier data integration tool that brings impressive ETL strength. It assists you in the overlap of data sets derived from different sources, assuring data for analysis and business intelligence remains strong and trustworthy.
Key Features
- Powerful ETL Capabilities: Data from numerous sources can be extracted, transformed and loaded quickly and smoothly.
- Data Quality Management: Solutions are provided to improve the reliability and validity of data.
- Scalable Solution: Effective in managing a great amount of data, it is applicable for companies.
- Cloud and On-Premise Support: Provides deployment in both cloud as well as on-premises environments to enhance flexibility.
Apache NiFi
Apache NiFi is an open-source data integration tool that automates the movement of data between systems. Its usage is acknowledged widely due to its easy Interface and its capability to perform data operations on moving streams, enabling the efficiency of data integration.
Key Features
- Visual Data Flow Management: It enables users to use a drag-and-drop interface to construct data flows.
- Real-Time Data Processing: It allows for the transfer and transformation of data in real-time.
- Built-in Security: It provides security features such as SSL, encryption, and access control.
- Scalability: It is appropriate for complicated data environments as it is capable of large-scale data processing.
Choosing a data analytics tool will strengthen or undermine your efforts at data analysis. Use this step-by-step guide to pinpoint the best tool for your particular case and needs:
1. Understand Your Business Requirements
- Formulate the objectives you would like to achieve using analytic techniques.
- Formulate into what particular forms of the data you are going to work on – structured or unstructured.
- Identify the use cases, for example, how the data is intended to be used, e.g., for visualisation, statistical analysis, machine learning models, etc.
2. Assess Tool Features
- Search for tools that meet your needs, for example, data cleaning, visualisation, or forecasting.
- Make sure that the tool has the necessary integrations, such as databases or cloud services.
- Check for automation capabilities to save time on repetitive tasks.
3. Evaluate Ease of Use
- Consider whether the tool is user-friendly for your team.
- Look for drag-and-drop interfaces or other features that simplify tasks.
- If the tool requires coding, ensure your team has the necessary skills.
4. Check Scalability
- Assess whether the tool can handle increasing data volumes as your business grows.
- Choose a solution that supports future expansion and additional features.
5. Compare Costs
- Analyse the total cost of ownership, including subscription, training, and implementation costs.
- Find out if a free trial or an open-source option is a good substitute for you.
6. Consider Security and Compliance
- Find out if the tool meets relevant laws and regulations, such as the GDPR.
- Make sure that the tool has adequate security features such as data encryption, access control, and user authentication goods.
7. Read Reviews and Seek Recommendations
- Read reviews and get testimonials from users in the same field as you are in.
- Ask your colleagues to hear about their experiences with certain tools.
8. Test Before Committing
- Take advantage of free trials or any available demos to try out the tool with your data.
- Watch out for its performance, speed, or user experience before you make that choice.
If you follow these steps, you will be able to select suitable data analytics tools that fulfil your business requirements and improve your data analytics capability and decision-making in your organisation.
Conclusion
The importance of data analytics cannot be understated, especially when it comes to making tough decisions that require effective evaluation of extensive data. From data cleansing to modelling, these tools allow organisations to optimise their strategies, leading to improved results. Selecting a tool that is appropriate for the job implies that the time and resources used are properly utilised and aligned with your objectives.
In this blog, 25 essential tools across various categories, such as visualisation, machine learning, big data and other categories, were reviewed. Familiarising yourself with these features will ensure that you choose the most useful tools that fit your company’s requirements and finally start deriving useful business information from your data. To learn more about Data Analytics, you must opt for the Certification Program in Data Analytics With Microsoft by Hero Vired, which provides all the information that you may require to become a professional in this field.
FAQs
Data analytics tools do the data cleaning and processing followed by analysis to reveal patterns that are useful in decision-making.
Tableau, Power BI, and Google Data Studio are good tools for interactive data visualisation.
Data integration tools are those that connect different types of data sources, cleaning tools are those that organise and prepare raw data for analysis.
Yes, the tools are R, Apache NiFi, and KNIME are open-source and are tools used for data analytics.
The businesses’ needs, the functionality of the tools, how scalable the tools are, the usability of the tools and the costs involved in obtaining the tools are some of the factors to consider while choosing the right tool.
Updated on November 19, 2024