Companies churn out large volumes of data each year. A current source proposed nearly 2.5 quintillion bytes of data being generated daily. Managing such colossal amounts of data can be a pain point for your business. Here’s where the classification of data mining becomes functional.
If you are keen on applying for a Business Analytics and Data Science course, sufficient knowledge about data mining can help dramatically. Learn more below.
What is Classification of Data Mining?
Classification of data mining systems is the process of segregating data points into distinctive classes. It eases the organization of data sets of diverse sorts. So, whether you have small & simple databases or large & complex ones, this process can provide a seamless classification of all.
Also read about: Data Warehouse
Types of Classification f Data Mining
Below are the key types of data mining classifications:
Supervised Classification
The type of data mining classification fits when you are on the lookout for a specific target value to make classifications and predictions in data mining. The targets can generate one or two results pertaining to the possibilities.
Unsupervised Classification
Here there is no concentration drawn to predetermined attributes. It also has very few things to do with the target value. It is only used to map out hidden relations and data structures.
Semi-Supervised Classification
This classification of data mining is the middle ground between the supervised and unsupervised classification of data mining. Here, the fusion of unlabeled and labelled datasets becomes functional at the time of the training period.
Reinforcement Classification
This data mining classification type involves trial and error to determine ways to best react to situations. It also allows the software agent to understand its behaviour depending on the environmental reviews.
What are the Classification Techniques in Data Mining?
Below are the major classification techniques in data mining:
Generative Classification
It models individual class segmentation. The classification type uses the generation of data that occurs through assumptions and estimations. It learns from this generated data and predicts the unseen data.
Discriminative Classification
This is responsible for the classification of all rows involved in data. It uses the power of data quality to make accurate classifications of the data available.
Data Mining Classification as Per the Type of Knowledge Mined
Classification of data mining systems can occur relevant to the form of knowledge mined. This implies that the type is reliable on a few functionalities, namely:
-
-
- Correlation And Association Analysis
- Classification and Prediction in data mining
- Characterization
- Discrimination
- Evolution Analysis
- Outlier Analysis
Data Mining Classification Based on the Techniques Utilized
The classification techniques in data mining play a cardinality here. They can be defined in relation to the user interaction degree.
Data Mining Classification Based on the Applications Adapted
The applications that have been adapted play a key role in the classification of data mining systems here. These may range from:
-
-
- Stock Markets
- DNA
- Finance
- Email
- Telecommunications
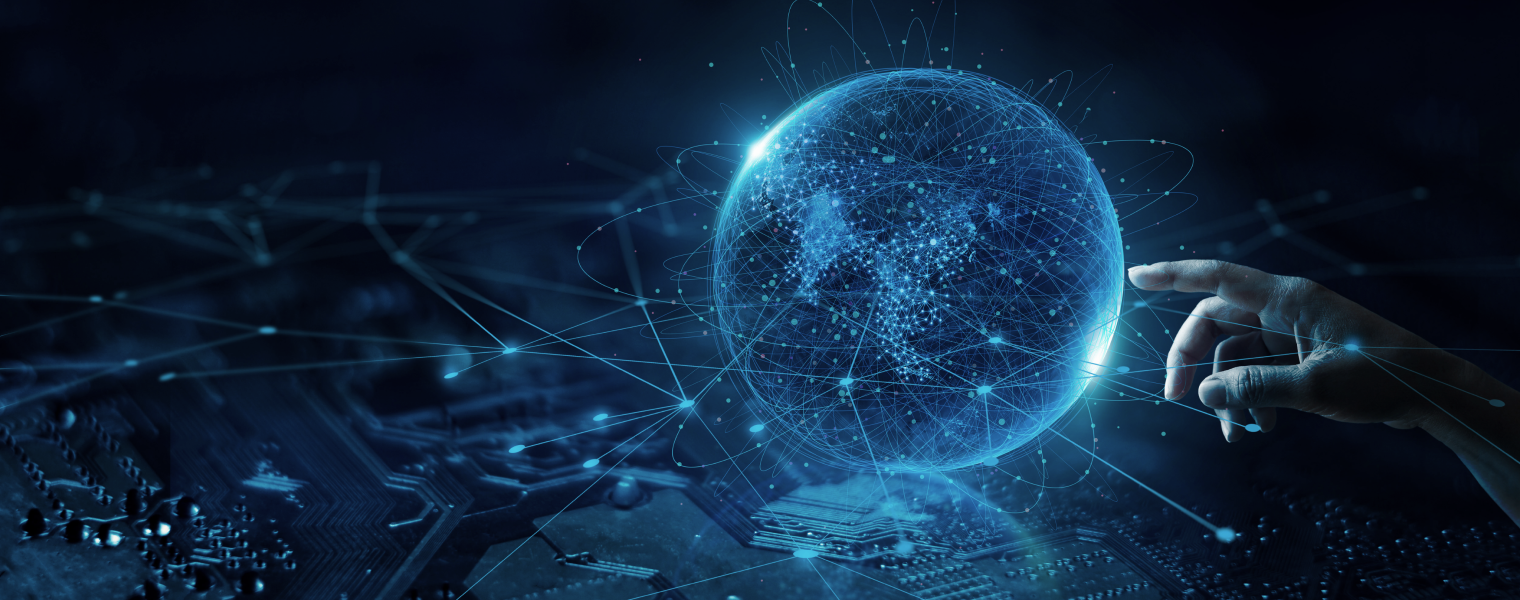
Internship Assurance
Business Analytics and Data Science
Classifiers in Data Mining
Below are the key data mining classifiers in detail:
Decision Trees
Here, the classification of data mining is robust. The flowchart it includes resembles the structure of a tree with the classes hanging on leaf nodes with labels.
The internodes contain decision classification algorithms in data mining. These are routed to the neighbouring leaf node.
Naive Bayes
This algorithm assumes that each parameter standing on an individual foot will have equal effects on the results. That is why they are also equally important. Naive Bayes estimates the event probability that it is to occur.
K-nearest Neighbors
It includes non-linear prediction boundaries. This is due to K-nearest Neighbors falling into the category of non-linear classifier. The classifier uses the k nearest neighbours class to make classifications and predictions in data mining concerning a new test data point.
Support Vendor Machines
Also called SVM, it reflects the in-space training data. These are segregated into distinctive categories using large gaps. Then, new data points are identified in this space, following which the prediction of categories is conducted with a focus on the gap side they belong to.
Random Forests
This one is compatible with numerous decision trees on diverse subsamples of databases. Here, the average is implemented to improve the accuracy of its predictions and administer overfitting.
Neural Networks
This classification of data mining method obtains the input and then learns to identify its patterns. This helps neural networks to make output predictions for similar new inputs.
Ensemble Method
It combines diverse models to empower the outcomes of machine learning. This process leverages better predictive performance production when in comparison to a singular model. Applications of Classification of Data Mining Systems
Marketing and Customer Analysis
Marketers focus on audience segmentation using classification algorithms in data mining. They chalk out their target audiences into varied segments leveraging algorithms. Thus, marketing and customer analysis are possible with efficient marketing strategies and accuracy.
Fraud Detection and Prevention
It becomes important for financial institutions to map out the profiles that deserve loan and card approval. So, they use classification in data mining to identify fraud which helps foster only authentic loan and card approval.
Medical Diagnosis
Diverse healthcare professionals use the power of classification algorithms in data mining to segment the risk of diverse diseases.
This classification of data mining systems helps them to mitigate health risks accordingly and boost timely recovery.
Conclusion
Classification of data mining systems involves complex processes. Initially, you may experience a daunting time learning about them. However, you are bound to find it easy to understand once you get acquainted with the diverse classification types and their outcomes.
You can also learn about data visualization tools to amplify your knowledge in this field of study. This can help you crack potential career prospects in Data Science without straddling the fence. Also, do not forget to consult your trainers about classification techniques in data mining for further inquiry upon the same.
FAQs
It is a colossal storehouse of data that comes from multiple sources within an organization.
Yes.
Data warehousing and data mining are interlinked, so they are not the same. The former is used to define the process of organizing and compiling data into one mutual database. On the contrary, the other involves a process where data extraction is implemented in numerous databases.
Classification of data mining systems encourages data confidentiality. They also enable data integrity and better accessibility.