The world, according to IDC, will generate 175 zettabytes of data by 2025. In other words, it is an exponential increase from the 33 zettabytes recorded in 2018. This gigantic amount of data presents huge challenges and opportunities for businesses. Management of raw data in multiple systems often prevents effective decision-making and lost opportunities.
A data warehouse is a centralised system that aggregates information from multiple sources. This is a structured environment specifically built to retrieve historical and real-time data and query and convert the information into actionable insights. It’s meant for analysis and reporting.
From predicting customer behaviour in retail to optimising healthcare operations, data warehouse technology is driving smarter strategies and more efficient operations. Let’s take a look at how this transformative tool is shaping industries and advancing business intelligence.
Features That Define and Differentiate Data Warehouses
So, what makes a data warehouse stand out? Here are its defining features:
- Centralised Data Storage: Data from sales, operations, and customer interactions are all consolidated into one platform. This eliminates silos and enables a holistic view of business operations.
- Historical Data Management: Unlike operational databases, which focus on live transactions, data warehouses store historical records. This helps us identify trends and make predictions.
- Fast Query Performance: By using specialised structures like OLAP (Online Analytical Processing), data warehouses handle complex queries quickly. Whether comparing yearly revenue or tracking marketing ROI, the answers come fast.
- Data Cleansing and Integration: Raw data often comes with errors or inconsistencies. A data warehouse ensures the information is cleaned, structured, and ready for use.
- Enhanced Security: Robust access controls protect sensitive business data. For instance, only HR personnel can access payroll details, while sales teams work with customer purchase histories.
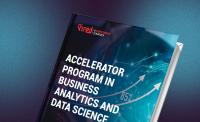
Get curriculum highlights, career paths, industry insights and accelerate your data science journey.
Download brochure
Architecture of Data Warehouses: Foundation for Applications
Every data warehouse is built on a strong foundation, and this comes down to its architecture. A poorly designed data warehouse will slow down operations. A solid architecture ensures speed, accuracy, and scalability so the warehouse grows with your business.
Let’s break it down into three simple layers.
The Bottom Layer: Collecting Data
This is where data enters the system. Information flows in from multiple sources:
- Operational databases
- CRM systems
- Transaction records
- External sources like social media metrics
Using ETL (Extract, Transform, Load) processes, the raw data is extracted, cleaned, and loaded into the warehouse. Think of this as filtering water before storing it in a tank.
The Middle Layer: Powering Analytics
Once stored, the data needs to be analysed. This is where OLAP systems come into play. OLAP lets us explore data across multiple dimensions—for example, analysing sales by region, year, and product simultaneously.
- ROLAP (Relational OLAP): Works with traditional relational databases.
- MOLAP (Multidimensional OLAP): Uses array-based storage for faster processing.
- Hybrid OLAP: Combines the strengths of both.
For example, an Indian banking firm may use OLAP to study customer behaviour by comparing monthly withdrawals, deposits, and loan repayment patterns. This helps them identify which services customers value most.
The Top Layer: Making Data Usable
This layer connects data to the end users through dashboards, reports, and visualisation tools. It transforms raw numbers into actionable insights.
For instance, a retail chain’s marketing team can use a dashboard to track the impact of its promotional campaigns in real-time. The visual clarity allows faster decision-making.
Layer |
Function |
Example |
Bottom (Storage) |
Data collection and cleaning |
Gathering sales data from Flipkart |
Middle (Analytics) |
Powering OLAP queries |
Analysing regional growth for ICICI |
Top (Visualisation) |
Dashboards and insights |
Tracking marketing ROI for Reliance |
Business Application of Data Warehousing Across Industries
Data is everywhere, but how do businesses turn it into something useful?
Scattered information makes decision-making slow and uncertain. With a data warehouse, businesses can consolidate data, uncover patterns, and act on insights efficiently.
Here are some specific ways data warehouses shine in different industries.
Application of Data Warehousing in E-Commerce: Using Data Warehousing to Drive Personalised Shopping
In e-commerce, speed and accuracy are critical.
Imagine a platform like Myntra analysing purchase history, browsing patterns, and feedback to recommend the right products. Data warehouses make this possible.
- Customer Insights: Analyse what shoppers buy most often and what they abandon in carts.
- Optimised Campaigns: Adjust marketing strategies based on real-time performance metrics like clicks and conversion rates.
- Inventory Management: Forecast demand during festive seasons like Diwali, ensuring popular products are always in stock.
Application of Data Warehousing in Retail Industry: Real-Time Inventory and Consumer Demand Insights
Retailers rely on precise data to keep shelves stocked with what customers want.
A chain like Big Bazaar can use a data warehouse to track which products sell best and adjust inventory levels dynamically.
- Fast-Selling Products: Identify and prioritise popular items in stores.
- Regional Preferences: Tailor stock based on local demand—for instance, more winter clothing in northern regions.
- Supplier Coordination: Communicate with vendors instantly to replenish stock based on real-time sales data.
Application of Data Warehousing in Healthcare: Improving Patient Care and Operational Management
The healthcare sector produces enormous amounts of data every day, from patient records to results.
Hospitals like Apollo can use data warehouses to streamline operations and improve patient outcomes.
- Treatment Insights: Combine both past and live time data to predict what patients need and reduce waiting time.
- Operational Efficiency: Analyse resource usage, such as bed availability and doctor schedules, to optimise hospital workflows.
- Individualised Treatment: Synthesize patient history with diagnostic findings to customise treatment strategies proficiently.
Application of Data Warehousing in Agriculture: Revolutionising Farming with Predictive Analytics
Farming is no longer about growing crops but about using data to grow them better.
Indian farmers can be helped through data warehouses in monitoring soil conditions for rainfall forecasting and, thus, yield improvements.
- Resource Management: Follow the pesticide application and avoid excess use.
- Meteorological Forecasts: Use past climatic data for scheduling agricultural cycles.
- Cost Optimisation: Examine costs for seeds and fertilisers. Determine where cost savings are possible.
Application of Data Warehousing in Climate Action: Data Warehousing for Environmental Sustainability
Climate action depends on accurate and timely data.
For organisations working on renewable energy projects, data warehouses help track progress and plan for future challenges.
- Carbon Footprint Monitoring: Consolidate data from various facilities to measure emissions.
- Renewable Energy Performance: Analyse energy output from solar and wind installations for better efficiency.
- Disaster Management: Use historical data to forecast and prepare plans for natural disasters such as floods or droughts.
Application of Data Warehousing in Banking and Financial Services: Advanced Risk Assessment and Fraud Detection
In the financial sector, data is the key to managing risks and improving customer experience.
Financial institutions such as HDFC can utilise data warehouses to monitor transactions and identify anomalous patterns.
- Prevention Against Fraud: Activity tracks in real-time to alert suspicious activities, such as multiple attempts to log in.
- Customer Insights: Analyse spending patterns that may suggest financial products such as loans or credit cards.
- Branch Performance: Assess profitability on the branch level to help guide decisions regarding branch expansion.
Application of Data Warehousing in Manufacturing and Supply Chain: Efficient Logistics and Supplier Management
Efficiency in supply chain management requires accurate and timely data.
A company like Tata Steel would leverage data warehousing to ensure control of production and delivery schedules.
- Vendor Performance: Monitoring the delivery time of suppliers and quality of goods to meet high standards.
- Inventory Optimisation: Know when the stock level is low and restock proactively.
- Shipping Efficiency: Make use of real-time information to optimise shipping routes and minimise transit time.
Application of Data Warehousing in Education: Data Warehousing for Learning and Administrative Excellence
Educational institutions handle vast amounts of data, from student performance to resource allocation.
A university like IIT Delhi can use a data warehouse to enhance both learning and management.
- Student Tracking: Monitor grades and attendance to identify struggling students early.
- Resource Planning: Allocate budgets for labs, libraries, and other facilities based on usage patterns.
- Admissions Analysis: Review application data to refine the admissions process and attract top talent.
Data warehousing is not only about storage and analytics. New trends change the way businesses use this powerful tool.
Integration of AI & ML
AI and ML are no longer buzzwords—they’re essential for data-driven decisions.
Advanced AI models are being trained with data. This provides organisations with an easy capacity to build predictive systems with relatively clean and structured data.
- Fraud Detection: Train models to spot irregularities in financial transactions.
- Customer Personalisation: Tailor product recommendations based on buying history.
- Operational Optimisation: Forecast demand spikes in manufacturing or service industries.
Reverse ETL for Actionable Data in Business Applications
Data sitting idle in a warehouse doesn’t add value. Reverse ETL ensures that insights flow back into operational systems.
This trend allows teams to use warehouse data directly in everyday tools like CRM or marketing platforms.
- Enhanced Campaigns: Sync customer data with tools like Salesforce to personalise outreach.
- Sales Efficiency: Equip sales teams with detailed client insights drawn from warehouse data.
- Real-Time Actions: Trigger automated workflows based on live data changes.
Cloud-Centric Data Warehousing for Scalability
The cloud has transformed data warehousing by providing unmatched scalability and flexibility.
Businesses no longer need to worry about hardware costs or maintenance. With cloud-native solutions, they can grow seamlessly.
- Cost Savings: Pay only for the storage and processing power you use.
- Global Accessibility: Access data from anywhere, enabling better collaboration.
- Quick Setup: Get started faster without the need for physical infrastructure.
Data Lakehouses: An Integrated Approach to Modern Data Management
A data lakehouse integrates benefits from both a data lake and a data warehouse. It supports structured and unstructured data, which makes it suitable for organisations working with varieties of datasets.
- Unified Storage: Store raw social media data alongside refined sales reports in one platform.
- AI Readiness: Use unstructured data like images and videos to train AI models.
- Simplified Management: Reduce the need for separate systems by integrating all data types.
Benefits of Data Warehousing Across Industries
Why do businesses invest in data warehouses?
It’s simple—data warehouses solve problems no other system can. When data is scattered across multiple systems, decisions become a guessing game. But with a data warehouse, you get clarity, speed, and confidence.
Here’s why businesses across industries rely on data warehouses.
1. Faster Query Performance
- Designed for speed, data warehouses handle complex queries efficiently.
- Example: E-commerce platforms restock items faster by analysing festive sale data in real time.
2. Improved Data Quality
- Data warehouses clean and organise raw information for accurate analysis.
- Example: Banks avoid duplicate entries and mismatched customer data, enhancing service quality.
3. Smarter Decisions
- Historical data provides context for informed decision-making.
- Example: Retail chains use three years of sales data to predict future trends and act strategically.
4. Cost Savings
- Centralising data reduces the need for multiple tools, lowering operational costs.
- Example: Manufacturing companies track inventory across multiple factories with a single system.
5. Scalability for Growth
- Data warehouses scale effortlessly to meet growing business demands.
- Example: Healthcare networks integrate new hospitals’ records seamlessly into their system.
These benefits are why data warehouses are indispensable for modern businesses. They don’t just store data—they unlock its true potential.
Challenges in Implementing Data Warehousing Applications and Solutions
No data warehouse is free of obstacles. Such obstacles can be overwhelming, but knowledge of them is the first stride towards overcoming them.
Challenges
Challenge |
Explanation |
Example |
High Initial Costs |
A data warehouse incurs enormous investment in terms of both hardware and software and qualified manpower. |
Small businesses might find it challenging, but cloud-based solutions like Google BigQuery or Amazon Redshift offer pay-as-you-go models to lower costs. |
Integration Complexities |
Combining data from multiple sources with different formats, systems, and inconsistencies creates integration issues. |
Logistics companies merging data from GPS trackers, order systems, and delivery apps can face chaos without proper ETL tools. |
Data Governance Issues |
Ensuring data is secure, accurate, and compliant is difficult. Mishandling sensitive data can lead to legal and reputational damage. |
Banks require strict controls to protect customer account details while maintaining transparency for audits. |
Performance Bottlenecks |
Growing data demands can strain the warehouse, leading to slowdowns without proper architecture. |
Retail chains analysing peak holiday sales may experience delays in decision-making due to slow query performance. |
Solutions
- Use Cloud Services: Increase capacity dynamically without any upfront investment.
- Invest in ETL Tools: Automate data extraction and transformation for seamless integration.
- Implement Data Governance Policies: Clear policies should govern access, security, and compliance with the data.
- Optimise Performance: Periodically change and test the architecture to absorb growing amounts of work.
Addressing these challenges guarantees a more seamless implementation and optimises the return on investment.
Conclusion
A data warehouse transforms scattered information into actionable insights, enabling businesses to stay competitive and agile. Its ability to centralise data, improve quality, and provide historical context ensures faster decision-making across industries.
The application of data warehouse technology increases efficiency, scalability, and innovation across the retail, banking, health, and agriculture sectors. New trends like AI integration, cloud scalability, and reverse ETL are changing the way its potential is applied, making it a non-negotiable for any modern enterprise. However, the challenges can be handled by strategic planning with the proper tools to make the implementation process smooth.
By harnessing the power of a data warehouse, businesses unlock the full potential of their data, paving the way for smarter and more informed decisions.
Unlock the true potential of instruments like data warehouses with up-skilling in cloud engineering and DevOps. The Certificate Program in DevOps and Cloud Engineering at Hero Vired teaches industry-aligned skills, thereby building capabilities in areas where innovation and efficiency are necessary. This hands-on program takes you forward in your career while leading the way for future success in data-driven applications.
FAQs
Data warehousing has great applications, especially in the retail, health, banking, and manufacturing areas. Any business that involves handling huge data volumes can benefit from it.
Challenges include high initial costs, integration complexities, and data governance. Proper planning and tools can address these effectively.
Start with a clear strategy. Use cloud-based platforms, invest in skilled teams, and prioritise data security and governance.
Updated on December 4, 2024