Generative AI has been a fastest ever growing field and has the capability to grow much further. In this day and age where technology surpasses so much of the human capabilities Generative AI is excelling through the roof. We as humans are not only getting dependent on these tools but also using them for other purposes.
It doesn’t matter if you’re in healthcare, finance, entertainment, art and design, or any other field, Generative AI makes it easy for the user in every aspect. Gen AI advancement goes beyond traditional artificial intelligence systems, which mostly concentrate on categorization and forecasting.
This introductory article on generative AI will teach you about the major objectives of generative AI, its operation, its difficulties, and its incredible advantages. You’ll witness instances from everyday life of how this technology is influencing the future. You’ll learn how Generative AI can be the next big thing in your career by the end.
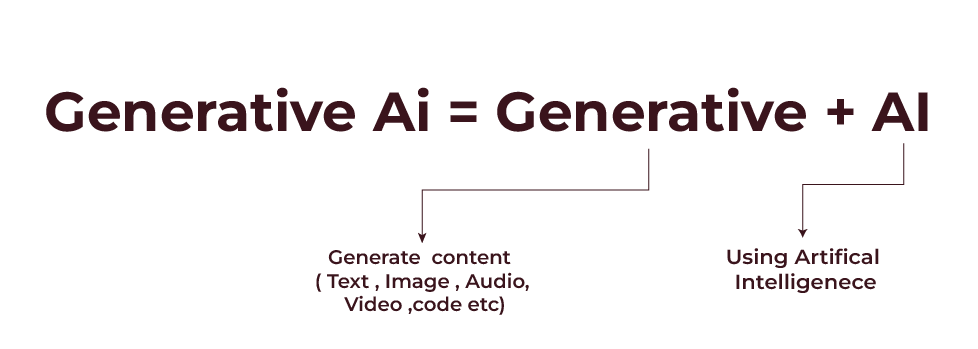
Working of Generative AI
Using the information and instructions provided, generative AI models employ neural networks to find patterns and structures in existing data in order to produce new and unique content. Different learning strategies, such as unsupervised or semi-supervised learning, can be used by generative AI models to train. Organizations can now more quickly and easily use a lot of unlabeled data to build foundation models thanks to this. As the name implies, AI systems that are capable of multiple tasks can be built on foundation models. GPT-3 and Stable Diffusion are two foundation models that let users take advantage of language’s power.
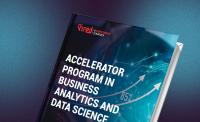
Get curriculum highlights, career paths, industry insights and accelerate your data science journey.
Download brochure
Evaluating Generative AI Models
The key requirements of a successful generative AI model are:
- Quality: Having high-quality generation outputs is essential, especially for applications that communicate directly with users. For instance, low-quality speech is hard to understand when it comes to speech generation. Similar to this, when creating images, the intended results should be identical to natural images in terms of appearance.
- Diversity: Without compromising the quality of its generation, a good generative model captures the minority modes in its data distribution. As a result, the learned models’ undesirable biases are lessened.
- Speed: For use in workflows for content creation, many interactive applications like real-time image editing—need to be generated quickly.
Generative AI applications
Numerous real-world uses for generative AI exist in fields like computer vision, where it can improve data augmentation methods. The use of generative models has essentially endless possibilities. Large volumes of data are processed by generative AI, which then produces answers and insights in text, picture, and user-friendly format. Generative AI can also be used for the following purposes:
- Boost consumer interactions with better chat and search functionality
- Investigate enormous volumes of unstructured data using summaries and conversational interfaces.
- Help with repetitive duties such as responding to RFPs, translating marketing materials into five different languages, ensuring that customer contracts are compliant, and more. A few well-known use cases with already astounding outcomes are listed below.
- Image generation
- Image-to-image translation
- Text-to-image translation
- Text-to-speech
- Audio generation
- Video generation
- Image and video resolution enhancement
- Synthetic data generation
Also Read: Top Generative AI Applications
Benefits of Gen AI
Generative AI acts as a powerful creative partner. Whether you’re an artist, writer, or designer, AI can help spark new ideas, explore different styles, and enhance your creative process. Let us take a look at a few of the benefits provided by Generative AI.
● Automation Of Creativity
Artificial intelligence (AI) tools that facilitate ideation and content creation are revolutionizing the creative industries. The use of artificial intelligence (AI-powered tools) opens up new creative possibilities and allows writers, designers, and artists to express themselves more fully.
● Efficiency
Because generative AI speeds up the creation of original content and automates repetitive tasks, it increases productivity across a range of industries. Businesses can now generate personalized content, enhance designs, and obtain new insights faster than ever before.
● Customization
Generative AI excels at providing tailored experiences. With the use of this technology, businesses can engage with clients more effectively and provide unique experiences to a broad audience. Examples of these experiences include customized user content and targeted advertising.
Practical Applications of Generative AI
Although use cases for generative AI are already emerging in the areas of synthetic data, generative engineering, generative design, creative content, and content improvement, the field will advance quickly in both scientific discovery and technology commercialization. High-level practical applications that are currently in use include the following:
- Augmenting and creating written content involves creating a “draft” output of text in the desired length and style.
- Answering questions and discovery: Giving users the ability to find answers to input by using data and timely information.
- Tone: Modification of text to make language more palatable or formal.
- Summarization: Presenting condensed forms of discussions, papers, emails, and websites.
- Simplifying involves dissecting titles, forming outlines, and identifying the most important content.
- Content classification for particular use cases: Sorting based on topic, sentiment, etc.
- Enhancing entity extraction, classifying sentiment throughout the entire conversation, and creating journey flows from broad descriptions are three ways to improve chatbot performance.
- Software coding includes the creation, interpretation, justification, and validation of code.
Emerging use cases with long-term impacts include:
- Creating medical images that show the future development of a disease
- Synthetic data helping augment scarce data, mitigate bias, preserve data privacy and simulate future scenarios
- Applications proactively suggesting additional actions to users and providing them with information
- Legacy code modernization
Risks of Generative AI
The risks posed by generative AI are substantial and changing quickly. The technology has already been utilized by a wide range of threat actors to produce artifacts to support increasingly sophisticated scams and “deep fakes,” or copies of products. Some oversight risks to keep an eye on are:
- Lack of transparency: Even the companies that developed ChatGPT and generative AI models don’t always fully understand how they operate, so these technologies are unpredictable.
- Accuracy: Sometimes answers generated by generative AI systems are fabricated and inaccurate. Examine each output for correctness, suitability, and practical value prior to relying on or disseminating information in public.
- Bias: Policies or controls must be in place to identify biased outputs and handle them in a way that complies with applicable legal requirements as well as business policy.
- Intellectual property (IP) and copyright: As of right now, there are no substantiated guarantees for data governance and protection in relation to private company data. Users should anticipate that whatever information they enter into ChatGPT and its competitors will be made public, and we recommend that businesses implement security measures to prevent accidentally disclosing confidential company information.
- Cybersecurity and fraud: Businesses need to ensure that mitigating controls are in place and ready for the use of generative AI systems by malicious actors for cyber and fraud attacks, including those that use deep fakes to social engineer staff members. Speak with your cyber-insurance provider to find out how much AI-related breach coverage is included in your current policy.
- Sustainability: Significant amounts of electricity are consumed by generative AI. To lessen the impact on your sustainability objectives, select suppliers who use high-quality renewable energy sources and minimize power consumption.
Challenges of Generative AI
As an evolving space, generative models are still considered to be in their early stages, giving them space for growth in the following areas.
- Scale of compute infrastructure: During training, generative AI models—which have billions of parameters—need quick and effective data pipelines. Generative models require substantial compute infrastructure, technical know-how, and capital investment to maintain and develop. Diffusion models, for instance, might need training on millions or billions of images. Furthermore, enormous computational power is required to train such large datasets, and AI practitioners must be able to acquire and use hundreds of GPUs for model training.
- Sampling speed: The time it takes to generate an instance may have latency because of the size of generative models. Conversations need to happen fast and accurately, especially for interactive use cases like chatbots, AI voice assistants, or customer support apps. Diffusion models’ slow sampling speeds have become more noticeable as their ability to produce high-quality samples has increased in popularity.
- Lack of high-quality data: Generative AI models are frequently employed to generate synthetic data for various use cases. Furthermore, not all domains have sufficient data to train a model. For instance, the development of 3D assets is costly and there aren’t many of them. For these areas to develop and mature, substantial resources will be needed.
- Data licenses: Many organizations struggle to obtain a commercial license to use pre-existing datasets or create custom datasets for the purpose of training generative models, which exacerbates the problem of a shortage of high-quality data. This procedure is crucial for preventing problems with intellectual property infringement.
Also read: Practical and Useful Generative AI Examples
Conclusion
An overview of generative artificial intelligence (AI) and its potential effects on different industries were presented in this article. Imagine a world in which artificial intelligence (AI) and humans collaborate to produce breathtaking works of art, moving music, and uplifting tales that uplift and unite us all.
There are many possibilities, difficulties, and exciting opportunities on this new journey. By fusing human creativity with AI’s remarkable capacity for creation, we can transform the way we create. Imagine writers who can quickly create captivating stories, musicians who can quickly compose songs, and artists who can quickly bring entire worlds to life with just a few words. Want to learn more about generative AI? Try the Certificate Program in Artificial Intelligence and Machine Learning by Hero Vired.
FAQs
Generative AI's primary objective is to produce new information or content that closely resembles the input data it has been trained on. This kind of AI is capable of producing content that is identical to that produced by humans, including text, music, images, and even videos.
When it comes to producing new content or images, finding patterns in data, or creating text, generative AI shines. On the other hand, predictive AI is perfect if you want to examine patterns and use the data to create forecasts and predictions that can influence choices.
The following are some possible advantages of applying generative AI: automating the laborious process of content creation by hand. lowering the time it takes to reply to emails. enhancing the answer to particular technical inquiries.
Updated on November 6, 2024