Data analytics gathers raw data and balances the scales for such information. It applies an algorithmic or mechanical process to develop insights and support decision-making. With the exponential growth in the volume of data businesses generate and the different kinds of data analytics needed to make effective decision areas critical, this article explores what data analytics are, their varied roles, and how they influence decision-making.
Data Analytics Introduction
Data analytics is how we look at data to extract useful information, make conclusions and support decision-making. Data analytics uses various analytic techniques to transform raw data into business intelligence, including basic statistics and complex machine learning.
The problem with growing businesses today is that they produce a lot of data from various sources, such as social media, sales transactions and sensors. This data is useful because it can help businesses know better what they are doing under the hood, what trends are, what their customers are doing, etc. Accurate decision-making, innovation, competitive edge, and data analytics helped companies differentiate themselves from competitors.
Also Read: Career in Data Analytics in India
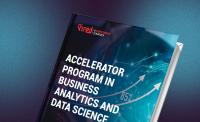
Get curriculum highlights, career paths, industry insights and accelerate your data science journey.
Download brochure
Types of Data Analytics
You have several different kinds of data analytics, each serving a different objective and providing different insights. Understanding these types is necessary so they can fully leverage the decision-making process.
1. Descriptive Analytics
Descriptive analytics is the simplest of data analytics. It says, “What’s happened?” by summarizing past data. Descriptive analytics focuses on looking at data, aggregating it, and mining it to see what happened in the past during a period.
Key Techniques and Tools:
- Data Aggregation: Fetching data from many sources and grouping them.
- Data Mining: Patterns for extraction of large datasets.
- Data Visualization: Showing data in charts, graphs and dashboards.
Applications:
- Business Reporting: Reports, other performance reports (e.g. Monthly sales reports) and financial statements.
- Trend Analysis: Futures strategy – knowledge of what happened in the past to understand what to do in the future.
2. Diagnostic Analytics
Descriptive analytics simply answers “What happened,” while diagnostic analytics asks ‘Why did it happen?’ This is an analysis of data to know what causes events and behaviour. Then diagnostic analytics is more complex than descriptive analytics, and we need more advanced techniques.
Key Techniques and Tools:
- Drill-Down Analysis: Details enable it to break data into finer details, thereby understanding the underlying causes.
- Correlation Analysis: Finding out relationships with other variables.
- Data Discovery: Data is explored using tools to explore data and find hidden patterns.
Applications:
- Root Cause Analysis: What causes operational issues and/or customer complaints?
- Performance Analysis: The ability to understand why some strategies worked or not.
3. Predictive Analytics
Predictive analytics looks at support for the following question: What could happen? The way we use historical data to forecast future outcomes. It uses statistical models and machine learning algorithms to locate trends or patterns that predict the future.
Key Techniques and Tools:
- Regression Analysis: Computer science is about analyzing relationships between variables to make predictions of future values.
- Time Series Analysis: Forecast future trends by looking at data points over time.
- Machine Learning: Algorithms that improve themselves someday through experience.
Applications:
- Sales Forecasting: It predicts future sales based on historical data.
- Risk Management: Potentially risks and their influence on the business.
4. Prescriptive Analytics
Predictive analytics predicts future outcomes, whereas prescriptive analytics provides suggested actions to achieve the desired results. The response to the question, “But What should We do?” uses insights from predictive analytics combined with techniques for optimization.
Key Techniques and Tools:
- Optimization Models: Solutions that best fit a set of constraints and objectives.
- Simulation: Predicting the outcome of different scenarios modelling.
- Decision Analysis: Making a more well-informed decision with the potential for negative impacts on different options.
Applications:
- Supply Chain Optimization: How the inventories should be best determined and which strategies one can use to distribute the inventories.
- Pricing Strategies: Fixing prices so that we maximize revenue and market share.
5. Real-Time Analytics
Real-time analytics processes the data as it becomes available, allowing immediate insights and the opportunity to work agile and, more importantly, to make timely decisions based on that data. This type of analytics is essential in a fast-moving environment.
Key Techniques and Tools:
- Stream Processing: Continuous data streams in real-time.
- Complex Event Processing (CEP): Detecting significant occurrences from event patterns.
Applications:
- Fraud Detection: This will identify fraudulent transactions as they are happening.
- Customer Experience Management: Monitoring and responding to the customer’s interactions in real-time.
6. Cognitive Analytics
Cognitive analytics uses artificial intelligence (AI) and machine learning to simulate human thought processes in analyzing complex data. It goes beyond traditional analytics by interpreting unstructured data such as text, images, and speech.
Key Techniques and Tools:
- Natural Language Processing (NLP): Understanding and processing human language.
- Deep Learning: Modeling complex patterns in the data with the help of neural networks.
- Text and Speech Analytics: Get insights from textual and spoken data.
Applications:
- Chatbots: Automated customer support and interaction.
- Recommendation Systems: Showing products or services based on user behaviour and preferences.
The Importance of Data Analytics
Data analytics is vital for several reasons:
- Better Decision-Making: Businesses can choose based on facts rather than guesswork with data analytics. As the data from the past tells companies what was and was not the case, they can see the trends and predict future outcomes accordingly.
- Increased Efficiency: Businesses can find more efficient ways to use data analytics. A look at a company’s operations can reveal bottlenecks, make processes easier, and free up company resources.
- Improved Customer Understanding: Businesses can find out how their customers behave and what they want by analyzing their customer data. Companies can tailor their product, service, and marketing efforts based on this better understanding of customer needs.
- Risk Management: Data analytics can do this by finding possible problems before they reach crisis proportions. Companies use prediction to get early warnings about trends or anomalies and proactively respond to keep risks from becoming actual events.
- Competitive Advantage: Using data analytics correctly means companies can stay one step ahead of their competitors. They can better respond to market changes, they can innovate continually, and they can give better outcomes for customers.
The Impact of Data Analytics on Decision-Making
Data analytics revolutionizes decision-making by systematically mining data and giving decision-makers valuable data-driven insights, revolutionizing the efficiency and effectiveness of decision-making. The integration of various types of data analytics helps organizations:
- Improve Operational Efficiency: By bringing in the bottlenecks and optimizing processes.
- Enhance Customer Experience: By personalized services and products.
- Drive Innovation: Discovering new peak insight and trend potentials.
- Mitigate Risks: By way of early detection and prevention strategies.
Conclusion
It is important to understand which type of data analytics you have and how you can leverage their full potential in your decision-making process. Six types of analytics are descriptive, diagnostic, predictive, prescriptive, real-time and cognitive, each with its benefits; when they work together, they provide comprehensive insights to drive strategic initiatives and enable innovation. These analytics techniques will have been mastered by the future success of tomorrow’s data as data increases in volume and complexity.
More generally, data analytics is the field and the tools and technologies that allow organizations to navigate the complexity of the modern business environment. Thus, it is indispensable to the search for a competitive advantage. Work your way up with this Certification Program in Data Analytics With Microsoft by Hero Vired, learn everything about Data Science professionally, and gain a certificate.
FAQs
Real-time analytics allow the data to be processed as it is generated to gain immediate insights and imminent decisions. This is critical in environments where optional speed is not an option, or at the very least, the cost merits a decision, or in fraud detection, customer experience management, or operational efficiency.
AI and machine learning improve at perceiving the world using patterns and applying human expertise to interpret unstructured data such as text, speech or images to uncover deeper, more complete human understanding. The power of handlebar.io is to process all sorts of data types, learn using machine learning, simulate human thought with chatbots, and offer personalized recommendations to customers.
Data analytics uses raw data to discover patterns, make conclusions and drive decisions. It is important because it allows for the creation of an ability that organizations can benefit from to inform their decisions, optimize their operation, better service the client or remain on par with competitors based on data-powered permeability.
Updated on December 13, 2024