Time Index
A fundamental element, the time index represents the chronological order of data points. Depending on the context, it could be in various units, like seconds, days, months, or years.
Data Points
These are the observed values corresponding to specific time instances. They could be measurements, counts, or any other quantifiable data.
Trends
Trends depict the long-term movement of the data over time. They indicate whether the values generally increase, decrease, or remain stable.
Seasonality
Seasonality captures recurring patterns within specific time intervals, often related to calendar seasons, days of the week, or months. These cyclic patterns can influence data behavior.
Cyclic Patterns
Unlike seasonality, cycles are not tied to fixed calendar periods. They represent longer-term undulating patterns that may not have a fixed duration.
Irregular Fluctuations
Also known as noise or random variations, these are unpredictable changes in the data that aren’t related to trends, seasonality, or cycles.
Outliers
Outliers are data points that deviate significantly from the overall data pattern. They could result from measurement errors, anomalies, or exceptional events.
Autocorrelation
This refers to the correlation of a data point with previous data points in the series. It helps identify dependencies and patterns within the data.
Stationarity
A stationary time series exhibits consistent statistical properties, like constant mean and variance. Stationarity is often necessary for accurate analysis and time series models.
Lags
Lags involve comparing a data point with past data points at certain time intervals. This is crucial for understanding relationships and predicting future values.
Missing Values
Time series data might have gaps due to various reasons. Dealing with missing values is essential for maintaining data integrity.
Click here to learn Big Data Analytics: What It Is, How It Works?
Time Series Data Visualization Techniques
Line Charts: A basic but powerful tool, line charts plot data points over time, illustrating trends and fluctuations. They’re perfect for showcasing gradual changes and spotting anomalies.
Bar Charts: Useful for comparing data at specific time points, bar charts show changes in categorical data over time or highlight differences between periods.
Area Charts: These build on line charts by filling the area under the line, making it easier to visualize cumulative data or compare proportions between different categories.
Heatmaps: Heatmaps display data using colors, revealing patterns through shades. They’re ideal for visualizing seasonal patterns or detecting correlations between time periods.
Seasonal Plots: Designed to reveal seasonality, these plots stack multiple periods on top of each other, aiding in spotting patterns that recur in specific time frames.
Box Plots: These illustrate the data distribution, showing median, quartiles, and potential outliers. They’re insightful for understanding how data varies across different times.
Histograms: By grouping data into bins and displaying their frequencies, histograms help comprehend the distribution of values and identify trends.
Time Series Decomposition
Time series decomposition is like dissecting a puzzle to reveal its hidden pieces. It’s a method that breaks down a time series data into its fundamental components: trend, seasonality, and residuals. Trend captures the long-term movement, seasonality unveils recurring patterns, and residuals are the unpredictable fluctuations. By separating these parts, we better understand how they contribute to the overall data.
This process helps us uncover valuable insights, predict future behavior, and make informed decisions. Just as an art restorer reveals a masterpiece’s layers, time series decomposition unveils the dynamics shaping our data-driven world. Further, it helps organize our time series analysis for efficiency.
Seasonal and Trend Analysis
Seasonal and trend analysis is like peeling back layers of a time-based story to reveal its core elements. In the world of data, many patterns repeat over time. The “seasonal” aspect captures these repeating cycles, like sales spikes during holidays or temperature changes across the year. On the other hand, the “trend” unveils the bigger picture, showing whether data is consistently going up or down.
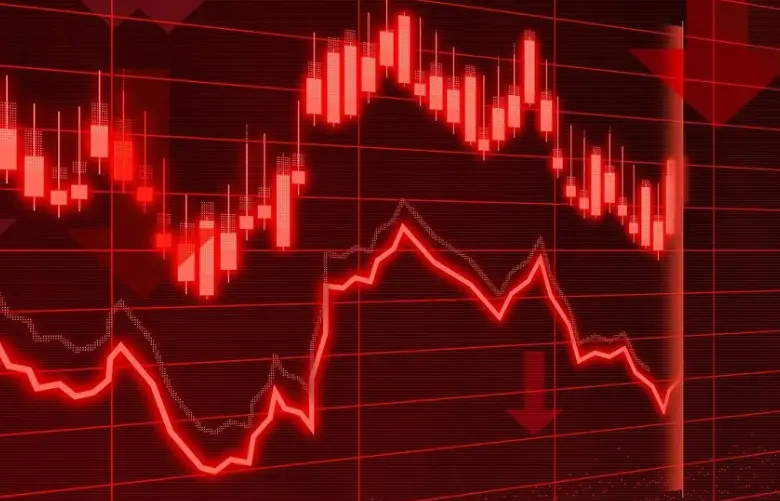
By dissecting a time series into these two parts, we grasp the short-term ups and downs as well as the overall direction. This time series analysis helps businesses prepare for busy seasons, spot long-term shifts, and make smarter decisions based on the ebb and flow of time-related data.
Statistical Measures for Time Series Analysis
Some of these measures include:
- Mean: This time series data analysis metric calculates the average value of the data points, giving us an idea of the central tendency.
- Median: The middle value in a dataset; this measure is less affected by outliers than the mean.
- Variance: Variance indicates how much the data points deviate from the mean, giving insight into the data’s spread.
- Standard Deviation: This is the square root of variance and helps us understand the average amount of variation from the mean.
- Autocorrelation: This measures the relationship between a data point and its past values, revealing patterns of dependence over time.