Data is one of the most powerful assets a business could own today. It’s everywhere: every interaction, every transaction, and every digital footprint generates an opportunity to collect valuable data that can be turned into operational transformation, increased productivity, and profitability if used correctly. Data-driven companies have used this potential to extract the insights that guide smart decision-making and find new growth opportunities.
Data must be analysed and understood, and it’s good to have data. At this point, fields such as Statistics and data analytics are entered. When applied properly, these disciplines help businesses decide what data is most important and how to organise, interpret and apply it to make well-informed strategic decisions. Statistics is about deriving insights using mathematically rigorous frameworks, while data analytics analyses these insights to solve real-world business problems.
This article looks into the differences between statistics and data analytics. That means taking those two fields simultaneously and showing how you can make the most of your data to realise business success, whether by turning raw information into a proprietary weapon.
What is Data Analytics?
Data analytics is a process of cleaning, transforming, examining and modelling your data to gain useful information and patterns. The goal is to mine insights from such datasets to assist decision-making. Today, data is used across several industries, from business and healthcare to finance and technology.
Data analytics typically involves several stages, including:
- Data Cleaning: Using data, finding and fixing errors in the data.
- Data Transformation: Turning raw data into something usable.
- Data Modeling: Trying to predict results using statistics and machine learning.
- Data Visualization: Processing the findings better through creating graphs, charts, and dashboards.
By creating visuals of the data that help them tell meaningful stories, businesses can make informed decisions to become more efficient, cost-effective and profitable.
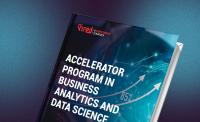
Get curriculum highlights, career paths, industry insights and accelerate your data science journey.
Download brochure
What is Statistics?
Data analysis is the branch of mathematics that deals with collecting, analysing, and interpreting data. It takes mathematical techniques to summarise or reduce data and infer conclusions from reduced data. Data are typically surveyed or collected from experiments or observational studies and analysed to determine sets, patterns, or relationships between variables.
That is the foundation for data-driven decision-making and research and is applied in science, economics, medicine and social sciences. Statistics enables businesses, governments, and researchers to understand data and make intelligent decisions.
Also Read: Statistical Analysis: Definition, Types, Importance & More
Some common statistical methods include:
- Descriptive Statistics: Summarizes and describes the features of a dataset (e.g. averages and percentages).
- Regression Analysis: Understanding of the relationships between variables.
- Hypothesis Testing: Using a small section of data to test assumptions or to test theories and conclude from that small section that the broader population does the same.
Key Differences Between Data Analytics and Statistics
While both fields involve analysing data to uncover insights, there are several key differences between data analytics and statistics:
Aspects |
Data Analytics |
Statistics |
Scope and Approach |
Data Analytics involves using broader tools and techniques, including statistical methods, machine learning algorithms and big data tools. It is more practical application-based and works to help businesses make decisions based on patterns and predictions. |
It concentrates on mathematical techniques and theories to understand and analyse data. Often, they consider sample data to know something about a larger population and try to quantify uncertainty. |
Tools and Techniques |
It uses many tools like Python, Data visualisation software and Machine learning algorithms. These tools will help process and analyse large datasets, identify trends, and predict future outcomes. |
Data analysis is more specialised in statistical methods such as regression analysis, correlation and hypothesis testing. With mathematical foundations and usually more limited to small datasets or samples, these methods. |
Purpose |
Data Analytics involves extracting actionable insights from data to enable an organisation to tackle a particular problem or make strategic decisions. Likewise, it also calculates future trends by using historical data. |
Makes attempts to validate results to ensure that conclusions reached from data stand up. Using hypothesis testing confirms whether observed trends or relationships are statistically significant. |
Data Size |
it works with big data (aka large volumes) and will need complex analytical tools and algorithms. These may come from social media, sensors and customer interactions. |
Most of the time, it analyses sample data that represent a small subset of a larger population. Statisticians use mathematical methods to infer something about the entire population from an observation or sampling. |
Real-World Applications of Data Analytics and Statistics
Data Analytics and Statistics are interrelated when converting the raw information into meaningful insight.
E-commerce
The e-commerce customers’ data analysis facilitates marketing, forecasts customers’ demands and possibly creates an ‘individual’ shopping experience for the consumers. Sales data and customer relationship feedback on a product can be used to fine-tune its offerings to match trends. The demand is also forecasted using machine learning algorithms to make predictions for inventory management and price optimiser.
Healthcare
Healthcare is today’s data analytics and statistical methods game. They contribute to increasing the quality of services by anticipating the outcomes of the patients, increasing resource utilisation, and, based on that, decreasing the costs of the healthcare delivery systems. It is, for instance, possible to use studies of patterns in patient data by healthcare providers to assess the occurrence of diseases and also to provide tailored treatment. Clinical trials are also crucial in statistics, where the latest drugs to be marketed have to be tested as harmless and effective.
Finance
In economics, statistical and quantitative analysis are employed in market and risk analysis and other aspects of finance. It is common for financial institutions to employ techniques that utilise data to identify extraneous information concerning fraud, as well as the other equations used to model the market to aid in trading. Finally, the theory is supported by other statistics means of relating risks, forecasting earnings, providing ideas for strategic planning, and enhancing business decisions by making them less costly and more lucrative.
Government and Public Policy
Government employees employ analytics to improve the services delivered to the public and inform policy choices that will meet society’s needs. For instance, health and economic predictors’ data can then be used to predict the change flow and manage resources better. Also needed are statistical tools for compiling, analysing, and reporting population, as well as survey and research data to provide the foundation for policy formulation and to address the needs of a changing populace.
Also Read: Top 14 Real-World Applications for Python to Know
Challenges in Data Analytics and Statistics
Both data analytics and statistics come with their own set of challenges:
- Data Quality: Bad quality or level of data is an issue in data analytics, leading to wrong conclusions. One of the biggest issues is getting the quality and consistency of the data.
- Big Data: For big data, you need special tools and algorithms. Big data must be stored and processed in real time to be resource-hungry.
- Bias and Overfitting in Machine Learning: Machine learning models can incorrectly predict if they are biased and can (or can not!) learn on biased data. One such issue is the problem of overfitting, where a model is so ‘locked in’ to the training data that it cannot generalise.
Also Read: Big Data Analytics: What It Is, How It Works?
Conclusion
In conclusion, data analytics and statistics, while they represent data, differ in methodology, techniques and objectives. Data analytics is not only statistics enhancing its integration with machine learning and data visualisation. It is something more; it is the field that focuses on providing businesses and organisations with solutions and advice based on their data. However, there is a problem with relying on statistical findings since they are built on mathematical models and data theories and produce results.
A crucial component and sub-process of IT in today’s data analysis involves using machine learning to give forecasts and guidance, self-execute and manage large quantities of information. Analytics, as a crucial part of comprehensive decision-making, needs both fields. However, because the tools and approaches differ based on the type of analysis necessary or the questions that may be asked, it becomes a question of what one wants to achieve. Quantitative data is a key component for coherently understanding our intricate world and interpreting and making sense of quantitative data, whether it is placing a bet on customer behaviour, advancing personalised health, or sector-specific trends. If you are interested in data analytics, go through the Certification Program in Data Analytics with Microsoft by Hero Vired to gain deeper knowledge.
FAQs
While data analytics explores events and explanations, Statistics compares them, giving weight to some explanations and casting doubt on others. Both processes are vital to a business's success, but the roles are organised differently. The toolset used by data analysts is more focused.
Statistics is used in biology, physics, chemistry, meteorology, sociology, communication, and information technology. For many of these categories, using statistics involves collecting data, analysing it, developing a hypothesis, and testing it.
Statistics are important because they can be applied to nearly everything, from personal life to critical and complex decisions by large companies and national governments. The results could be presented through tables, graphs, or charts to make them easy to understand and interpret. So, that's it! The four phases of a statistical investigation are to pose a question, collect data, analyse the data, and interpret the results.
Primary data refers to firsthand data gathered by the researcher himself. Secondary data means data collected by someone else earlier. Examples include surveys, observations, experiments, questionnaires, personal interviews, government publications, websites, books, journal articles, internal records, etc.
Updated on November 9, 2024