Understanding public sentiment from vast amounts of textual data has become crucial in today’s digitally interconnected world. Sentiment analysis, a powerful branch of natural language processing, aims to decode the emotions, opinions, and attitudes expressed within written content.
Whether social media posts, product reviews, news articles, or customer feedback, extracting insights from text can provide invaluable information for businesses, policymakers, and researchers.
In the section below, we’ll discuss sentiment analysis’s significance in deciphering the intricate tapestry of human emotions woven into text.
Role of Textual Data in Expressing Sentiments
Textual data serves as a rich tapestry for expressing sentiments and emotions. When writing, people naturally infuse their words with their feelings, opinions, and attitudes.
This makes written content a goldmine of information for understanding how individuals and groups feel about a particular topic, product, event, or situation.
Whether it’s the enthusiastic review of a new gadget, a passionate social media post about a social issue, or a cautiously worded news article, the words chosen and how they’re arranged can reveal joy, anger, concern, or many other emotions.
Analyzing this textual data allows us to unveil the intricate nuances of human sentiment.
Here are the Major Differences Between Data Science and Artificial Intelligence.
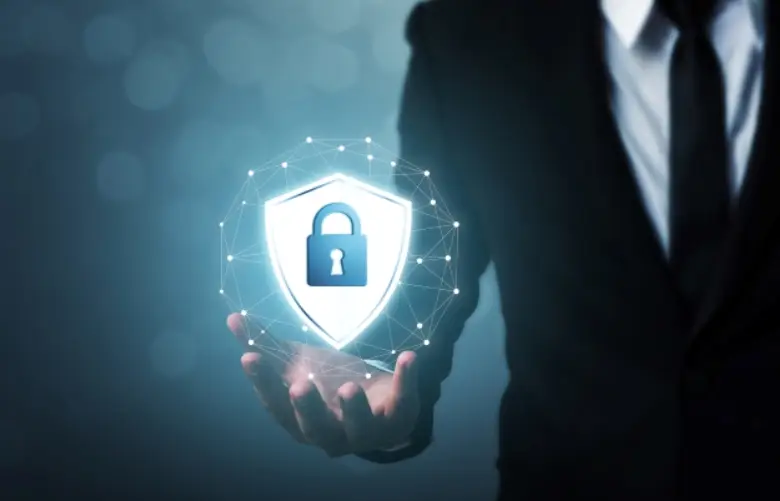
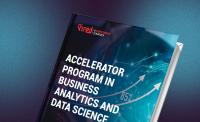
Get curriculum highlights, career paths, industry insights and accelerate your data science journey.
Download brochure
Challenges in Interpreting Textual Sentiments
Here are the challenges in interpreting textual sentiments:
- Words can have different connotations based on the surrounding words or the topic being discussed, making it tricky to determine sentiment accurately.
- Textual content frequently employs sarcasm and irony, where the literal meaning of words contradicts the intended sentiment.
- Deciphering whether a statement is positive, negative, or neutral requires understanding the complexity of these linguistic constructs.
- Sentiment analysis models must consider cultural variations in language usage and expression of emotions. What’s considered positive in one culture might be neutral or negative in another.
- Text can express a mix of positive and negative sentiments, reflecting the complexity of human emotions. These mixed sentiments can be challenging to quantify accurately.
Types of Textual Data Sources
- Social Media: Platforms like Twitter, Facebook & Instagram provide a treasure trove of real-time textual content.
- Product Reviews: Online marketplaces like Amazon and Yelp host customer reviews.
- Transcripts: Transcripts of interviews, speeches, podcasts, and videos capture spoken words that can be converted into textual data for sentiment analysis.
- News Articles: News outlets and blogs produce articles covering current events, politics, technology, and more.
- Blogs and Personal Websites: Bloggers and individuals share personal opinions, experiences, and narratives.
- Surveys and Feedback Forms: Organizations collect feedback from customers or users through surveys and feedback forms.
Basics of Sentiment Analysis
Positive Sentiment
When text is categorized as positive sentiment, the language expresses favorable emotions, happiness, satisfaction, or enthusiasm. This could include words that indicate joy, admiration, appreciation, and optimism.
For example, phrases like “amazing experience,” “loved it,” or “highly recommend” indicate positive sentiment.
Negative Sentiment
Negative sentiment arises when the text conveys unfavourable emotions, dissatisfaction, disappointment, or criticism. This category includes words and phrases that express anger, sadness, frustration, and dislike.
For instance, statements like “terrible service,” “disappointed with the quality,” or “won’t buy again” fall under negative sentiment.
Neutral Sentiment
When text is considered neutral, it lacks any solid emotional tone. The language used is typically factual and objective, without expressing strong feelings one way or the other.
Neutral sentiment is often seen in statements that provide information without conveying personal opinions or emotions. For example, “The weather forecast for tomorrow is sunny.”
Polarity Detection
Polarity detection is like tuning into the emotional frequency of text. It’s all about figuring out whether a piece of writing leans more towards a positive, negative, or neutral vibe.
Like you can tell if someone’s happy or upset from their tone of voice, polarity detection helps us decode the emotional tone hidden within words.
Whether it’s an enthusiastic product review, a critical movie comment, or a simple statement of fact, polarity detection adds a layer of understanding, letting us see the feelings and attitudes woven into the fabric of text.
Click here to learn about Understanding the Difference Between Artificial Intelligence and Machine Learning?
Preprocessing Text Data
Text Cleaning and Normalization
Text cleaning and normalization involve getting rid of unnecessary stuff in the text that might confuse computers, like extra spaces, punctuation marks, or special characters.
Normalization also means making everything consistent, like turning all letters to lowercase so that words like “Hello” and “hello” are treated the same way. This helps computers understand and process text more accurately.
Tokenization and Stemming
Tokenization is like breaking down a sentence into individual words. Just as you break a chocolate bar into smaller pieces, computers break sentences into words to understand them better.
Stemming is like finding the base form of a word. For example, “running” and “runs” both come from the base word “run.” This helps computers see that different word forms have a similar meaning.
Handling emojis, slang, and abbreviations
Handling emojis, slang, and abbreviations means making sense of people’s unique expressions in text. Emojis show emotions, slang is informal language, and abbreviations are short forms of words.
Computers need to understand these to catch text’s full meaning and emotions. It’s like learning to understand a new type of communication.
Supervised Sentiment Analysis
Creating Labeled Datasets for Training
Like learning from examples, computers learn to understand sentiments from labeled datasets. In supervised sentiment analysis, we provide computers with text and labels indicating whether it’s positive, negative, or neutral.
Feature extraction: Bag-of-words, TF-IDF
Feature extraction is about simplifying text for computers to analyze. Bag-of-words is like counting how many times each word appears in a text. This creates a “word checklist” that shows which words are present.
TF-IDF takes it a step further. It considers how often a word appears in one text and how unique it is across all texts.
Ethical and Privacy Considerations
Privacy concerns with analyzing public text data
When we analyze public text data, like social media posts, we dive into what people say online. But it’s important to remember that even though it’s public, it’s still personal.
Just like you might chat with friends in a public park, you wouldn’t want strangers eavesdropping on everything.
Privacy concerns in sentiment analysis remind us to respect people’s online conversations and not use their words without permission.
Bias and fairness in sentiment analysis
Sentiment analysis is like judging from one viewpoint. Just like a fair judge, computers must avoid bias by training on varied examples.
Ethical concerns remind us to respect online privacy and ensure fairness for diverse opinions, so sentiment analysis represents everyone accurately, without hidden biases.
Transparency in Reporting Results
Transparency in reporting means being open about how we reached our conclusions in sentiment analysis. Just like showing your steps in math, we explain our process, decisions, and results.
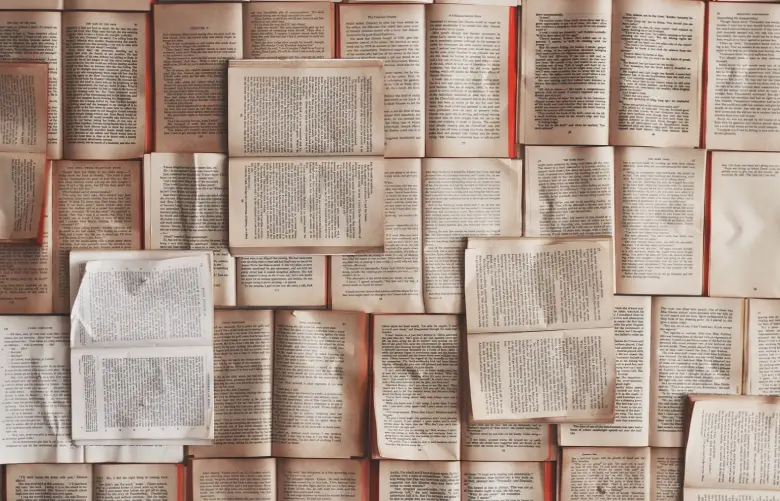
This builds trust by letting others see how emotions were analyzed, ensuring honesty and accuracy. It’s like sharing the recipe for our findings.
Conclusion
Sentiment analysis is like deciphering the language of emotions in text. Uncovering the feelings, opinions, and tones within written words provides a powerful tool for comprehending how people truly feel.
From social media to customer reviews, this technology helps us tap into collective sentiments, enabling informed decisions and deeper insights into public perceptions. Check out Data Science, Artificial Intelligence & Machine Learning course by Hero Vired to learn more.
FAQs
Sentiment analysis deciphers emotions from text, aiding insights into public feelings. It's a way to gauge opinions, attitudes, and reactions within written content.
Sentiment analysis classifies text as positive, negative, or neutral. It reveals emotions expressed in social media, reviews, and more.
Social media, reviews, news, surveys, and chats provide textual data for sentiment analysis, offering insights into public opinions.
Tools like NLTK, TextBlob, Lexalytics, and Clarabridge are used for sentiment analysis, assisting in decoding emotions within the text.
Process text, extract features, and apply machine learning with tools like NLTK or TextBlob. This helps classify sentiments in a dataset, providing emotional insights.