In the present age of big data, new opportunities rely more on predictive analytics to gain insights about things that are likely to happen. Predictive analytics combines historical data, statistical algorithms, and machine learning to equip organizations with the strategies they require to make better decisions, transform operational activities, and prepare for the problems most likely to occur shortly. This paper outlines predictive analytics, how it operates, when it can be used, and its advantages to organizations.
What is Predictive Analytics?
The business analytics system predictive analytics is a type of system wherein a mix of statistical methods, machine learning and data mining techniques are utilized to locate past and present facts that can be used to forecast future events. It is one of the ways of looking at data, and what comes out as an output of these predictive models forecast is the ability to make decisions, for instance, as and when required at the organization. From finance to marketing, even logistics, business intelligence is used to improve strategic planning.
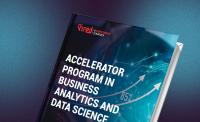
Get curriculum highlights, career paths, industry insights and accelerate your data science journey.
Download brochure
Six Predictive Analytics Examples
Predictive analytics can be valuable for companies split by industry or even something that can save the lives of those who need them most. An example of this is predictive analytics technology for local weather forecasts.
- Automotive industry: In the automotive industry, predictive analytics is used to manage the performance and quality of the building as well as customer satisfaction. For instance, Predictive maintenance gathers data from sensors fixed in vehicles to predict when a part may fail so that it can be replaced. This can decrease time off the road, increase vehicle performance, and increase customer satisfaction. The analytical models can assist in predicting customer’s future needs in terms of car models and the requirements for particular parts, which will assist manufacturers in arranging production and storing inventories.
- Financial Services and Risk Reduction: Predictive analytics is used for financial service management decisions. Financial institutions analyze historical data and customer behaviour to evaluate loan defaults, fraudulent activities, and credit risk opportunities. For example, banks predict if they are likely to lend to an individual based on their past payment behaviour, income level, and spending habits. It reduces financial risk and aids companies with better lending decisions. Moreover, it helps in fraud detection based on patterns and anomalies of this transaction that may lead to fraud.
- Manufacturing and Industrial Automation: Predictive analytics is on the cutting edge of the manufacturing world for optimizing production lines and increasing efficiency. Manufacturers can now predict when machines will fail or might perform poorly without unplanned downtime — with data from machines and sensors. The mining industry can also use predictive models to predict demand fluctuations so better inventory can be taken and production schedules more closely aligned with market demand. Through predictive maintenance, you know what machines you need to repair before they break down.
- Healthcare Industry: Predictive analytics in healthcare enhances patient care and organizational performance. For instance, one can use models to analyse patterns of patient readmissions and hence, hospitals can intervene to enhance the results. Risk analysis is also applied to disease prognosis; for example, estimating the patient’s probability of developing such illnesses as diabetes or heart disease, given their behaviour, heredity, and treatment history. This makes it possible for patients to be accorded individual treatment care plans to enhance their general health status and, at the same time, reduce treatment costs.
- Marketing and Retail: In marketing and retailing, predictive modelling assists companies in decision-making by providing customer insight. Sales forecasting, consumer buying behaviour, and stock management utilize retailers’ predictive models. For instance, a cloth seller might use predictive techniques to predict the demand for certain types of clothes relating to past seasonality and trends. This allows them to be sure they hold the best amount of the right product at the best time. Further, businesses’ application of predictive analytics facilitates customer categorization towards enhanced targeted marketing techniques, which enhances the customer attachment rate, thus boosting sales.
- Oil, Gas, and Utilities: Big data analytics is mostly used in asset management and operational asset management in the oil, gas, and utilities industries. For instance, an organization in the oil and gas industry will use a predictive model to determine when some equipment is likely to fail or require servicing to avoid, for example, an unplanned shutdown while at the same time improving safety. A second use case is the example of consumption predictors, which are generators that take the weather, previous consumption, and any other parameters to generate predictors for the forecasted energy demand to enable the provision of energy by the utilities at the least cost possible. In the oil and gas industry, the population uses predictive analytics to manage the drilling processes and improve the overall exploration performance.
Benefits of Predictive Analytics
Predictive analytics has become crucial for businesses, organizations, and industries, helping them anticipate future outcomes and make data-driven decisions.
- Improved Decision-Making: Data-driven insights provide organizations with predictive analytics that help them make informed decisions using their predictions. Accurate forecasts of what will happen will allow organizations to make strategic changes, resource effectively and plan for any possible challenge. It makes making better, faster, and more accurate decisions easier.
- Cost Reduction: The use of predictive analytics becomes helpful to businesses in identifying inefficiencies and areas of saving cost. Companies can forecast future trends to change their operations to maximize waste and attain better performance without much expenditure. Predictive maintenance can avoid the expense of expensive equipment breakdowns, while inventory forecasting minimizes storage costs.
- Risk Management: Assessing and mitigating risks is exactly what predictive analytics can do for you. Businesses can predict possible risks – financial, operational or reputational – and proactively minimize their effect. Predictive models are used in finance for credit scoring and fraud detection and in healthcare for detecting patients who are likely at risk for some conditions.
- Better Marketing Strategies: This tool allows businesses to create better marketing campaigns by forecasting a customer’s behaviour. Personalized offers or messages to the right audience at the right time increase conversion and ROI on marketing investment.
- Competitive Advantage: They use predictive analytics to predict market changes, demands, and customers, so organizations using predictive analytics are ahead of the competition. Forecasts of trends and consumer needs make possible planning by businesses that allow them to anticipate competitors’ actions, precede new products and services with them, and respond to changing conditions in the market.
Predictive Analytics Models and Techniques
- Classification Models: A classification model simply partitions data objects into other sub-segments to categorize each object as belonging to a specific type. These models answer questions like Yes and No about the data. They can be used to detect strange transactions on a farm or to examine the creditworthiness of a person or an organization. Classification models are of two general types: regression and decision tree models.
- Clustering Models: Clustering models use unsupervised learning to make open-ended decisions, such as sorting customers into audience segments. The model identifies patterns in the data to return the most effective means of organizing the data. Examples of clustering models include k-means clustering, mean-shift clustering, hierarchical clustering, and the Gaussian clustering model.
- Time Series Models: The more included, the better the models give information about when or how often something occurs. For example, time series models could give an insight into when certain months of the year will attract more traffic to your business. The two most frequently employed time series models are autoregressive and moving average.
- Neural Networks: Neural networks are artificial systems that mimic the workings of the human brain. This type of predictive analytics is suitable whenever the data have an unpredictable response to one another. For example, today, nodes of neural networks are used for content moderation services that delete toxic content by analyzing keywords and images.
Conclusion
Business forecasting uses quantitative methods to predict likely future scenarios based on past activity. Such methods as statistical analysis, machine learning and data mining will enable businesses to improve their knowledge of current and future trends and danger spots. Residents within sectors worldwide, increasing operational effectiveness, customer satisfaction, and predictive analytics make implementing better tactics in plenty of frontiers possible. With time and the availability of more data, the ability to harness predictive analytics to foretell the future of decision-making remains a possibility that every business will hold close to its heart for competitive advantage in a world with too much data. Gain industry-recognized skills and accelerate your career growth with the Accelerator Program in Business Analytics and Data Science Program offered by Hero Vired with edX aligned with Nasscom.
FAQs
Predictive analytics is a great solution that provides intelligent decision-making about expected future tendencies in combination with data. Using statistics, machine learning, and data mining, business organizations can gain insights in the short run concerning trend, risk, and opportunity analysis. Across industries, predictive analytics improve efficiency levels, availability, reliability and even customer satisfaction. In this context, predictive analytics's role appears promising as a predictor of the future and a guide to management decisions in a world where business opportunities are increasingly conditioned by technological progress and data availability.
Predictive analytics uses records of data, detailed patterns, and models to forecast occurrences. They use algorithms such as regression, decision trees and many machine learning algorithms to produce these predictions.
Predictive analytics uses structured data (e.g. databases, spreadsheets) and unstructured data (e.g. social media, customer reviews) to make predictions.
Yes, technical reasons include an application in a business where predictive analytics gain insights into areas of optimization and process improvement, where and when cost-cutting measures can be introduced to eliminate considered wastage.
Predictive analytics calculates customer behaviour, tendency to purchase or not, type of products of interest, etc. This assists business organizations in targeting consumers, enhancing communication, effective advertising, and enhancing product variety.
Updated on December 16, 2024