Today, data science has received a lot of attention owing to how it improves industries and the decision-making process. The rapid adoption of technology has made data-driven insights crucial for success.
This field is pretty dynamic and full of rewards, especially for problem solvers with technical skills. This trend has attracted many professionals and interns in this domain.
In this blog, we explore what data science is, why it’s an excellent career choice, the challenges involved, and the steps to start your journey.
What is Data Science?
Data science is simply defined as extracting useful information from data. It is the science that gathers large amounts of data using mathematics, statistics, and computer science, followed up with data analysis and modelling to draw useful insights out of such data. A data scientist processes data into usable information to help one make an informed business decision by finding trends in data.
This field is involved in working with machine learning, data visualisation, and predictive modelling. These methods allow data professionals to find solutions to complex problems. From determining customer behaviour to understanding the dynamics of the market itself, data science is able to offer intelligent solutions in many areas.
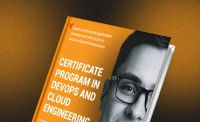
Get curriculum highlights, career paths, industry insights and accelerate your technology journey.
Download brochure
What Does a Data Scientist Do?
A data scientist position consists of several responsibilities, such as:
- Data Collection: Gathering raw data from multiple sources, such as databases, APIs, or user-generated data.
- Exploratory Data Analysis: This entails analysing a set of datasets with the aim of categorising them according to specific patterns, trends, and relationships.
- Model Development: This includes building predictive or analytical models using Machine learning and statistical techniques.
- Data Visualization: This includes the development of charts, graphs, and dashboards to enhance understanding of the findings made.
- Collaboration: It entails discussions and understanding goals with teams from different domains and providing them with data-driven solutions.
- Optimisation: This entails improving accuracy as well as efficiency through continuous improvement of the models and algorithms.
The role of a data scientist is not static, but it is a combination of technical and business-centred skills. Here, they are the solution providers whose role is to harness data for strategic purposes.
Is Data Science in Demand?
The demand for the data science field is pretty high since it is a part of many industries and relevant to a number of domains. According to the U.S. Bureau of Labor Statistics, there’s a projection of a 35% rise in data scientists jobs from 2022 up to 2032. This figure is pretty much higher than any other job.
This demand is observable in every area. In the health sector, data specialists assist in enhancing the quality of service and optimising processes. Retailers use data insights to understand customer behaviour and optimise inventory. These cases illustrate the universal need for data science.
There has been an increase in the skills in artificial intelligence and machine learning that is driving demand for the requirements of data science. Corporations spend money on AI projects, and they thus open new scopes of work for data scientists. The tech industry continues to look for data professionals even amid economic headwinds so as to stay ahead of the competition.
Reasons Why Data Science is a Good Career Choice
As a data scientist, you’ll enter into a field that is increasingly in great demand, and you’ll be learning dozens of exciting new technologies. Let’s see in greater detail why it is a good idea to pursue a career in this field:
High Demand Across Industries
Data science professionals are needed in almost every sector. Businesses have to make data-driven choices in order to stay alive. Data science isn’t simply helpful anymore, but it has become a necessity. This intimidating trend of dependence on data has brought a shortage of experts, and this means capable data scientists are in great demand.
- There are more jobs than qualified data scientists.
- Data drives insights for companies to increase their performance.
- Data is needed in Government bodies and NGOs as well.
Competitive Salaries
Working in data science can be a rewarding experience, owing to the high salaries one can receive. Data science is among the professions with the highest earning potential anywhere in the world.
Freshers who do not have relevant experience and relatively new jobs often pay higher than the industry standard for these positions. For seasoned experts, this figure goes into six figures.
- The average salary for data scientists in the United States is more than $120,000 per year.
- Above-average pay is usually offered for entry-level jobs.
- The executive level has a six-figure salary plus bonuses.
- Salaries are competitive worldwide, not just in tech hubs.
- In addition, certification and experience also increase earning ability.
Opportunities for Growth
There is a great scope for growth in a career in data science. Currently, professionals can choose to specialise in machine learning, artificial intelligence or big data engineering. The fast-changing technology world means that you always have new challenges to learn. In expanding your career path, you will also get chances to move into management or consulting positions, which offer you a range of career options.
- You may work in a specialised field, like AI or big data.
- The industry is constantly changing, so it is best to keep upgrading skills.
- Both startups and large companies are open to new talents.
- Career options are flexible and reflect the individual’s preference.
- Leadership roles are available for seasoned professionals.
Versatile Skill Set
The skills you acquire in data science are quite transferrable. Programming, data visualisation, statistical analysis and machine learning are some of them. With expertise in tools like Python, SQL, and R, data scientists can work across industries. Such an all-rounder profile guarantees jobs even in changing times and the ability to change roles if need be.
- Gain skills like programming and data analysis.
- Learn tools like Python, SQL, and Tableau.
- Skills are applicable across various industries.
- Versatility makes it easier to switch career paths.
- Adaptability makes sure that one is up to date with the current job trends and skills in the market.
Abundance of Internship Opportunities
In data science, an abundance of internship opportunities is presented, making it more effortless for novices to build practical know-how. The focus of these internships is to assist one with the use of tools and techniques on real-life projects. Whether a student or a working professional changes his profession, internships help individuals gain experience and are helpful in getting full-time positions in the relevant industry.
- Internships are offered in startups, multinational corporations, or even in government organisations.
- Such exposure enhances technical and troubleshooting capabilities.
- Internships are, therefore, useful for preparing a stunning portfolio for job purposes.
- There are chances of networking with industry practitioners when doing internships.
- Most of these internships are such that they convert the interns into permanent workers.
Opportunity to Work with Experts
In data science, one has the opportunity to engage with expert people in the industry and well-honed professionals. Working with professionals also provides support to understand various intricacies in the execution of large projects.
- Join together with qualified people and the industry’s key players.
- Learn from real-world problem-solving and innovative approaches.
- Learn about current industry tools and the workflows they follow.
Driving Innovation
Innovation is one of the core areas where data scientists focus, as they are instrumental in automating processes and decision-making. They help companies discover new opportunities, predict trends, and improve services. By leveraging data, they enable breakthroughs in areas like healthcare, environmental science, and customer engagement, making the role exciting and impactful.
- Facilitate the automation of activities using machine learning.
- Address relevant issues in the contemporary world using a quantitative approach.
- Assist in enhancing development in a variety of fields, for instance, in medicine and education.
Industries That Have High Demands for Data Scientists
Data science has revolutionised numerous industries, making it an essential field. Here are eight key industries where data scientists are in high demand:
1. Healthcare
Data science is transforming healthcare by improving patient care and operational efficiency. Predictive analytics helps identify potential health risks, while machine learning models support early disease detection.
- Enhances patient diagnosis and treatment plans.
- Assists in medical research and drug discovery.
- Optimises hospital resource management.
- Enables personalised medicine based on patient data.
- Predicts disease outbreaks for better preparedness.
2. Finance and Banking
Data science ensures secure transactions and enhances customer experience in finance and banking. Fraud detection systems and credit scoring models are among its key applications.
- Detects fraudulent transactions in real time.
- Helps assess creditworthiness for loans.
- Optimises investment strategies using predictive analysis.
- Provides personalised financial recommendations.
- Supports risk management and compliance.
3. Retail and E-commerce
Retailers use data science to analyse customer behaviour, predict trends, and optimise supply chains. This boosts sales and improves customer satisfaction.
- Creates personalised shopping experiences for customers.
- Predicts demand and manages inventory effectively.
- Analyses market trends for better product development.
- Optimises pricing strategies for maximum profitability.
- Improves customer retention through targeted marketing.
4. Technology and Software Development
In the tech industry, data science powers artificial intelligence, cloud computing, and automation, driving innovation and efficiency.
- Develops intelligent systems using machine learning.
- Enhances product performance through data-driven insights.
- Analyses user behaviour for software optimization.
- Improves cybersecurity using predictive models.
- Powers virtual assistants and recommendation engines.
5. Manufacturing
Manufacturers use data science to streamline operations, reduce costs, and improve quality. Predictive maintenance is a game-changer for equipment reliability.
- Monitors and predicts equipment failures.
- Optimises supply chain and inventory management.
- Enhances product design using data insights.
- Reduces production costs through efficiency models.
- Improves safety measures in factories.
6. Entertainment and Media
Data science helps entertainment companies understand audience preferences, recommend content, and optimise production processes.
- Personalised recommendations on streaming platforms.
- Analyses viewer preferences for targeted marketing.
- Optimises content production based on audience trends.
- Predicts box office or streaming success of content.
- Supports real-time audience engagement during live events.
7. Transportation and Logistics
Data science ensures efficient route planning and resource allocation, making transportation and logistics smarter and more reliable.
- Optimize delivery routes and fleet management.
- Enhances supply chain efficiency using predictive models.
- Improves public transportation systems through data analysis.
- Assists in vehicle performance monitoring and maintenance.
- Supports the development of autonomous vehicles.
8. Energy and Utilities
The energy sector leverages data science to improve efficiency and sustainability. It supports renewable energy integration and better resource allocation.
- Predicts energy consumption patterns for grid management.
- Enhances renewable energy adoption using data insights.
- Optimises utility operations for reduced wastage.
- Improves equipment maintenance through predictive analytics.
- Analyses environmental data for sustainability efforts.
Challenges in a Data Science Career
Data science is a very rewarding career, but with it comes the hurdles that one may face. Among them include:
- Quality Issues of Data: Data that is of not good quality may limit the extent to which analysis and predictions are done.
- Changes in tools and technology: As technologies are advancing quickly, professionals are required to continuously upgrade their levels of expertise.
- Complex Problem Solving: The creation of machine learning of models is often interspersed with unstructured data, making some of the tasks extremely difficult.
- Interdisciplinary Knowledge: A data scientist requires programming, statistics, and domain-specific expertise. This task can be overwhelming.
- Data Privacy Concerns: Sensitive data has to be treated with caution and in a limited way by only following the regulations of the organisation for privacy.
- Resource Limitations: Most small-scale organisations do not possess the resources and the infrastructure that will be able to analyse the data effectively.
- Team Collaboration: Technical findings are very difficult to communicate to non-technical stakeholders.
Who Should Consider a Career in Data Science?
Data science is certainly appropriate for people who love challenges and enjoy working with mathematics and numbers. It suits people from various backgrounds who have an analytical mindset and a desire to learn.
- Analytical Thinkers: A data science career is very satisfying if you love to find patterns and trends in the data.
- Tech Enthusiasts: People with an interest in the technology domain, programming and data visualisation tools would find this area rewarding.
- Statistical and Mathematical Minds: Those who are good at mathematics or statistics will be great at doing data science.
- Creative Problem Solvers: Those who can think outside the box and combine logic and creativity to solve problems.
- Professionals Seeking Growth: Perfect for those who want to move from the traditional realm of IT to areas which are in high demand.
- Lifelong Learners: People who are committed to mastering new technologies and following developments in the environment.
If you meet these characteristics, data science is probably the right career choice for you!
Different Job Roles For Data Scientists
Data science offers a variety of career paths, each with unique responsibilities and required skills. Let’s discuss 7 of the most important roles within the domain:
Data Scientist
Data scientists work with large sets of raw data, cleansing it and structuring it for analysis according to the business needs, developing trends and predictions from it. In order to overcome challenges in business, they utilise a variety of technical and analytical communication skills.
- Average Salary in the UK: £80,000–£1,70,000 annually.
- Tools used: Python, R, and machine learning libraries.
- Typical industries: Marketing, technology, and food.
- Requires an understanding of statistical analysis, AI, and at least one computer language.
Machine Learning Engineer
Machine Learning engineers create and implement algorithms that enable systems to learn and evolve. A key emphasis for them is building scalable design using the most appropriate technologies.
- Average Salary in the UK: £70,000–£1,50,000 annually.
- Common tools: TensorFlow, PyTorch and Scikit-learn.
- Typical industries: IT, e-commerce and health.
- Requires knowledge of neural networks and deep learning.
Statistician
Statistician’s work often includes designing surveys, managing sample distributions, and drawing conclusions. Such specialists are most frequently engaged in research activities and make significant vocational contributions.
- Average Salary in the UK: Between £65,000 to £1,15,000 annually.
- Typical industries: Health, education and the state.
- Tools used: SAS, R, and Python.
Data Architect
Data architects design the infrastructure that organises and secures a company’s data systems. They work closely with engineers to build scalable and efficient architectures.
- Average Salary in the UK: Between £90,000 to £1,50,000 annually.
- Focus areas: Data modelling and security compliance.
- Tools used: Erwin, snowflake and AWS.
- Typical industries: Information Technology services, finance and retail.
Data Analyst
Data Analysts are always dedicated to the right use of data in drawing useful information for the organisation’s decision-making. They are concerned with data cleaning activities, visualisation and reporting. Overall, this brings an association between raw data and strategy.
- Average Salary in the UK: £80,000–£1,35,000 annually.
- Common tools: Excel, SQL, Tableau, and Power BI.
- Typical industries: Retail, healthcare, and finance.
- It includes designing dashboards and reporting templates to assess performance.
Data Engineer
Data engineers design and manage high-performance systems used for the acquisition, storage, and retrieval of data. They also play an important role in providing data to analysts and scientists.
- Average Salary in the UK: £85000-1,15,000 annually.
- Common tools: Hadoop, Apache Spark, and ETL tools.
- Scope: Databases and big data.
- Typical industries: Banking, logistics, and telecom.
Business Intelligence (BI) Developer
BI developers interpret and present data in dashboards, charts, and reports. They have a specified concern with business issues and present complex data in pictorial formats to help solve such issues.
- Average Salary in the UK: £70,000–£1,50,000 annually.
- Common tools: Power BI, Tableau, and QlikView.
- Typical industries: Manufacturing, insurance, and consulting.
- Focus on automating reporting for feature stakeholders.
Data Science Future Outlook
Data science is evolving very fast, with a few key trends remodelling its future:
- Generative AI Expansion: Generative AI is being used in almost all sectors and industries and has created a cascading explosion in content creation, design, and problem-solving.
- Industrialization of Data Science: The evolution from custom-made data science procedures to automated, industrialised ones is a positive change as it enhances efficiency and scalability.
- Synthetic Data Utilisation: To address data scarcity, especially in AI training, the generation of synthetic data is becoming more prevalent, offering alternatives to real-world data.
- Integration of AI in Business: The integration of AI technologies in business is the upsurge in improving customer interaction, information processing, and targeted marketing efforts.
- Advancements in Healthcare: AI and data science technologies are increasingly transforming healthcare through disease prediction, tailored treatment and delivery methods enhancing patient management.
How Can You Start Your Data Science Career?
You cannot simply sway into a data scientist position, as it requires great commitment and a well-thought-out strategy. Follow these simple steps to get you going:
Step 1: Understand the Basics of Data Science
- Learn what data science is, its applications, and why it’s important in various industries.
- It is also important and helpful to master the data science processes. This involves collecting, cleansing, analysing and visualising data science.
Step 2: Build a Strong Foundation in Mathematics and Statistics
- Study topics like probabilistics, linear geometry algebra, calculus and statistical inference.
- First of all, it is very important to understand such fundamental concepts as regression, distributions, and hypothesis testing to be able to become efficient in data analysis.
Step 3: Learn Programming Languages
- According to the reports, the two most in-demand programming languages used for data science applications are R and Python.
- Try some coding exercises such as data manipulation, exploratory data analysis, and model building.
Step 4: Master Data Tools
- Know some SQL databases and know how to work with them.
- You can also try to understand the workings of basic tools for data visualisation. Some of the tools that you can use are Tableau, Power BI, Python’s Matplotlib, and Seaborn.
- Explore big data technologies like Spark and Hadoop.
Step 5: Familiarisation with Machine Learning
- Basic concepts like supervised and unsupervised learning, decision trees, and linear regression
- Followed by deep learning, neural networks, and NLP
- Practice model development by working on real projects using libraries like Scikit-learn, TensorFlow, and PyTorch
Step 6: Working on Real World Projects
- Apply the knowledge gathered on datasets from platforms like Kaggle, UCI Machine Learning Repository, or GitHub.
- You can make different projects like customer segmentation, sales prediction, or sentiment analysis to showcase your skills.
Step 7: Develop a Strong Portfolio
- Document your projects and push them up on GitHub.
- Always ensure that you mention each project with a proper README file. This will give users a clear indication of the steps for the approach taken, the tools used, and the results received.
Step 8: Network and Join Communities
- Use LinkedIn, forums, and meetups to connect with other data scientists
- Get involved in any of the following communities: Kaggle, Data Science Central, or the r/datascience community in Reddit to learn from and work with its members.
Step 9: Data Science Courses or Certifications
- Take courses through sites such as Hero Vired.
- Seek certification through Google Data Analytics Professional Certificate or IBM Data Science Professional Certificate.
Step 10: Internship and Entry-Level Jobs
- There are also internships, data analyst roles, or junior data science positions, which can always provide hands-on experience.
- Continue developing practical skills and understand how data science works in industry.
Step 11: Continuous Learning Improvement
- Keep updating your knowledge about new trends, tools, and techniques that are emerging in the field of data science.
- Study advanced topics like AI Ethics, Cloud Computing, and AutoML.
- Reed blogs, research papers, and case studies constantly to update your knowledge with deeper knowledge.
Conclusion
Starting your career in data science can seem challenging but working step by step and constant learning will make it look easier and accomplishable. A good grasp of mathematics, programming, and data tools will enable you to learn to analyse and interpret data.
All this, along with real-world applications, necessary certification and networking will allow the best sense of confidence in you, even in prospects in the field. Generally speaking, as the field of data science continues to grow, it will always keep a curious and ever-updated outlook on your workings. You must choose the Accelerator Program in Business Analytics and Data Science with edX and Harvard University aligned with Nasscom and Futureskills Prime by Hero Vired as it brings you an excellent professional view to expand your knowledge. This is a highly exciting and interesting subject which will help you thrive in this dynamic profession.
FAQs
Take online classes, implement projects that you are capable of executing, and engage in datasets in a number of ways.
Not necessarily, although having a relevant academic degree or certificate would be an additional advantage.
Python and R are the most widely adopted programming languages.
Work on concepts such as SQL, Tableau, Power BI, and frameworks for managing big data like Hadoop and Spark.
Yes, with the proper learning materials and constant hard work, data science can be transitioned to anyone in this world.
Updated on November 20, 2024