The interpretation of data and understanding of trends and prediction is a subject dependent on Statistics. It describes a way to analyse information effectively. Descriptive and inferential statistics are two of its branches, the methods you worked with to process data. Both have complementary uses, but it’s important to understand them to make informed decisions. In this guide, we’ll look into their meaning, uses, things in common, tools, and much more.
What Are Descriptive Statistics?
Descriptive statistics start by summarising and organising raw data into something meaningful. Measures like averages, proportions, and graphs provide a data snapshot. They make neither predictions nor conclusions about anything other than the dataset but reveal key facets of the data.
Key Features of Descriptive Statistics:
- Summarisation: It condenses large datasets into easy-to-understand metrics.
- Visualisation: It presents data using charts, tables or graphs.
- No Inferences: Nothing beyond the data is explained.
For instance, a company might use descriptive statistics to calculate the average sales, total revenue, and most popular products based on monthly sales data.
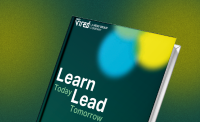
Get curriculum highlights, career paths, industry insights and accelerate your management journey.
Download brochure
What Are Inferential Statistics?
Inferential statistics can extend from simply describing data and giving probable predictions; they also test a hypothesis and conclude a larger population due to a sample. The value of this method lies in probability theory and statistical models for generalising populations from sample to population.
Key Features of Inferential Statistics:
- Generalisation: Inferential statistics allow conclusions about a population based on sample data.
- Hypothesis Testing: It validates or refuses to look at any given assumptions using statistical tests.
- Uncertainty Measurement: Provides quantification of the confidence level of results by probabilities and margins of error.
Example: To predict the voting behaviour of a city with a population of 3.5 million, a researcher studies 1,000 voters using inferential statistics.
Descriptive and Inferential Statistics: Key Differences
Aspect |
Descriptive Statistics |
Inferential Statistics |
Purpose |
It summarises and organises raw data. |
It makes inferences or predictions about a population. |
Scope |
Focuses only on the available dataset |
Extends findings beyond the dataset to a larger group |
Techniques |
A summary of measures of central tendency, variability along with visualisation |
Confidence intervals, regression, hypothesis testing |
Data Dependency |
It depends highly on the dataset given |
based on sample data that then infer population characteristics |
Examples |
standard deviation, mean, median |
chi-square tests, T-tests, ANOVA, regression analysis |
Description and Inferential Statistics Are Alike
While they differ in purpose and techniques, descriptive and inferential statistics share some commonalities:
- Data-Driven Nature: Both approaches in the operation are based on data. However, data is necessary to generate insights. Both crunch raw data to analyse, summarise, and arrive at conclusions.
- Statistical Foundations: Both use important statistical standards, such as probability, normal distributions, and sampling. Probability is instrumental in inferential statistics, hypothesis testing, and descriptive measures, such as standard deviation.
- Use of Visualization Tools: Charts, graphs, and tables are essential in both methods of presenting the data in a simple, lucid way. Descriptive statistics, for example, using frequencies in a bar graph and inferential results, can be summarised using confidence intervals or scatter plots.
- Complementary Roles: Inferential analysis usually commences with descriptive statistics. Researchers first summarise and organise their data using descriptive techniques before making inferences or testing hypotheses. For example, calculating the mean and standard deviation is a prerequisite of many inferential tests.
- Focus on Patterns and Relationships: Both methods seek patterns or relationships in the data. Descriptive statistics provide information on trends within the sample level, while inferential statistics provide generalised trends for the larger population.
Introduction to Major Types of Descriptive Statistics
Descriptive statistics can be categorised into three main types:
1. Measures of Central Tendency
These measures summarise the centre or typical value of a dataset:
- Mean (Average): The average value is the sum of all values of the two times a value number.
- Median: The middle data value when data is ordered.
- Mode: This is the most frequently occurring value.
2. Measures of Spread
These measures describe the dispersion or spread of data:
- Range: The range between the highest and lowest values.
- Variance: Squared difference of the average from the mean.
- Standard Deviation: This means measuring how spread the values are around the mean.
3. Data Visualisation
Visual tools help visualise data in an accessible format:
- Histograms: Show frequency distributions.
- Pie Charts: Proportions of categories are featured.
- Box Plots: They summarise data distribution and variability.
Inferential Statistics in Major Types
Inferential statistics includes advanced techniques to analyse data and draw conclusions:
1. Estimation
- Point Estimation: Offers a single value estimate of a population parameter, such as a sample mean.
- Interval Estimation: This gives us a range or confidence interval for the parameter to fall.
2. Hypothesis Testing
- Null Hypothesis (H0): It assumes a tied relationship or no effect.
- Alternative Hypothesis (H1): It assumes an important effect or relationship.
- Significance Level (α): Decides how often we reject the null hypothesis (p = example: 0.05).
3. Regression Analysis
- Assesses relationships between variables and predicts outcomes (sales based on advertising spend).
Modern statistics relies on various tools and software to perform analyses effectively:
Descriptive Statistics Tools:
- Excel: It’s great for quick calculations and data visualisation.
- Google Sheets: Good for basic statistical summaries and charts.
- Tableau: For creating high fidelity, interactive data visualisations.
Inferential Statistics Tools:
- SPSS: A place for hypothesis testing and regression.
- R Programming: Advanced statistical modelling Open Source software.
- Python: Statistical analysis is largely done through libraries like NumPy, SciPy, and pandas.
Statistical Literacy: Why It Matters
Today, Statistics matter much to professionals, students and decision-makers in our data-driven world. Here’s why statistical literacy matters:
- Informed Decision-Making: We interpret data so individuals and organisations can make evidence-based decisions. Businesses can use statistical insights to optimise operations or marketing strategies.
- Avoiding Misinterpretation: Reading data statistically requires statistical literacy to prevent misreading, which can distort data and lead to flawed conclusions. Understanding correlation vs. causation, for example, can help avoid providing inaccurate insight.
- Professional Relevance: In health, it is only possible to process and manage life conditions with statistical knowledge, such as risk assessment, finance, technology, trend analysis, and process improvement.
- Critical Thinking helps one understand real-world issues. Survey results about public health campaigns can be more significantly interpreted regarding their impact.
- Everyday Applications: For example, statistics explain polls during elections and personal investment options.
Statistical Analysis Beginning Practical Tips
Starting with statistical analysis can feel overwhelming, but these practical tips can make the process more manageable:
- Understand Your Data: First, define your data type as categorical or numerical. This will help you choose the right statistical methods.
- Start with Descriptive Statistics: Use mean, median, and standard deviation (or other summary) measures in the summary of your data. Patterns are used to be visualised in graphs and charts.
- Learn Basic Inferential Techniques: Learn confidence intervals, hypothesis testing and regression analysis so you can make data-driven predictions.
- Use Statistical Software: Automate the calculation using Excel, Python, or R tools and effectively lose the results.
- Check Assumptions: Before running your tests, ensure your data meets the required tests (e.g., normality and sample size).
- Practice with Real Data: Apply your knowledge with work on publicly available datasets. You will understand the practical problems and develop your skills.
- Learn Gradually: Master one statistical method at a time, learning the basics before advancing to more complex techniques.
- Seek Resources: Delve online tutorials, courses and books about statistical analysis.
Which Statistical Approach to Choose
Choosing the right statistical method depends on your goal and data type:
- Descriptive Statistics: When you want to summarise and visualise your data, use Descriptive Statistics. This extends only as far as the dataset, with no hint of extrapolation.
Example: Contributing to calculating average sales and visualising monthly trends.
- Inferential Statistics: However, we used Inferential Statistics when we wanted to use sample data to make predictions or draw conclusions about a population.
Example: A test of whether a new teaching method improves exam scores.
- Combine both approaches for a complete analysis: First, use descriptive statistics to understand the dataset. Then, use inferential methods to test hypotheses or predict future outcomes.
Conclusion
Knowing what descriptive and inferential statistics mean is crucial in effective data analysis. By summarising the data, descriptive statistics are basic, while inferential statistics are meant for prediction and generalisation. Together, they allow researchers, analysts, and decision-makers to unlock the maximum amount of data.
Learning both approaches will allow you to handle data confidently and precisely by analysing trends, testing hypotheses, or forecasting outcomes. The right tools and techniques can transform raw data into actionable insights that will help you drive success. Get your hands on and learn Statistics with Hero Vired’s Advanced Certification Program in Data Science & Analytics powered by the University of Chicago and get professional certification.
FAQs
Using descriptive statistics to summarise data without making predictions or generalisations is possible.
Examples are
hypothesis testing, regression analysis, and confidence intervals for inference on a population.
Descriptive and inferential analysis tools are Excel, Tableau, SPSS, R and Python.
Updated on January 21, 2025