In the captivating realm of Data Science, one can assert that it is a fusion of two domains: data and science. Data, whether tangible or conceptual, converges with the methodical exploration of the physical and natural realms—science. Data stands as the present, actively shaping the future. However, many concepts within the Data Science Life Cycle remain obscured due to a lack of clarity, often leading to a haze of vagueness surrounding project understanding.
The Data Science Life cycle revolves around employing machine learning and various analytical strategies to extract insights and predictions from data, aligning with commercial objectives. This comprehensive process comprises multiple steps, making it a lengthy procedure that may span several months.
Therefore, adhering to a standardised structure is crucial for tackling each unique challenge. A globally recognised framework for resolving analytical problems is the Cross Industry Standard Process for Data Mining, commonly known as the CRISP-DM framework. Intrigued by the field of Data Science? Let’s delve into the entire Data Science Life Cycle.
What Constitutes the Life Cycle of Data Science?
A data science life cycle delineates the iterative procedures undertaken to construct, deploy, and sustain any data science product. Given the diversity of data science projects, their lifecycles exhibit variations. Nonetheless, a generalised framework encompasses common data science steps, integrating machine learning algorithms and statistical practices to enhance prediction models.
Key stages in the data science process include data extraction, preparation, cleansing, modelling, evaluation, and more. Widely recognised in the field, this overarching process is termed the “Cross Industry Standard Process for Data Mining.” In subsequent sections, we will delve into each step, unravelling how businesses implement these processes in their data science projects. Before that exploration, let’s examine data science professionals’ roles in any project.
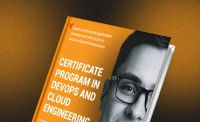
Get curriculum highlights, career paths, industry insights and accelerate your technology journey.
Download brochure
What Roles Do Various Individuals Play in Data Science Projects?
Explore the diverse individuals participating in the lifecycle of data science:
- Subject Matter Expert: Subject Matter Experts (SMEs) in data science are seasoned individuals with extensive domain-specific experience. Playing a vital role in the data science lifecycle, SMEs contribute valuable insights from their industry knowledge.They assist in problem identification, ensuring project goals align with industry nuances. SMEs collaborate with data science professionals to provide context, enhancing the accuracy of analyses and models.SMEs’ expertise extends to result interpretation, ensuring data-driven insights are statistically sound and relevant to real-world industry challenges. Acting as a bridge between technical aspects and industry intricacies, SMEs play a crucial role in the successful application of data-driven strategies within their specific domain.
- Business Analyst: Professionals adept at comprehending the business requirements of a particular domain or industry. Their primary duties involve identifying suitable solutions and timelines for the specified needs.
- Machine Learning Engineer: Tasked with advising on the appropriate model for generating the desired output. Additionally, they are responsible for devising suitable solutions to ensure accurate and requisite outcomes.
- Data Engineer and Data Architect: Finally, Data Engineers and Data Architects specialise in data modelling. From data visualisation to storage and retrieval, these experts manage all aspects of data handling.
The Data Science Life Cycle
It is crucial to recognise that many data science life cycle steps are involved in the Data Science life cycle process, which may differ based on various factors. Yet, the major steps in the Data Science life cycle project are given below:
- Problem Identification: Initiating any data science project involves understanding the problem at its core. Clearly defining the issue or query is essential before establishing project goals. In some cases, the problem is evident, while in others, defining clear objectives and specific challenges is the first step. For instance, discerning whether the objective is to reduce credit loss or predict product value sets the foundation for subsequent Data Science Life Cycle steps.
- Business Understanding: Business Understanding entails grasping the client’s needs from a business perspective. This includes determining whether predictions, sales improvements, loss minimisation, or process optimisation align with business goals. Two critical components of this stage are Key Performance Indicators (KPI) and Service Level Agreement (SLA). KPIs define project success, aligning business indicators with data science goals, while SLAs establish terms based on business objectives.
- Collecting Data: Data Collection forms a pivotal step in the Data Science Life cycle, providing the crucial foundation to achieve targeted business goals. Various methods, such as querying databases, using data science packages, accessing Web APIs, or downloading from repositories like Kaggle, contribute to gathering diverse data. Understanding data sources, types, relevance, and organisation is vital, involving technical skills like SQL querying and utilising visualisation tools for comprehensive data exploration.
- Data Pre-processing: Dealing with large, diverse data sets involves extracting, transforming, and loading (ETL) operations. Constructing a data warehouse and ensuring data uniformity are key in this stage. A data architect plays a crucial role in ETL operations, determining the structure of the data warehouse. Establishing a uniform format facilitates subsequent analysis and modelling.
- Analysing Data: Analysing Data is a pivotal phase in the Data Science Life Cycle where the prepared data undergoes in-depth scrutiny, commonly known as Exploratory Data Analysis (EDA). During this stage, data engineers leverage statistical tools and visualisation platforms such as Tableau or PowerBI to delve into the dataset. Their objective is to unveil patterns, identify significant variables, and visually represent the distribution of data. Exploratory Data Analysis is instrumental in extracting meaningful insights, guiding the formulation of hypotheses, and informing subsequent steps in the data science life cycle. By unravelling the intricacies of the data, analysts lay the groundwork for informed decision-making and the development of robust models.
- Modelling Data: Modelling Data is a crucial step in the Data Science Life Cycle that ensues data analysis, involving the refinement of the dataset based on identified patterns. The decision on how to model the data hinges on specific business requirements and the nature of tasks, whether they involve classification, regression, or other objectives. Machine Learning engineers play a pivotal role in this phase, employing a variety of algorithms to generate outputs that align with the project’s goals. To ensure the efficacy of the models, testing is conducted using dummy data before the final deployment. This iterative process allows for fine-tuning, optimising the models for real-world scenarios and enhancing their predictive capabilities.
- Assessing and Monitoring Models: Model Evaluation and Monitoring assess the effectiveness of various modelling approaches. Data Drift Analysis monitors changes in input data, while Model Drift Analysis uses techniques like Adaptive Windowing to detect shifts in models. Evaluating models with actual data ensures their adaptability and effectiveness in real-world scenarios.
- Model Training: Once tasks, models, and data drift analysis are finalised, model training in the Data Science Life Cycle commences. This phase allows fine-tuning of important parameters, exposing the model to actual data in production to monitor and enhance output accuracy.
- Implementing Models: Deploying the trained model involves exposing it to real-time data in the system. Models can be deployed as web services, embedded applications, or within edge and mobile applications, marking a critical transition from development to real-world application.
Extracting Insights and Generating Business Intelligence Reports: Following the deployment of the model, the data science life cycle enters a critical phase centred on extracting valuable insights and generating comprehensive business intelligence reports. At this juncture, the primary objective is to evaluate the real-world performance of the deployed model. The model, now operational, actively processes and analyses incoming data, producing a wealth of insights that serve as a foundation for strategic decision-making.These insights, derived from the model’s predictions and analyses, play a pivotal role in informing and guiding key decisions aligned with overarching business goals. The generated reports serve as powerful tools, systematically assessing key process indicators and providing a holistic view of business performance. Through these reports, stakeholders gain actionable intelligence, enabling them to identify trends, uncover patterns, and make informed decisions that contribute to the optimisation of business processes, identification of growth opportunities, and overall enhancement of organisational effectiveness.
This phase represents the culmination of the data science life cycle, where the transformation of raw data into meaningful insights empowers organisations to navigate the dynamic business landscape with confidence and agility.
- Making Decisions Based on Insights: The culmination of the data science life cycle involves leveraging insights for strategic decision-making. Properly executed data science steps contribute to reports that guide key decisions, allowing organisations to predict future needs, optimise processes, and foster business growth. The insights garnered play a crucial role in steering the organisation towards better revenue generation and sustained success.
In a Nutshell
A careful understanding of the data science life cycle and its proper implementation helps in business growth. Begin your data science journey by exploring the Accelerator Programme in Data Science, Artificial Intelligence, and Machine Learning at Hero Vired, which will equip you with the necessary skills for data analysis and the construction of sophisticated models, enabling you to address complex business challenges effectively. Acquire the skills needed to thrive in a data-driven future and chart a successful career trajectory in this dynamic field.
FAQs
The study of data science is suitable for individuals with a strong foundation in mathematics, as it forms the bedrock for delving into the intricacies of this dynamic field. A sound mathematical background is crucial because data science projects heavily rely on various statistical methods for analysis, interpretation, and drawing meaningful insights from data.
Proficiency in programming languages is equally essential for those venturing into data science. Programming skills, particularly in languages like Python and R, empower individuals to implement algorithms, process data efficiently, and create robust models.
This technical proficiency not only facilitates data manipulation and analysis but also enables the development and deployment of machine-learning models. Therefore, a combination of mathematical aptitude and programming skills forms the ideal groundwork for individuals seeking to excel in the study and practice of data science. In today's world, data reigns supreme. Whether it's a Boeing 787 aircraft or the daily use of mobile phones, everything generates and consumes data. A mere Google search or liking a post on Instagram contributes to this vast pool of data. Amidst this data abundance, we require individuals capable of managing and deriving meaningful insights from it, and that's precisely the role of a data scientist. Data Science is the craft of processing extensive volumes of big data and distilling valuable information from it.
Certainly! Data science is renowned for providing a secure and promising career path. The increasing demand for data-driven insights across various industries ensures ample opportunities and stability for professionals in the field.
Throughout different stages of data science, an array of tools proves beneficial. For analysis and visualisation, tools like PowerBI and Tableau excel. Python and R serve dual purposes in modelling and visualisation within the data science realm. Additionally, Spark and Hadoop come into play for efficiently processing streaming data and handling large-scale datasets.
Data Science stands as a field that offers substantial rewards, perhaps more than many others, but it demands adherence to a specific career trajectory to earn the title of a proficient data scientist. Initially, securing a bachelor’s degree in Computer Science (CS), Information Technology (IT), or Mathematics is imperative. Post-graduation, gaining hands-on experience is advised through entry-level roles like data analyst or junior data scientist.
For enhanced opportunities, pursuing a master’s or PhD becomes essential in the data science domain. Simultaneously acquiring a master's degree alongside entry-level employment is a viable option. Qualifications significantly influence career advancement, and after completing higher studies, one can aspire to take on the role of a senior data scientist.