In the contemporary data-driven world, there are many facts that encourage various agencies to make the right choice and win over the competition. Data analysis is one of such key tools, which can forecast trends and improve techniques in all types of businesses. With the help of this, you can translate raw data into meaningful insights that help organisations solve problems and work to achieve their goals.
Data analytics for commercial organisations offers a variety of strategies for capturing, processing, and interpreting data sets that result in actionable results. Companies use these insights to improve the buyer experience. It also helps in increasing operational efficiency and overall profit boost.
What Is Data Analytics for Business?
Business analytics basically refers to the steps being taken towards the analysis of business data towards a better interpretation and decision-making to reach core objectives. It forms the basis for identifying problems as well as opportunities and aids in converting the largest volume of data that business addresses into practical tasks. Such Business data analytics enables businesses to be relevant and competitive in this age of information.
By applying statistical tools, algorithms, and software, data analytics transforms raw numbers into actionable strategies. Organisations use it, for example, to enhance processes, understand their customers, and forecast. It is useful both in sales and marketing, finance and operations.
In other words, data analysis provides the information needed to make decisions with more confidence. It helps reduce risk, increase returns and ensure that the right decisions are based on measurable results.
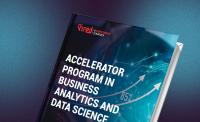
Get curriculum highlights, career paths, industry insights and accelerate your data science journey.
Download brochure
Types of Data Analytics Used By Businesses
1. Descriptive Analytics
Descriptive analytics is concerned with the summarisation of historical data and its attributes with a view to the prediction of future trends. The historical insights would answer the question of “what happened” with respect to sales performance or customer behaviour. Businesses use it to report and understand their current position.
Key Steps:
- Collect and organise historical data.
- Clean and preprocess the data for accuracy.
- Create visualisations, charts, and dashboards to summarise trends.
Challenges:
- Managing large volumes of data efficiently.
- Ensuring data accuracy and consistency across sources.
2. Diagnostic Analytics
Diagnostic analytics digs deeper to understand the “why” behind certain trends or events. It identifies root causes by correlating various data points and applying statistical techniques.
Key Steps:
- Perform drill-down analysis to explore detailed data layers.
- Compare data sets to identify deviations and correlations.
- Apply statistical methods to validate findings.
Challenges:
- Requires deep domain expertise to interpret results accurately.
- Time-intensive process due to the detailed nature of analysis.
3. Predictive Analytics
Predictive analytics uses historical data and statistical models to forecast future outcomes. It helps businesses anticipate customer needs, market trends, or risks.
Key Steps:
- Collect historical data and prepare it for modelling.
- Develop predictive models using machine learning or statistical methods.
- Train models and validate their accuracy on test data.
Challenges:
- Requires high-quality, structured data for accurate predictions.
- Demands significant computational power and expertise in advanced analytics.
4. Prescriptive Analytics
Prescriptive analytics provides actionable recommendations by analysing potential outcomes and suggesting the best course of action.
Key Steps:
- Use predictive models to evaluate potential scenarios.
- Apply optimisation algorithms to recommend the best decisions.
- Test recommendations against real-world conditions for reliability.
Challenges:
- Complexity in implementing models and interpreting results.
- Aligning recommendations with organisational goals and constraints.
5. Real-Time Analytics
Real-time analytics processes data instantly, enabling businesses to act on insights as events occur.
Key Steps:
- Set up systems to capture streaming data from live sources.
- Process and analyse data in real time using advanced tools.
- Visualise insights immediately through dashboards.
Challenges:
- Requires robust infrastructure to handle high-speed data.
- Ensuring system reliability and minimising downtime.
6. Text Analytics
Text analytics extracts insights from textual data, such as customer feedback, social media posts, or reviews.
Key Steps:
- Extract textual data from various sources.
- Process data using Natural Language Processing (NLP) techniques.
- Perform sentiment analysis and topic modelling to gain insights.
Challenges:
- Handling unstructured and context-sensitive data.
- Maintaining accuracy in diverse languages and contexts.
7. Spatial Analytics
Spatial analytics examines geographic or spatial data to derive insights related to locations.
Key Steps:
- Collect geographic data from sensors, maps, or databases.
- Apply spatial modelling techniques to analyse the data.
- Visualise results using GIS tools or spatial dashboards.
Challenges:
- Integrating geographic data with other business systems.
- Ensuring precision and accuracy in spatial data handling.
Each type offers unique advantages, but their effectiveness depends on addressing the associated challenges strategically.
How Does Data Analytics Help Businesses?
Data analysis helps businesses make informed decisions. It increases operational efficiency and overall efficiency by effectively leveraging data, and thus organisations can achieve greater efficiency and drive growth.
1. Enhancing Decision Making
Data analytics provides critical insights that support both strategic and operational decisions, leading to more effective outcomes and reduced risks for businesses.
Key Benefits:
- Informed Strategies: Enables the creation of data-driven strategies that align with business objectives.
- Evidence-Based Choices: Supports decisions with concrete data, reducing reliance on intuition.
- Performance Tracking: Allows monitoring and evaluation of decision outcomes over time.
2. Improving Customer Insights
It is important to comprehend how customers behave and the factors that inform their preferences in order to adequately target the right products and the associated services to the right market.
Key Benefits:
- Targeting: Helps to delineate particular audiences for specific marketing campaigns.
- Personalisation: Makes a better fit of products and services to individual customers.
- Consumer contentment: Enhances the overall customer experience through feedback analysis.
3. Optimising Operations
Re-engineering business functions results in better effectiveness and efficiency and economies of resource use.
Key Benefits:
- Processes efficiency: Identifies bottlenecks and areas for improvement within workflows.
- Resource allocation: Provides for appropriate use of resources so as to avoid wastage and minimise costs.
- Supply chain management: Improves the links and reduces time lag in the supply chain.
4. Increasing Revenue
Understanding the market and the clients through the use of data and analytics presents sales revenue expansion opportunities.
Key Benefits:
- Sales volume: Future sales should enable the planning of inventory purchases as well as sales and target marketing activities.
- Upselling: Selling the customers an extra service or product that complements their main purchase.
- Price setting: Optimises pricing based on market demand and competitive analysis.
5. Reducing Costs
Businesses can analyse their data and address areas of concern with unnecessary expenditure reducing their loss of revenue.
Key Benefits:
- Cost analysis: Discovers areas within a business that consume the most costs and are therefore the most driven.
- Operational Efficiency Enhancements: Focuses on the improvement of business processes to achieve lowered costs of operation outages.
- Reducing waste: Provides assurance of production processes and other business processes which minimise waste.
6. Enhancing Marketing Strategies
Creating successful marketing campaigns requires an accurate picture of market trends and customer habits.
Key Benefits:
- Campaign Transparency: Measures target audience reach for optimisation of marketing campaigns.
- Targeted Advertising: Enhances ROI by concentrating marketing on the most promising personae.
- Market Trends: Explores the potential target market and its expected developments in order to be competitive in developing the guiding marketing strategies.
7. Managing Risks
The system also assists with maintaining a stable business environment through routine risk identification and mitigation, thus assuring business sustainability.
Key Benefits:
- Risk assessment: Threats to the organisation are assessed and risk controls are developed.
- Fraud detection: Unusual behaviour trends that might signify fraud are detected and assets are safeguarded.
- Compliance monitoring: Legal issues are avoided by confirming compliance with laws and other requirements.
How Can Businesses leverage Data Analytics?
Businesses can use data analytics to follow a structured approach that aligns with their goals. Implementing an effective strategy ensures that data-driven insights are used to increase efficiency and drive growth.
Develop a Data Analysis Strategy
Creating a clear plan is essential for leveraging data analytics effectively.
Key Steps:
- Define Objectives: Identify specific goals, such as increasing sales or improving customer satisfaction.
- Select Relevant Metrics: Choose indicators that align with your business objectives.
- Plan Data Collection: Outline methods for gathering and storing necessary data.
Establish Data Management Systems
Implementing robust systems ensures data is handled efficiently and securely.
Key Steps:
- Choose Storage Solutions: Decide between cloud-based or on-premises data storage based on your needs.
- Ensure Data Quality: Implement processes to maintain accurate and consistent data.
- Implement Security Measures: Protect data from unauthorised access and breaches.
Conduct Data Analysis
Analysing data is crucial to extracting meaningful insights.
Key Steps:
- Use Analytical Tools: Utilise software like Excel or specialised platforms to analyse data.
- Apply Statistical Methods: Use basic statistics to identify trends and patterns.
- Visualise Data: Create charts and graphs to represent findings clearly.
Identify Data Analytics Sources
Understanding where your data comes from enhances analysis quality.
Key Steps:
- Internal Sources: Use sales records data, customer database, and operation metrics.
- External Sources: Use data from social media, market research, and industry reports.
- Evaluate Quality of Data: Assess the reliability and relevance of each data source.
Present Insights Effectively
Communicating data insights clearly helps stakeholders make informed decisions.
Key Steps:
- Create Visual Reports: Use graphs and charts to simplify complex data.
- Developed Interactive Dashboards: It provides real-time data access for decision-making.
- Tailor Presentations: Report Tailored to the different needs of your audience, be they executives or managers.
Consider Third-Party Solutions
Utilising external tools can enhance your data analytics capabilities.
Key Steps:
- Compare Analytics Platform: Compare the features and cost of analytics solutions, for example, Tableau and Power BI.
- Integration Assessment: Assess whether or not the analytics tools are going to be integrated easily into your systems.
- Scalable Solutions: Use scalable solutions that will grow with the needs of your business.
Formulate Insightful Questions
Asking the right questions directs your data analysis towards valuable insights.
Key Steps:
- Identify Business Challenges: Focus on areas that need improvement or growth.
- Develop Specific Queries: Create questions that target your objectives, such as “What drives customer loyalty?”
- Use Questions to Guide Analysis: Let your inquiries shape the data exploration process.
Implement Data-Driven Decisions
Integrating insights into your operations ensures practical benefits from data analytics.
Key Steps:
- Develop Action Plans: Create strategies based on data findings.
- Encourage a Data-Driven Culture: Promote the use of data in decision-making across the organisation.
- Monitor Outcomes: Track the impact of decisions and adjust strategies as needed.
Seek Patterns Everywhere
Recognising patterns in data can lead to innovative solutions and improvements.
Key Steps:
- Trend Analysis Periodically: Keep reviewing data to spot new trends.
- Apply Insights Across Departments: Use the findings to benefit any other areas of the business, such as marketing and operations.
- Foster Analytical Thinking: Allow your team to regularly look for patterns in their daily tasks and processes.
Explore Educational Opportunities
Enhancing your team’s data skills ensures the effective use of analytics tools and techniques.
Key Steps:
- Provide Training: Offer courses and workshops on data analytics and related software.
- Encourage Certifications: Support team members in getting the most relevant certifications.
- Encourage Continuous Learning: Keep abreast of the latest trends and innovations in data analytics.
With these steps, businesses will effectively support you in making an informed decision that leads to operations and growth. A systematic approach makes sure data is used strategically for long-term success.
Which is Better for Your Business: Business Analytics or Data Analytics?
Deciding between business analytics and data analytics depends on your organisation’s specific needs and goals. Though they are relatively similar since they both make decisions based on data, the purposes are different along with the area of application. The knowledge of differences would help you select the best strategies that would work the best for your business.
Business Analytics
Business analytics puts more emphasis on the use of data in a bid to improve business processes and outputs. It takes great emphasis on being able to analyse historical information with a view to making future informed decisions that will improve outputs.
Key Points:
- Purpose: Enhance business performance and decision-making.
- Scope: Primarily deals with business-related data and metrics.
- Tools: Utilises business intelligence software for reporting and visualisation.
- Benefits:
- Improves strategic planning.
- Enhances operational efficiency.
- Supports performance management.
Data Analytics
Data analytics is a more inclusive field that involves the analysis of different types of data to extract insights from them. It encompasses such techniques as predictive modelling and machine learning to predict trends and inform different decisions.
Key Points:
- Purpose: Extract comprehensive insights from diverse data sources.
- Scope: Encompasses both business and non-business data.
- Tools: Uses a wide range of tools, including statistical software and programming languages.
- Benefits:
- Provides predictive forecasting.
- Enables data-driven innovations.
- Supports various departments beyond just business strategy.
Key Considerations When Choosing
- Purpose and Goals
- Business Analytics: Best for improving specific business functions and strategies.
- Data Analytics: Suitable for a wide range of analytical needs, including research and development.
- Scope of Data
- Business Analytics: Focuses on internal business data.
- Data Analytics: This can include external data sources like social media and market trends.
- Tools and Techniques
- Business Analytics: Relies on business intelligence tools for dashboards and reports.
- Data Analytics: Employs advanced tools for statistical analysis and machine learning.
- Team Expertise
- Business Analytics: Requires knowledge of business operations and strategy.
- Data Analytics: Demands skills in data processing, statistical methods, and possibly programming.
- Implementation and Integration
- Business Analytics: Integrates closely with business processes and decision-making workflows.
- Data Analytics: This can be implemented independently for various analytical purposes.
- Cost and Resources
- Business Analytics: This may involve investment in business intelligence platforms and training.
- Data Analytics: This could require resources for data storage, advanced analytics tools, and skilled analysts.
- Flexibility and Scalability
- Business Analytics: Tailored to specific business needs and may be less flexible.
- Data Analytics: Emphasises having a more versatile structural approach that could work with various datasets and analytical approaches.
Making the Right Choice
Both business analytics and data analytics have their own merits, however, the choice that suits your business best depends on your goals:
- Consider Business Analytics if the sole purpose of your organisation is to improve performance and achieve certain targets.
- Consider Data Analytics if a wide range of target analysis is required with forecasting capabilities since it can cover many departments and has inputs from many types of data.
In many cases, integrating both approaches can provide a more comprehensive data-driven strategy, combining the focused insights of business analytics with the extensive capabilities of data analytics.
Roles and Duties of a Business Analyst and a Data Analyst
Organisations depend on Data Analysts to drive business success through informed decisions. With the careful insight Data Analysts provide, organisations increase efficiency and are able to work towards fulfilling their goals.
1. Data Collection and Management
It is the responsibility of Analysts to conduct research and consolidate data from various sources for the purpose of making it ready for analysis.
Key Responsibilities:
- Locating Data: Determine where relevant data can be obtained, such as databases, spreadsheets, or external sources.
- Data Mining: Apply necessary tools to collect the required information.
- Data Purification: The process of correcting errors and eliminating duplicates that are inconsistent data.
- Data Storage: Organise and store data securely for easy access and retrieval.
2. Data Analysis and Interpretation
An important part of Data Analysts’ work is to identify trends, patterns or relationships through the analysis of the gathered data.
Key Responsibilities:
- Statistical Analysis: Use statistical methods to analyse and determine trends within the data set.
- Trend Identification: Recognise patterns that can inform business strategies.
- Insight Acquisition: Helpful data is extracted from aggregated data analytics and is used to influence key decisions.
- Reporting: Stakeholders are presented with documents that contain particular analyses and their respective outcomes.
3. Data Visualisation
Presenting data in a clear and understandable manner helps stakeholders grasp complex information quickly.
Key Tasks and Responsibilities:
- Data Visualisation: Create visuals such as charts, graphs, and dashboards to communicate information in a convenient format.
- Software Applications: Create visual aids using tools such as Tableau, Power BI, and Excel among others.
- Data Presentation: Present complex data in a form that is easy to interpret.
- Advocacy: Alter the presentation to fit different audiences.
4. Collaborating with Teams
The role of a data analyst often requires them to liaise with different departments for their data requirements and initiatives.
Key Tasks and Responsibilities:
- Inter-departmental Collaboration: Work with other departments such as marketing, finance, and operations among others to understand their needs for data.
- Data Assistance: Aid departments in understanding data and how best to utilise the information for their work.
- Reporting: Brief and interpret the analyses proficiently to meet the teams’ strategies requirements.
- Encouragement: Advocating for the use of data information in decision-making processes throughout the organisation.
5. Developing Data Models
Data modelling assists in forecasting trends and results by analysing past data.
Key Tasks and Responsibilities:
- Forecasting Model Construction: Use statistical techniques and machine learning algorithms to forecast future events.
- Model Validation: Confirm the reliability of the models by validating them through a set of tests.
- Model Improvement: Adopting new information or feedback will allow the improvement of the model.
- Implement Models: Apply models to real-world scenarios to support business strategies.
6. Ensuring Data Security and Privacy
Protecting sensitive information is crucial to maintaining trust and complying with regulations.
Key Responsibilities:
- Data Protection Laws: Ensure data handling practices comply with laws and regulations like GDPR or HIPAA.
- Security Practices: Make use of encryption, access control, and other best practices to protect the data.
- Oversight of Data Usage: Know who is using the data, and why to ensure compliance.
- Security Threats: Try to assess and remove any potential risk for loss of data security.
7. Continuous Learning and Improvement
Data analysts will be more efficient in their work if they keep themselves abreast with the latest tools and methods available in the market.
Key Responsibilities:
- Market Knowledge: Keep up with industry trends, new technologies, and best practices in data analytics.
- Skills Acquisition: Pursue training and certifications to expand analytical capabilities.
- Adopt New Tools: Integrate advanced software and methodologies to improve data analysis processes.
- Seek Feedback: Regularly obtain feedback to refine analytical approaches and deliver better insights.
Conclusion
Data analytics will change the nature of businesses by giving them the power to provide insights that will influence informed decisions. The various kinds of analytics brought into interpretation will enable businesses to manage their activities, maximise customer satisfaction, and make revenues. Learn how to leverage data analytics for business with the Accelerator Program in Business Analytics and Data Science offered by Hero Vired with Nasscom and get a professional certificate.
In the dynamic market scenario, the need for analytics ensures that companies can fend off competition. Building the right infrastructure and acquiring the right skill sets will help companies use data for sustained growth and performance.
FAQs
Some of the most important uses of data analytics include many business goals like descriptive, diagnostic, prescriptive, diagnostic, real-time and geospatial analysis.
With the understanding of consumer behaviour and consumer tendencies that business analytics gives, organisations will be able to modify the products and services to suit individuals thus improving the customer experience.
The data analysis tools available which are prominent include Excel, Tableau, Power BI, Python, and R among other tools such as SAS and IBM Watson Analytics.
Some of the typical hurdles include guarding against the integrity of the data, working with very large volumes of data, combining data from several places, and employing qualified individuals to work on the analysis and interpretation of the data.
By applying predictive analysis, a business is in a position to predict upcoming trends in the market, understand the needs of clients in the future, and establish possible risk factors, thus averting risk during decision-making.
Updated on December 13, 2024