In today’s world, data is everywhere. From the information we share online to the statistics businesses rely on, data shapes our daily lives. Every day, large amounts of data are collected and used to guide decisions and strategies.
Managing this data effectively is crucial. People often hear terms like data analysis and data interpretation, but they might not understand what these terms truly mean or how they differ. Misunderstanding these concepts can lead to poor decision-making and missed opportunities.
In this blog, we will explore the differences between data analysis and data interpretation. You will discover how each process functions and why both are essential for making informed and effective decisions.
What is Data Analysis?
Data analysis describes the activity of studying information in order to extract useful knowledge. It involves structuring, cleansing, and interpreting such information to make it comprehensible. Usually, by analysing data, recurring issues and aberrations are recognised that help to solve the problem at hand.
This process is crucial and common in a variety of areas, for example, E-commerce, healthcare, education, etc. Raw data is converted into actionable insight through data analysis. It enhances the decision-making process by eliminating ambiguity concerning what the data says. Without proper analysis, data remains just numbers without any real value.
Key Features of Data Analysis
- Insight Generation: The process of uncovering untapped potential through information and trends.
- Informed Decision Making: Making decisions based on sound evidence that has information.
- Performance Measurement: Evaluating the performance of the different processes and processes and projects.
- Risk Management: Recognising existing and potential risks and if possible avoid them.
- Operational Efficiency: Eliminating redundancy within the processes for smooth operation.
- Customer Understanding: Paying more attention to the customers and their needs and requirements.
- Competitive Advantage: Utilising data so that one is always in front of the competition.
- Cost Reduction: Seeking the footholds to reduce costs but maintaining the expected quality.
These characteristics illustrate how data analysis works in turning raw data into relevant information. While concentrating on these results, organisations can make proper decisions with regard to their activities, and ultimately meet their targets. Data analysis also provides an answer to what is going on and a basis for the anticipation of events and trends that will happen in the future. Therefore, it becomes an important strategy for everyone who intends to utilise data with respect to his or her personal or working life.
Read More: Data Analysis Methods and Techniques
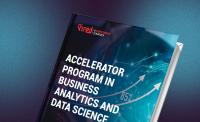
Get curriculum highlights, career paths, industry insights and accelerate your data science journey.
Download brochure
What is Data Interpretation?
Data interpretation is the phase that involves the interpretation of results after analysing data. It seeks to clarify the analysed data in a specific context. In other words, while data analysis is concerned with the handling and arrangement of data, interpretation seeks to explain the data in relation to the findings.
This phase is important because it is the link that connects the cold figures and statistics with practical action. As the definition implies, data interpretation simply means understanding the meaning of collected and analysed data in the context of these theories. Effective data interpretation transforms numbers and statistics into clear, understandable information that can guide actions and solve problems.
Key Features of Data Interpretation
- Meaningful Insights: Extracting valuable information that provides a deeper understanding of the data.
- Contextual Understanding: Relating data findings to the specific situation or environment.
- Drawing Conclusions: Formulating clear and logical outcomes based on the data.
- Communicating Findings: Presenting the interpreted data in an easy-to-understand manner for stakeholders.
- Guiding Decision-Making: Using interpreted data to support and influence business or research decisions.
- Identifying Implications: Recognising the potential impact of the data on various aspects of a project or organisation.
- Linking Data to Real-World Scenarios: Connecting data results to practical applications and real-life situations.
- Highlighting Trends and Patterns: Pointing out significant movements and recurring themes within the data.
- Providing Recommendations: Suggesting actionable steps based on the interpreted data.
- Enhancing Understanding: Improving the overall comprehension of the data through clear explanations and insights.
Thus, these features show how data interpretation brings value in terms of creating and shaping analysed data into useful stories. It makes sure that the information obtained as a result of data analysis is clearly utilised for the purpose of achieving some goals. In light of these factors, data interpretation aids in quality decision-making, process improvement, and success across multiple activities.
Read More: Statistical Analysis: Definition, Types, Importance & More
Key Differences Between Data Analysis and Interpretation
Aspect |
Data Analysis |
Data Interpretation |
Definition |
Examining data to find patterns and insights. |
Explain what the analysed data means. |
Purpose |
To organise and process data. |
To make sense of the processed data. |
Focus |
On the data itself and its characteristics. |
On the implications and meanings of the data. |
Process |
Involves cleaning, transforming, and modeling data. |
Involves summarising and explaining the results. |
Outcome |
Generates raw insights and trends. |
Provides conclusions and actionable recommendations. |
Tools Used |
Software like Excel, SQL, and statistical tools. |
Tools for reporting and visualisation like dashboards. |
Skills Required |
Technical skills in statistics and data handling. |
Analytical skills to interpret and communicate findings. |
Timing in Workflow |
Comes before interpretation in the data workflow. |
Follows data analysis in the data workflow. |
Example |
Identifying sales trends over the past year. |
Explaining why sales increased in a specific quarter. |
Nature of Work |
More technical and quantitative. |
More qualitative and explanatory. |
Decision-Making Role |
Provides the data needed for decisions. |
Helps in understanding the best actions to take based on data. |
Communication |
Focuses on presenting data clearly. |
Focuses on telling the story behind the data. |
Dependency |
Can be done without immediate interpretation. |
Relies on completed data analysis. |
Examples in Use |
Calculating average customer spend. |
Determining factors that influence customer spending. |
Value Addition |
Turns raw data into structured information. |
Adds meaning to structured information for better insights. |
Importance of Both Processes in Decision-Making
Data analysis and interpretation are vital for making informed decisions across various fields. Together, they transform raw data into actionable insights, ensuring that choices are based on solid evidence rather than assumptions.
Key Areas of Impact:
- Business:
- Strategy Development: Identifies market trends and customer behaviours.
- Performance Improvement: Guides marketing and inventory strategies based on sales data.
- Competitive Edge: Helps businesses stay ahead by anticipating market changes.
- Healthcare:
- Patient Care: Reveals common health issues and treatment effectiveness.
- Policy Making: Informs the development of better care plans and operational policies.
- Efficiency: Enhances the efficiency of healthcare facilities through data-driven decisions.
- Education:
- Learning Outcomes: Highlights student performance trends and areas needing improvement.
- Teaching Methods: Enables educators to create targeted teaching strategies.
- Support Systems: Develops support mechanisms based on data insights to improve educational outcomes.
- Government:
- Policy Formulation: Uses demographic and economic data to create effective policies.
- Resource Allocation: Ensures resources are distributed where they are most needed.
- Public Services: Enhances the delivery of public services through informed decision-making.
- Everyday Life:
- Personal Finance: Assists individuals in managing their finances wisely.
- Health Decisions: Guides personal health and wellness choices.
Best Practices for Effective Data Analysis and Interpretation
To achieve meaningful and reliable results, it’s essential to follow best practices in data analysis and interpretation. These practices ensure that the processes are efficient, accurate, and produce valuable insights.
Best Practices Include:
- Ensure Data Quality:
- Accuracy: Verify data for correctness.
- Completeness: Ensure all necessary data is collected.
- Consistency: Maintain uniform data formats and standards.
- Define Clear Objectives:
- Goal Setting: Clearly outline what you aim to achieve.
- Focused Analysis: Select methods and tools that align with your objectives.
- Use Appropriate Tools:
- Software Selection: Choose tools like Excel, SQL, Python, or Tableau based on your needs.
- Tool Proficiency: Ensure proficiency in the selected tools to maximise efficiency.
- Employ Statistical Methods:
- Technique Selection: Use the right statistical techniques for your data type and goals.
- Robust Analysis: Apply methods that provide reliable and valid results.
- Maintain Transparency:
- Documentation: Keep detailed records of your analysis process.
- Reproducibility: Ensure that others can replicate your analysis if needed.
- Focus on Visualisation:
- Clear Charts: Use charts and graphs to make data easily understandable.
- Highlight Key Trends: Emphasise significant patterns and insights through visual aids.
- Interpret in Context:
- Contextual Awareness: Consider the environment and circumstances surrounding the data.
- Relevant Conclusions: Draw conclusions that are meaningful within the specific context.
- Validate Findings:
- Cross-Verification: Compare results with other data sources or methods.
- Error Checking: Identify and correct any discrepancies or errors in the analysis.
- Communicate Clearly:
- Simple Language: Use straightforward language to explain findings.
- Target Audience: Tailor your communication to the understanding level of your audience.
- Implement Feedback:
- Review Process: Regularly seek feedback on your analysis and interpretation.
- Continuous Improvement: Use feedback to refine and enhance your processes.
Also Read: Exploratory Data Analysis : Unveiling Data Insights
Conclusion
Understanding the difference between data analysis and interpretation is necessary for effective decision-making. Data analysis involves exploring and understanding data to identify patterns and insights, while interpretation seeks to clarify the significance of such insights in context.
This way, both processes work together to make actionable strategies for the company’s growth. Furthermore, you can follow the best practices in this blog to achieve the desired outcomes through data analysis and interpretation. Learn more about data analysis and interpretation with the Integrated Program in Data Science, Artificial Intelligence, & Machine Learning offered in collaboration with MIT Open Learning by Hero Vired and get professional guidance and certification.
FAQs
Data analysis provides the facts, while interpretation adds meaning. Together, they enable informed and effective decision-making.
Data analysis involves examining and processing data to find patterns and insights. Data interpretation explains what these insights mean in a specific context.
Yes, but the results may lack context and actionable meaning. Interpretation is needed to make the insights useful.
Practice explaining data findings in simple terms, understand the context of the data, and develop strong analytical thinking skills.
Industries like business, healthcare, education, government, and technology greatly benefit from these processes.
To some extent, yes. It involves understanding the context and can be influenced by the interpreter’s perspective. However, it should be based on objective data.
Updated on December 24, 2024