In the realm of data analysis, the process of transforming raw data into meaningful insights is a crucial step. Data preprocessing, often described as the “secret ingredient” in the analytical recipe, plays a vital role in ensuring the quality and accuracy of results. By refining, cleaning, and organizing data before analysis, this stage addresses potential errors, inconsistencies, and outliers that can skew interpretations.
With a focus on enhancing the reliability of outcomes, effective time series data preprocessing data preprocessing paves the way for more robust analysis, leading to well-informed decisions and valuable discoveries across various fields and industries. In the section below, we’ll discuss about data preprocessing in data science.
So, let’s get started.
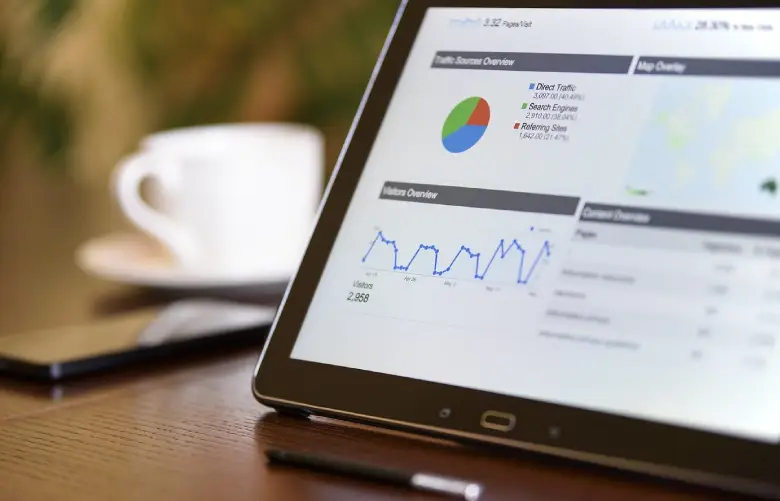
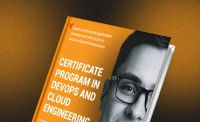
Get curriculum highlights, career paths, industry insights and accelerate your technology journey.
Download brochure
What Is Data Preprocessing
Data preprocessing is the essential groundwork in the journey from raw data to insightful conclusions. It involves a series of data preprocessing techniques and procedures to refine and structure the initial data for analysis. One key aspect is data transformation, where data is converted into a more suitable format, standardized, and made consistent. This can include tasks like scaling numerical values to a common range, encoding categorical variables, and handling missing values through imputation.
Data preprocessing also involves detecting and rectifying anomalies or outliers that might distort analysis outcomes. By cleansing noisy data and handling inconsistencies, this step ensures the accuracy and reliability of subsequent analyses. Moreover, time series data preprocessing prepares the data for specific analysis techniques, optimizing their effectiveness.
Click here to check out the Advanced Certification Program in Data Science & Analytics.
Data Preprocessing Importance
Data preprocessing is the cornerstone of effective data analysis, resembling the careful groundwork before constructing a sturdy building. It’s like polishing rough diamonds before they dazzle. This process ensures that the data you work with is clean, accurate, and ready for insightful analysis. By identifying and rectifying errors, handling missing values, and data transformation into suitable formats, preprocessing guarantees the integrity and reliability of your results.
Analysis outcomes could be distorted or misleading without proper preprocessing due to noisy, incomplete, or inconsistent data. As a chef washes, chops, and preps ingredients before cooking a delicious meal, data preprocessing readies your raw data for the analytical feast ahead. It saves time, minimizes errors, and ensures that the interpretations drawn from your analysis are solid, enabling you to make informed decisions and unearth valuable insights from the vast sea of data.
Data Quality Assessment
Before any analysis begins, it’s vital to assess the quality of the data. This involves identifying issues such as missing values, duplicates, and outliers. Data with these problems can lead to skewed interpretations. Through data quality assessment, analysts can clearly understand the data’s limitations and take appropriate measures to address these issues, ensuring that the final results are based on trustworthy information.
Data Cleaning
Data often comes with imperfections, such as missing values, incorrect entries, and inconsistencies. Data cleaning involves identifying and rectifying these issues to create a more accurate dataset. This process might involve imputing missing values, correcting errors, and removing redundant or irrelevant data. Data cleaning guarantees that the analysis is based on a solid foundation, preventing potentially misleading insights from noisy or flawed data.
Data Transformation
Data comes in various formats and units, making directly applying analysis techniques challenging. Data transformation involves converting data into a consistent format and scale. This could mean scaling numerical values to a common range, encoding categorical variables into numerical forms, or even applying mathematical functions to create new features which better represent the underlying patterns in the data. Data Transformation ensures that the data is ready for the specific analytical methods.
Data Reduction
Sometimes, datasets can be huge and complex, leading to longer processing times & increased computational requirements. Data reduction techniques aim to simplify the dataset while preserving its essential characteristics. This can involve methods like dimensionality reduction, which reduces the number of features while retaining the most essential information. Data reduction speeds up analysis and helps prevent overfitting, where models perform well on the training data but struggle with new, unseen data.
Learn more about What is Tableau: Uses and Application.
Feature Selection and Extraction
Feature Selection is akin to curating a masterpiece. The vast expanse of data attributes involves cherry-picking the most influential and relevant features while leaving behind the noise. Including only the prime qualities significantly impacting the analysis simplifies the data without losing its intrinsic value. This expedites computation and reduces the risk of overfitting, where models get too cozy with the training data and falter in the real world.
Feature Extraction is the data magician’s act, where intricate patterns and relationships are unveiled by creating new, compact features. This data transformation involves converting complex data into a more manageable format by combining or changing existing attributes. Dimensionality reduction data preprocessing techniques such as Principal Component Analysis (PCA), lead the way, compressing data while preserving its essence.
Feature Selection and Extraction are a dynamic duo that simplifies complex data. Feature Selection fine-tunes the cast, ensuring that only the stars take the stage, while Feature Extraction unveils the underlying magic, making the difficult appear clear. These techniques collectively elevate data analysis, yielding accurate and understandable insights—empowering decision-makers to transform raw data into actionable wisdom.
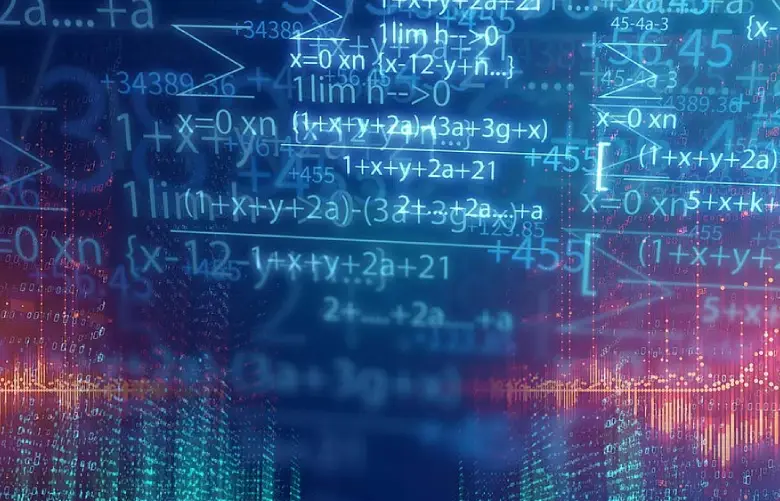
Normalization and Scaling for Consistent Data
Normalization is like adjusting the volume on speakers to ensure they play at the same level. In data terms, it’s transforming different attributes to a common scale, usually between 0 and 1. This level playing field helps prevent one attribute from overshadowing others due to its larger numerical range. Doing this ensures that every detail contributes fairly to your analysis, irrespective of its original magnitude.
Similarly, scaling ensures that all attributes have similar ranges in data, erasing the disparity in their numerical values. This balancing act prevents a feature with inherently larger values from exerting undue influence on your analysis, maintaining a sense of equality among all attributes. Together, normalization and scaling lay the groundwork for consistent data dynamics.
They ensure that the sheer differences in attribute scales don’t skew your analysis. Whether you’re working with attributes of various units, magnitudes, or ranges, these techniques harmonize your data, allowing for more accurate insights and conclusions. It’s like tuning a musical instrument to play in perfect harmony, turning a messy dataset into a symphony of structured information.
Conclusion
In the intricate realm of data analysis, the journey from raw data to meaningful insights is guided by the essential process of time series data preprocessing. By refining, cleaning, and data transformation, we pave the way for reliable analysis outcomes. As a painter prepares a canvas to bring out the brilliance of colors, data preprocessing sets the stage for impactful analysis. It weeds out inconsistencies, handles missing values, and ensures a consistent format, enhancing the quality of our insights.
Ultimately, this meticulous process elevates analysis quality, leading to more accurate decisions, valuable discoveries, and a deeper understanding of the intricate tapestry woven within our data.
FAQs
Data mining involves extracting valuable patterns, trends, or information from large datasets. It's like searching for gold in a mine. On the other hand, time series data preprocessing is the initial step before mining. It's like refining the raw gold ore – cleaning, purifying, and transforming it into a form suitable for analysis. In short, preprocessing sets the stage, while data mining digs out the treasures.
Data transformation is like molding clay to shape your creation. It involves changing the data format, scale, or structure to make it more suitable for analysis. For example, converting dates to day-of-the-week or scaling numerical values to a common range. These data transformations enhance data compatibility and prepare it for specific analysis methods.
Data preprocessing is the foundation of sound analysis. Imagine baking a cake – you don't just mix ingredients; you prep and measure them. Similarly, preprocessing cleans up errors, fills gaps, and standardizes data. This ensures analysis accuracy by preventing skewed results caused by noisy or incomplete data. It's like clearing a path in the forest before finding the treasure.
Normalization and scaling make data attributes play nicely together. Imagine comparing heights in feet and weights in pounds – it's like apples and oranges. Scaling brings them to the same unit, like kilograms and centimeters, ensuring fair comparison. Normalization then adjusts their ranges, like mapping them to values between 0 and 1, ensuring no attribute dominates others.
Data preprocessing acts as a decision-making compass. Raw data can be messy – like reading a map full of scribbles. Preprocessing cleans it up, so the path to insights is clear. Informed decisions rely on accurate information, and preprocessing ensures the data you're basing your choices on is trustworthy. It's the difference between navigating confidently and stumbling in the dark.